Generate, Annotate, and Learn: Generative Models Advance Self-Training and Knowledge Distillation
arxiv(2021)
摘要
Semi-Supervised Learning (SSL) has seen success in many application domains, but this success often hinges on the availability of task-specific unlabeled data. Knowledge distillation (KD) has enabled compressing deep networks and ensembles, achieving the best results when distilling knowledge on fresh task-specific unlabeled examples. However, task-specific unlabeled data can be challenging to find. We present a general framework called "generate, annotate, and learn (GAL)" that uses unconditional generative models to synthesize in-domain unlabeled data, helping advance SSL and KD on different tasks. To obtain strong task-specific generative models, we adopt generic generative models, pretrained on open-domain data, and fine-tune them on inputs from specific tasks. Then, we use existing classifiers to annotate generated unlabeled examples with soft pseudo labels, which are used for additional training. When self-training is combined with samples generated from GPT2-large, fine-tuned on the inputs of each GLUE task, we outperform a strong RoBERTa-large baseline on the GLUE benchmark. Moreover, KD on GPT-2 samples yields a new state-of-the-art for 6-layer transformers on the GLUE leaderboard. Finally, self-training with GAL offers significant gains on image classification on CIFAR-10 and four tabular tasks from the UCI repository
更多查看译文
关键词
knowledge distillation,annotate,self-training
AI 理解论文
溯源树
样例
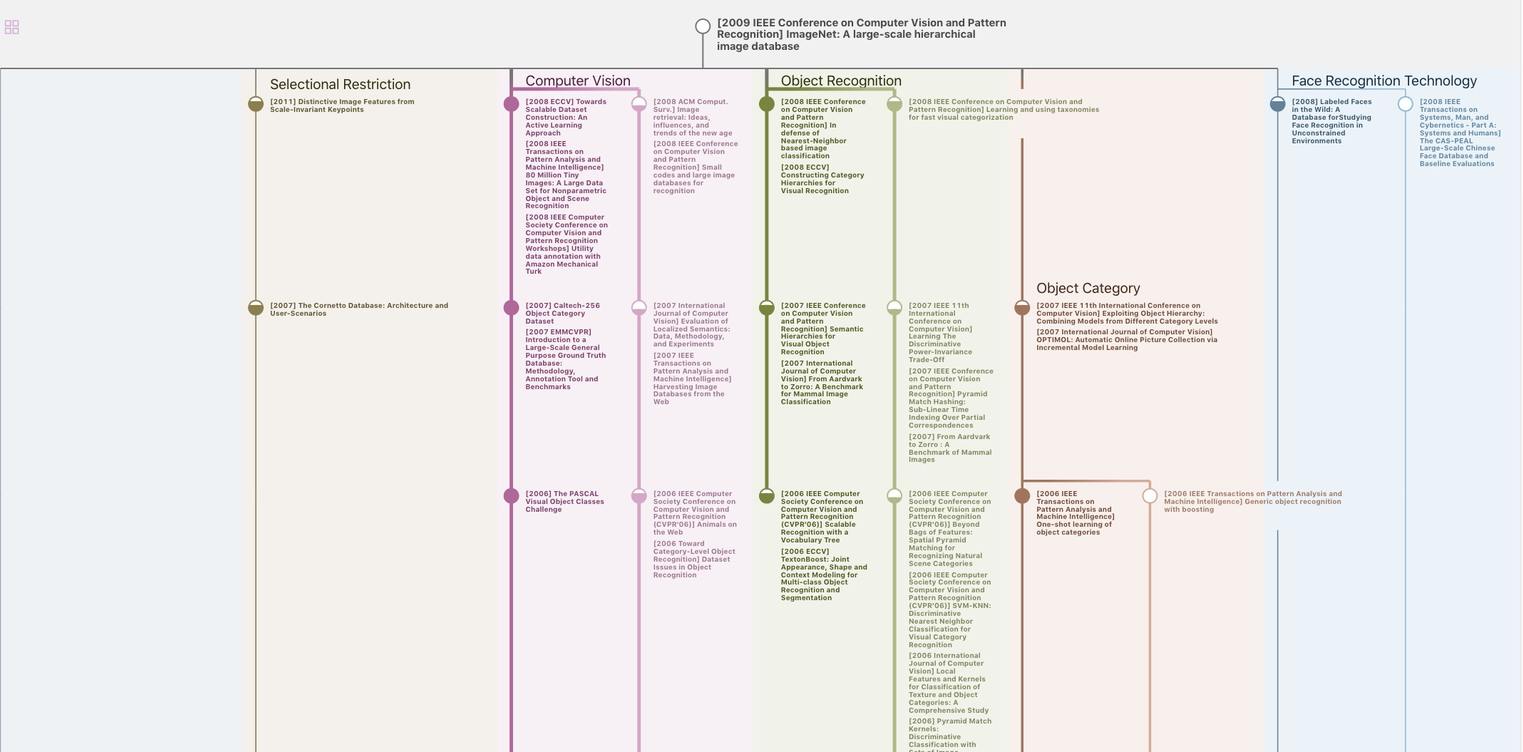
生成溯源树,研究论文发展脉络
Chat Paper
正在生成论文摘要