COMPLETER: Incomplete Multi-view Clustering via Contrastive Prediction
2021 IEEE/CVF CONFERENCE ON COMPUTER VISION AND PATTERN RECOGNITION, CVPR 2021(2021)
摘要
In this paper, we study two challenging problems in incomplete multi-view clustering analysis, namely, i) how to learn an informative and consistent representation among different views without the help of labels and ii) how to recover the missing views from data. To this end, we propose a novel objective that incorporates representation learning and data recovery into a unified framework from the view of information theory. To be specific, the informative and consistent representation is learned by maximizing the mutual information across different views through contrastive learning, and the missing views are recovered by minimizing the conditional entropy of different views through dual prediction. To the best of our knowledge, this could be the first work to provide a theoretical framework that unifies the consistent representation learning and cross-view data recovery. Extensive experimental results show the proposed method remarkably outperforms 10 competitive multi-view clustering methods on four challenging datasets. The code is available at https://pengxi.me.
更多查看译文
关键词
contrastive prediction,incomplete multiview clustering analysis,missing views,information theory,mutual information,contrastive learning,consistent representation learning,cross-view data recovery,competitive multiview,COMPLETER,conditional entropy
AI 理解论文
溯源树
样例
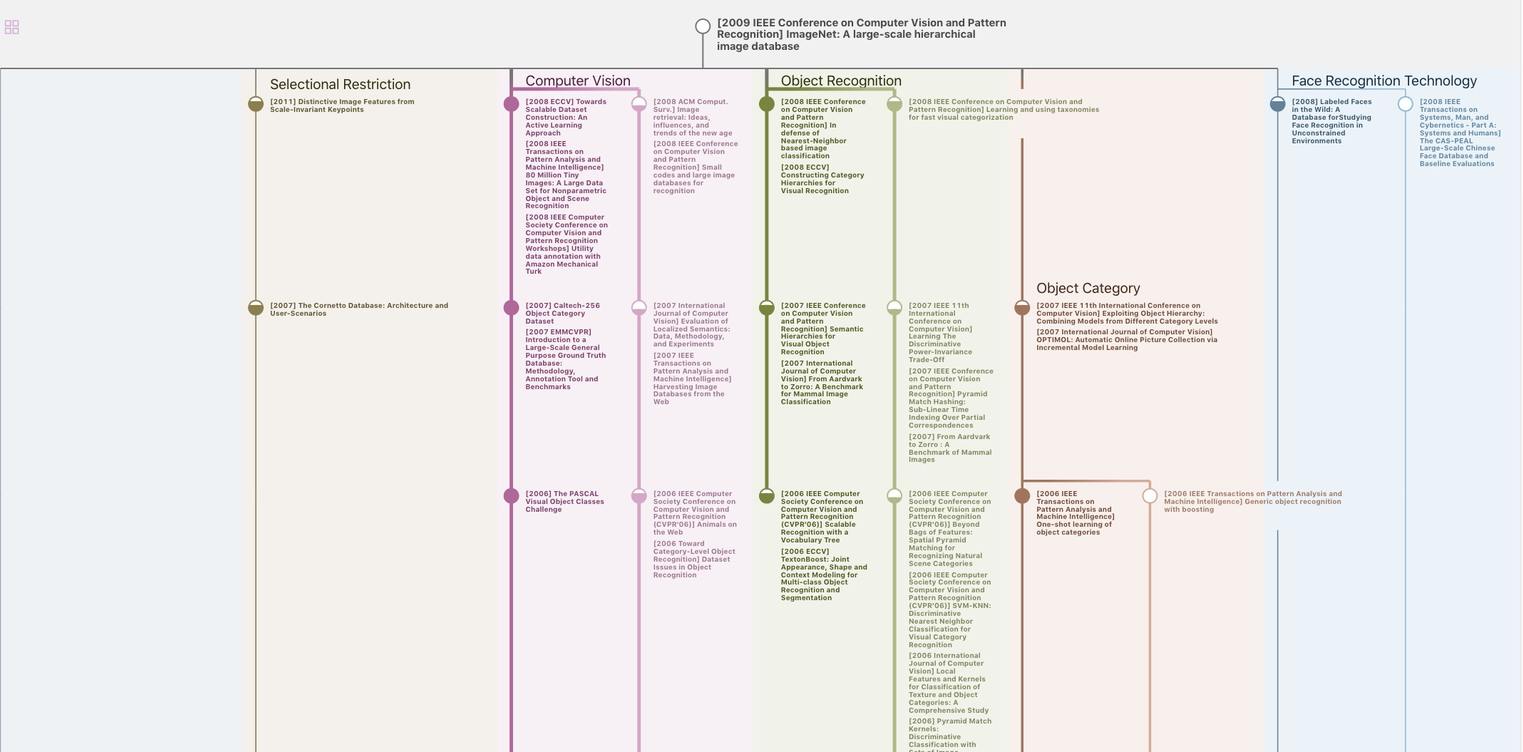
生成溯源树,研究论文发展脉络
Chat Paper
正在生成论文摘要