FBNetV3: Joint Architecture-Recipe Search using Predictor Pretraining
2021 IEEE/CVF CONFERENCE ON COMPUTER VISION AND PATTERN RECOGNITION, CVPR 2021(2021)
摘要
Neural Architecture Search (NAS) yields state-of-the-art neural networks that outperform their best manually-designed counterparts. However, previous NAS methods search for architectures under one set of training hyper-parameters (i.e., a training recipe), overlooking superior architecture-recipe combinations. To address this, we present Neural Architecture-Recipe Search (NARS) to search both (a) architectures and (b) their corresponding training recipes, simultaneously. NARS utilizes an accuracy predictor that scores architecture and training recipes jointly, guiding both sample selection and ranking. Furthermore, to compensate for the enlarged search space, we leverage "free" architecture statistics (e.g., FLOP count) to pretrain the predictor, significantly improving its sample efficiency and prediction reliability. After training the predictor via constrained iterative optimization, we run fast evolutionary searches in just CPU minutes to generate architecture-recipe pairs for a variety of resource constraints, called FBNetV3. FBNetV3 makes up a family of state-of-the-art compact neural networks that outperform both automatically and manually-designed competitors. For example, FB-NetV3 matches both EfficientNet and ResNeSt accuracy on ImageNet with up to 2.0. and 7.1. fewer FLOPs, respectively. Furthermore, FBNetV3 yields significant performance gains for downstream object detection tasks, improving mAP despite 18% fewer FLOPs and 34% fewer parameters than EfficientNet-based equivalents.
更多查看译文
关键词
NARS,search space,compact neural networks,predictor pretraining,neural networks,previous NAS methods search,training hyper-parameters,training recipe,neural architecture-recipe search,FBNetV3,free architecture statistics,evolutionary searches,constrained iterative optimization
AI 理解论文
溯源树
样例
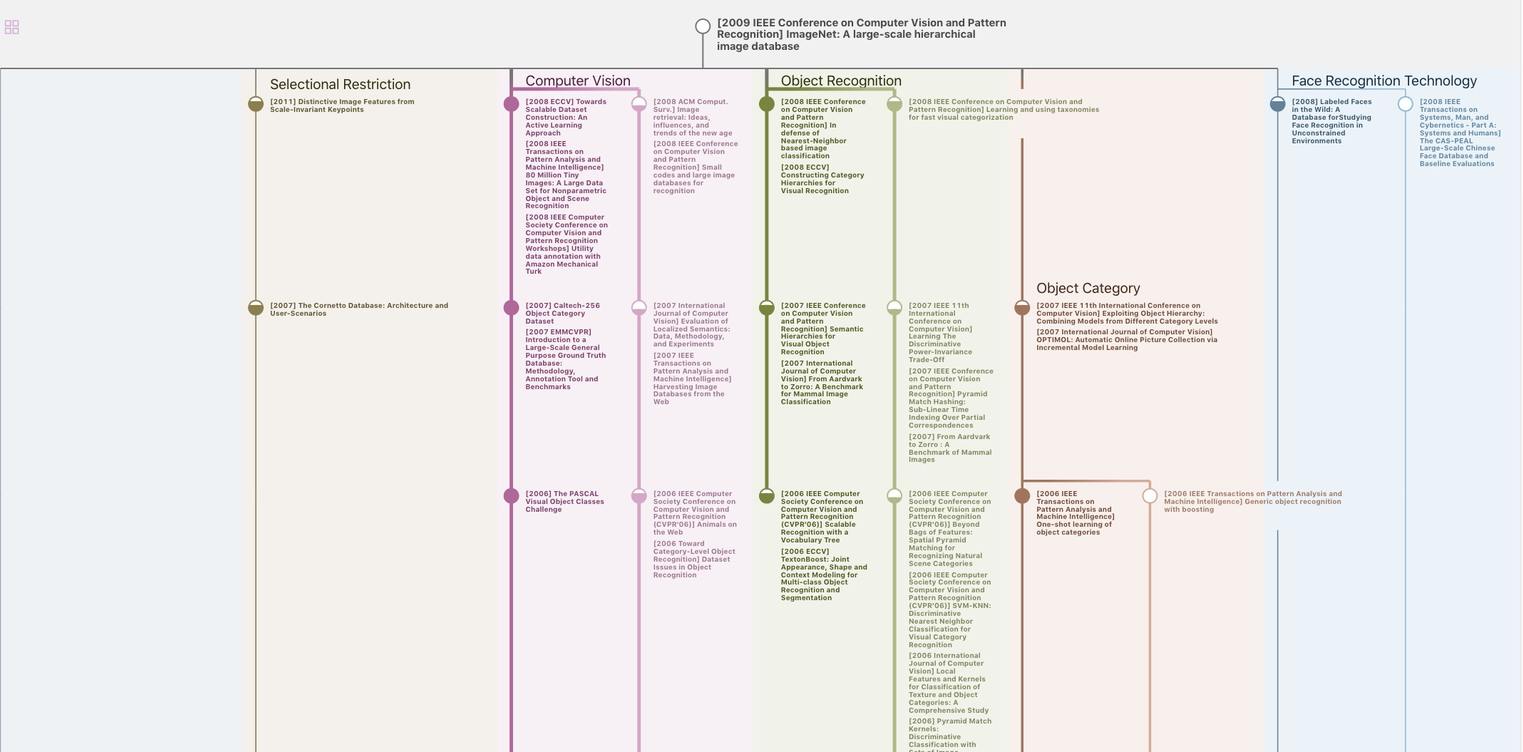
生成溯源树,研究论文发展脉络
Chat Paper
正在生成论文摘要