Are we really making much progress?: Revisiting, benchmarking and refining heterogeneous graph neural networks
Knowledge Discovery and Data Mining(2021)
摘要
ABSTRACTHeterogeneous graph neural networks (HGNNs) have been blossoming in recent years, but the unique data processing and evaluation setups used by each work obstruct a full understanding of their advancements. In this work, we present a systematical reproduction of 12 recent HGNNs by using their official codes, datasets, settings, and hyperparameters, revealing surprising findings about the progress of HGNNs. We find that the simple homogeneous GNNs, e.g., GCN and GAT, are largely underestimated due to improper settings. GAT with proper inputs can generally match or outperform all existing HGNNs across various scenarios. To facilitate robust and reproducible HGNN research, we construct the Heterogeneous Graph Benchmark (HGB) , consisting of 11 diverse datasets with three tasks. HGB standardizes the process of heterogeneous graph data splits, feature processing, and performance evaluation. Finally, we introduce a simple but very strong baseline Simple-HGN-which significantly outperforms all previous models on HGB-to accelerate the advancement of HGNNs in the future.
更多查看译文
关键词
Graph Neural Networks, Heterogeneous Graphs, Graph Representation Learning, Graph Benchmark, Heterogeneous Networks
AI 理解论文
溯源树
样例
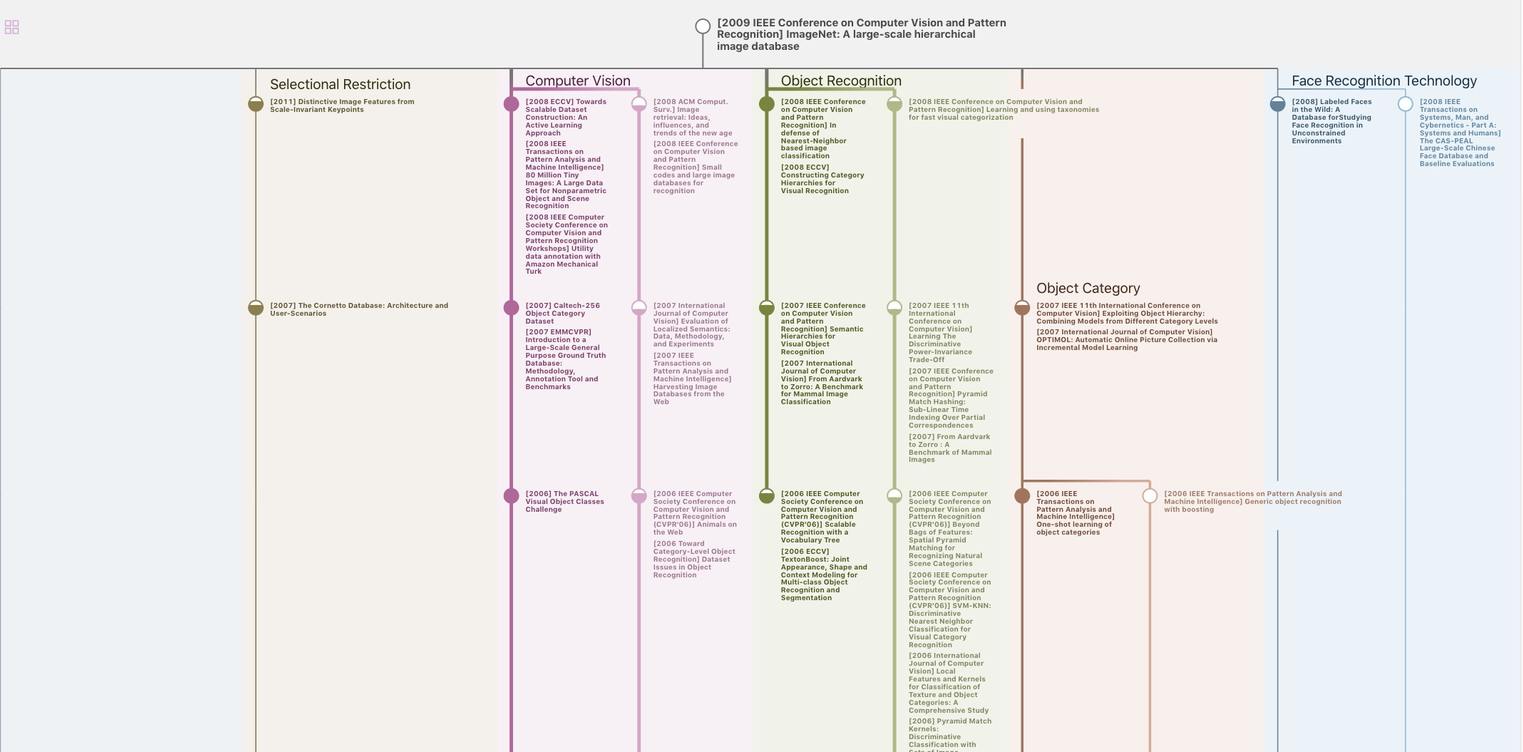
生成溯源树,研究论文发展脉络
Chat Paper
正在生成论文摘要