S-2-MLP: Spatial-Shift MLP Architecture for Vision
arxiv(2022)
摘要
Recently, visual Transformer (ViT) and its following works abandon the convolution and exploit the self-attention operation, attaining a comparable or even higher accuracy than CNN. More recently, MLP-mixer abandons both the convolution and the self-attention operation, proposing an architecture containing only MLP layers. To achieve crosspatch communications, it devises an additional token-mixing MLP besides the channel-mixing MLP. It achieves promising results when training on an extremely large-scale dataset such as JFT-300M. But it cannot achieve as outstanding performance as its CNN and ViT counterparts when training on medium-scale datasets such as ImageNet- 1K. The performance drop of MLP-mixer motivates us to rethink the token-mixing MLP. We discover that token-mixing operation in MLP-mixer is a variant of depthwise convolution with a global reception field and spatial-specific configuration. In this paper, we propose a novel pure MLP architecture, spatial-shift MLP (S-2 -MLP). Different from MLP-mixer, our S-2-MLP only contains channel-mixing MLP. We devise a spatial-shift operation for achieving the communication between patches. It has a local reception field and is spatial-agnostic. Meanwhile, it is parameter-free and efficient for computation. The proposed S-2 -MLP attains higher recognition accuracy than MLP-mixer when training on ImageNet-1K dataset. Meanwhile, S-2 -MLP accomplishes as excellent performance as ViT on ImageNet-1K dataset with considerably simpler architecture and fewer FLOPs and parameters.
更多查看译文
关键词
vision,architecture,spatial-shift
AI 理解论文
溯源树
样例
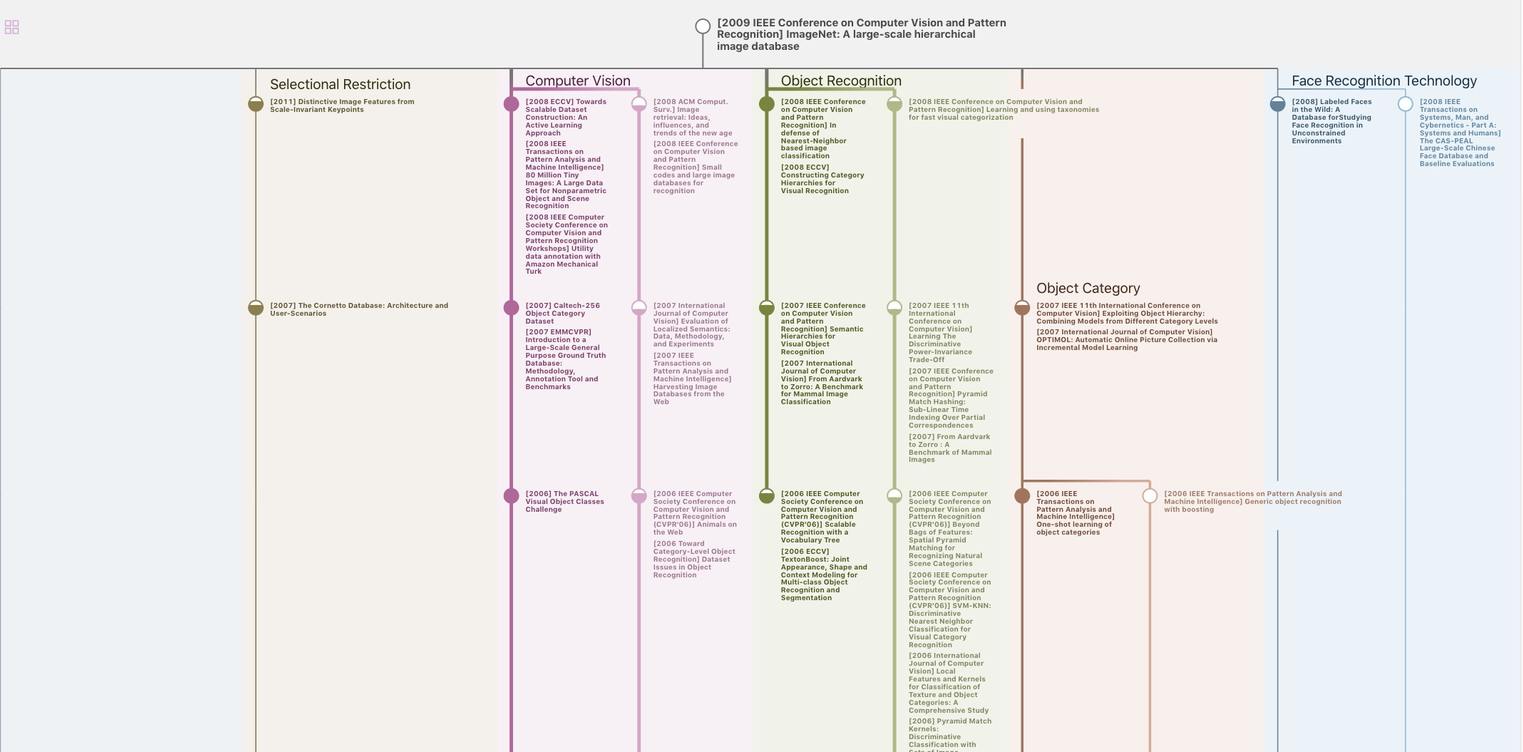
生成溯源树,研究论文发展脉络
Chat Paper
正在生成论文摘要