Predicting Prolonged Hospitalization and Supplemental Oxygenation in Patients with COVID-19 Infection from Ambulatory Chest Radiographs using Deep Learning
Academic Radiology(2021)
摘要
•Supervised multi-task deep learning with convolutional neural networks (CNNs) on frontal chest radiographs was able to predict many underlying patient comorbidities represented by hierarchical condition categories (HCCs) from the International Classification of Diseases, Tenth Revision, including those corresponding to diabetes with chronic complications, morbid obesity, congestive heart failure, cardiac arrhythmias, and chronic obstructive pulmonary disease. Using submitted HCC codes to train and test the CNNs, among all predicted comorbidities, the total area under the receiver operating characteristic (ROC) curve (AUC) was 0.856 (95% CI: 0.845-0.862), with individual AUCs ranging between 0.729 and 0.927.•Combining the multi-task CNN output with patient age and two standardized COVID-19 airspace disease predictors in 413 outpatients testing positive for COVID-19, a standard frontal chest radiograph predicted hospitalization of >2 days' duration and supplemental oxygenation with an ROC AUC of 0.837 (95% CI: 0.791-0.883), independent of additional clinical and laboratory data.
更多查看译文
关键词
COVID-19,deep learning,multi-task learning,convolutional neural networks,chest radiography
AI 理解论文
溯源树
样例
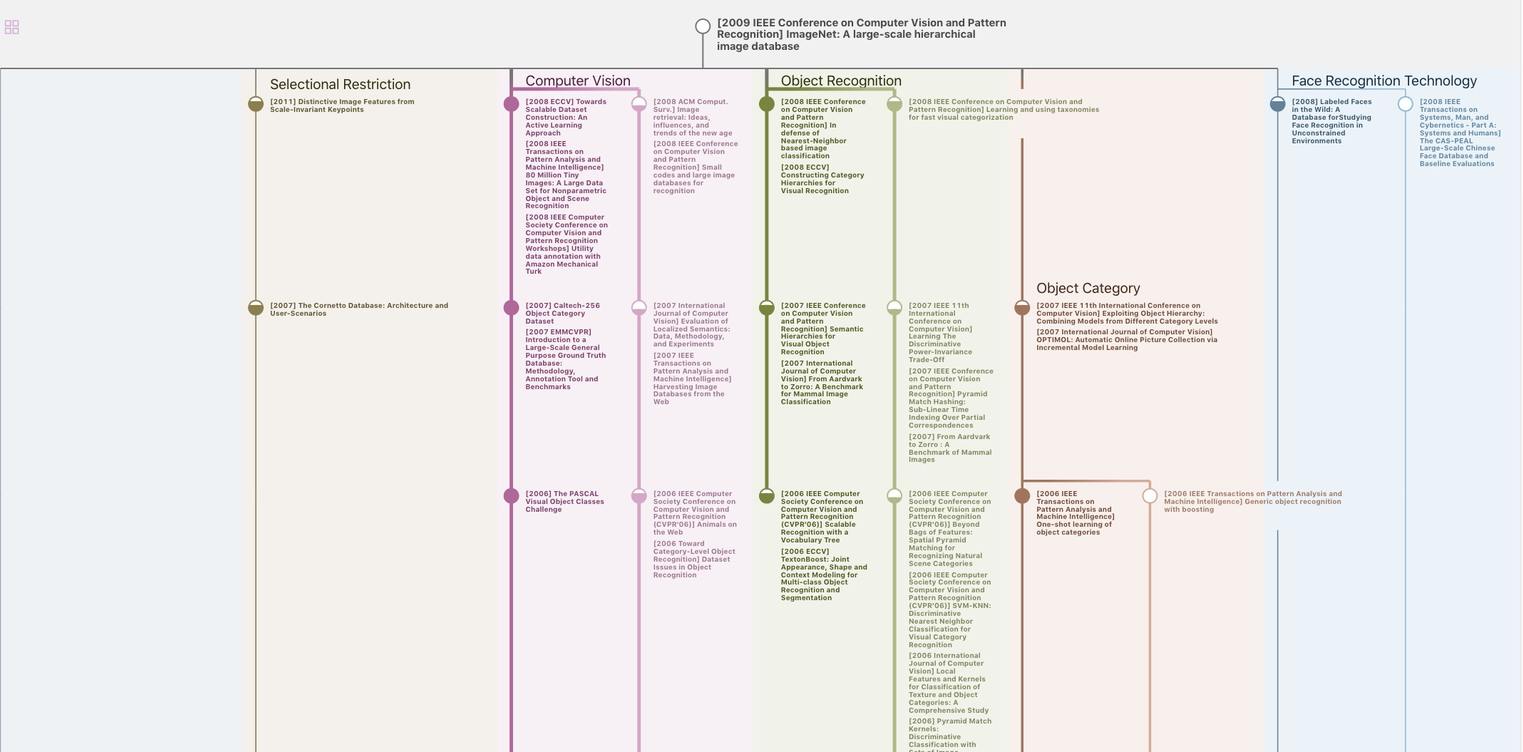
生成溯源树,研究论文发展脉络
Chat Paper
正在生成论文摘要