Identifying Insufficient Data Coverage for Ordinal Continuous-Valued Attributes
International Conference on Management of Data(2021)
摘要
ABSTRACTAppropriate training data is a requirement for building good machine-learned models. In this paper, we study the notion of coverage for ordinal and continuous-valued attributes, by formalizing the intuition that the learned model can accurately predict only at data points for which there are "enough" similar data points in the training data set. We develop an efficient algorithm to identify uncovered regions in low-dimensional attribute feature space, by making a connection to Voronoi diagrams. We also develop a randomized approximation algorithm for use in high-dimensional attribute space. We evaluate our algorithms through extensive experiments on real datasets.
更多查看译文
关键词
Responsible Data Science, Trustworthy AI, Fairness in Machine Learning, Bias Detection
AI 理解论文
溯源树
样例
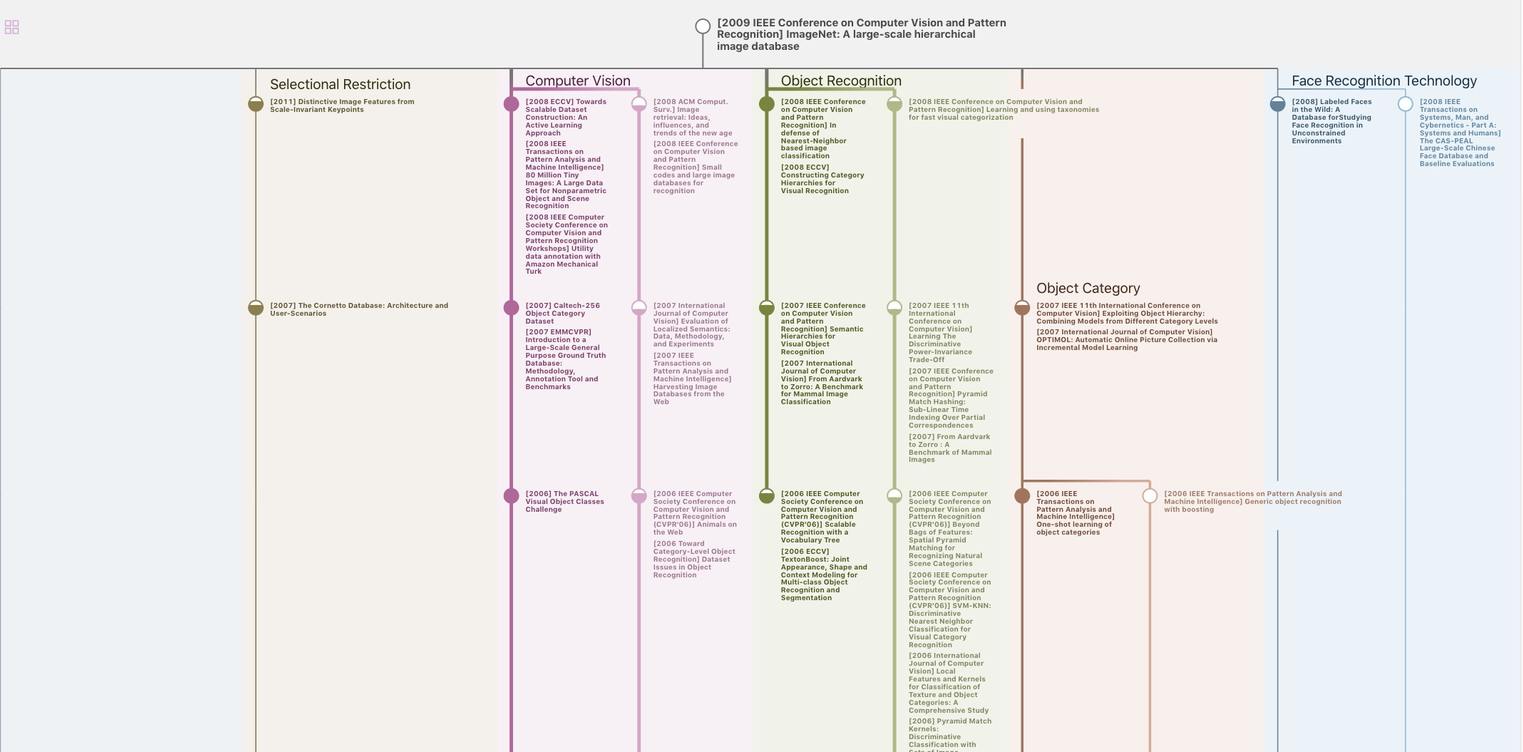
生成溯源树,研究论文发展脉络
Chat Paper
正在生成论文摘要