Hierarchical Topology-Based Cluster Representation for Scalable Evolutionary Multiobjective Clustering.
IEEE Transactions on Cybernetics(2022)
摘要
Evolutionary multiobjective clustering (MOC) algorithms have shown promising potential to outperform conventional single-objective clustering algorithms, especially when the number of clusters k is not set before clustering. However, the computational burden becomes a tricky problem due to the extensive search space and fitness computational time of the evolving population, especially when the data size is large. This article proposes a new, hierarchical, topology-based cluster representation for scalable MOC, which can simplify the search procedure and decrease computational overhead. A coarse-to-fine-trained topological structure that fits the spatial distribution of the data is utilized to identify a set of seed points/nodes, then a tree-based graph is built to represent clusters. During optimization, a bipartite graph partitioning strategy incorporated with the graph nodes helps in performing a cluster ensemble operation to generate offspring solutions more effectively. For the determination of the final result, which is underexplored in the existing methods, the usage of a cluster ensemble strategy is also presented, whether k is provided or not. Comparison experiments are conducted on a series of different data distributions, revealing the superiority of the proposed algorithm in terms of both clustering performance and computing efficiency.
更多查看译文
关键词
Clustering,ensemble strategy,multiobjective optimization,number of clusters,representation learning
AI 理解论文
溯源树
样例
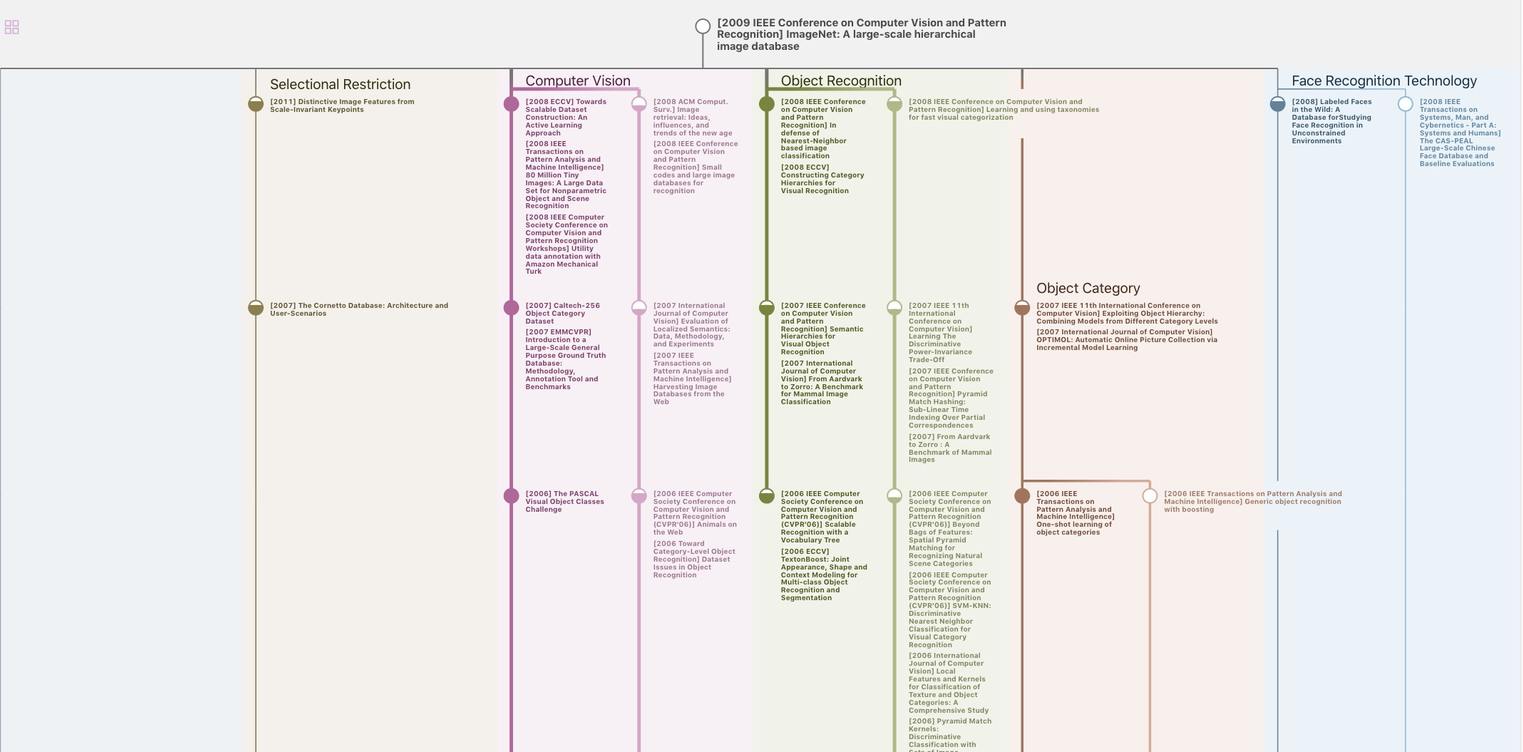
生成溯源树,研究论文发展脉络
Chat Paper
正在生成论文摘要