Uncertainty-Aware Camera Pose Estimation from Points and Lines
2021 IEEE/CVF CONFERENCE ON COMPUTER VISION AND PATTERN RECOGNITION, CVPR 2021(2021)
摘要
Perspective-n-Point-and-Line (PnPL) algorithms aim at fast, accurate, and robust camera localization with respect to a 3D model from 2D-3D feature correspondences, being a major part of modern robotic and AR/VR systems. Current point-based pose estimation methods use only 2D feature detection uncertainties, and the line-based methods do not take uncertainties into account. In our setup, both 3D coordinates and 2D projections of the features are considered uncertain. We propose PnP(L) solvers based on EPnP [20] and DLS [14] for the uncertainty-aware pose estimation. We also modify motion-only bundle adjustment to take 3D uncertainties into account. We perform exhaustive synthetic and real experiments on two different visual odometry datasets. The new PnP(L) methods outperform the state-of-the-art on real data in isolation, showing an increase in mean translation accuracy by 18% on a representative subset of KITTI, while the new uncertain refinement improves pose accuracy for most of the solvers, e.g. decreasing mean translation error for the EPnP by 16% compared to the standard refinement on the same dataset. The code is available at https://alexandervakhitov.github.io/uncertain-pnp/.
更多查看译文
关键词
camera,estimation,lines,uncertainty-aware
AI 理解论文
溯源树
样例
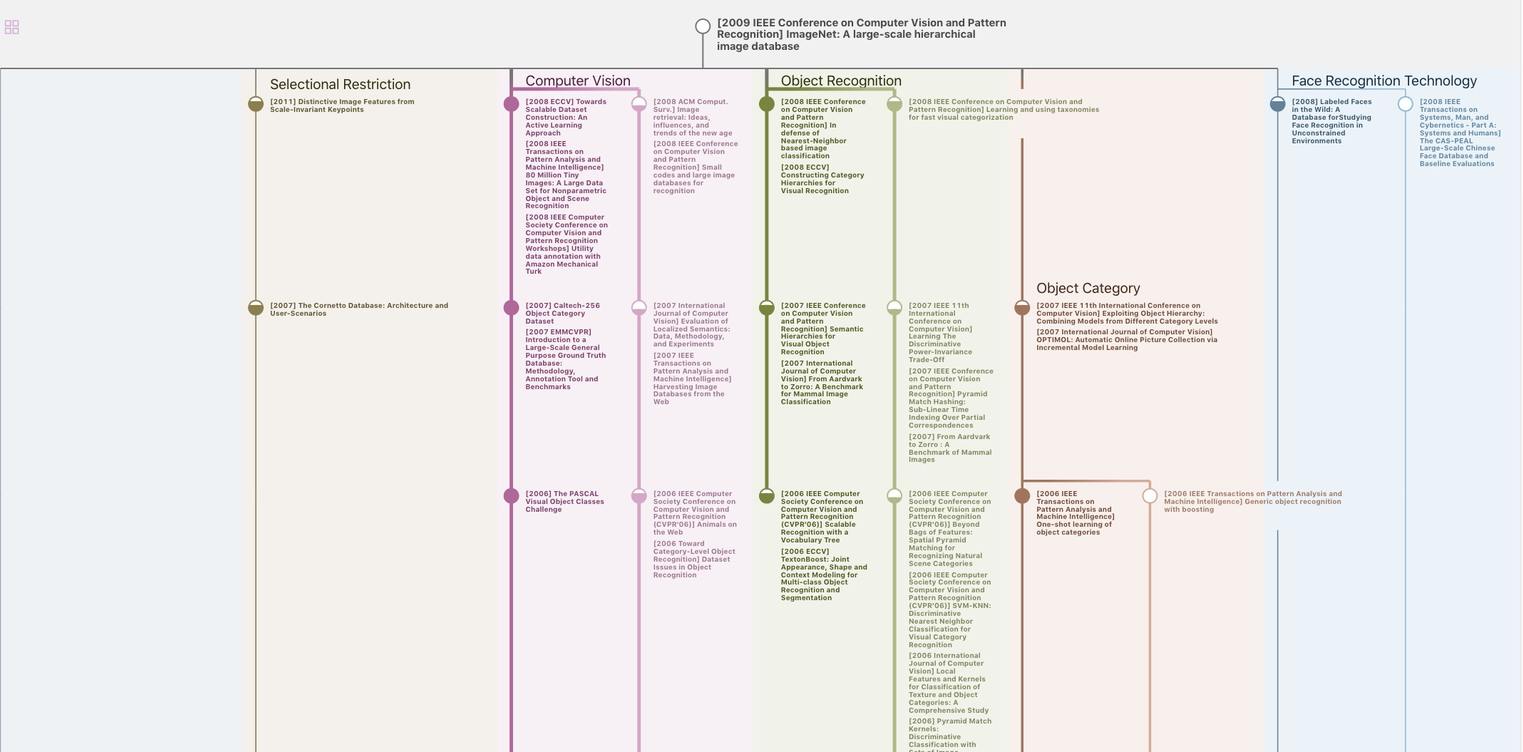
生成溯源树,研究论文发展脉络
Chat Paper
正在生成论文摘要