Bridging physiological and perceptual views of autism by means of sampling-based Bayesian inference
arxiv(2022)
摘要
Theories for autism spectrum disorder (ASD) have been formulated at different levels, ranging from physiological observations to perceptual and behavioral descriptions. Understanding the physiological underpinnings of perceptual traits in ASD remains a significant challenge in the field. Here we show how a recurrent neural circuit model that was optimized to perform sampling-based inference and displays characteristic features of cortical dynamics can help bridge this gap. The model was able to establish a mechanistic link between two descriptive levels for ASD: a physiological level, in terms of inhibitory dysfunction, neural variability, and oscillations, and a perceptual level, in terms of hypopriors in Bayesian computations. We took two parallel paths-inducing hypopriors in the probabilistic model, and an inhibitory dysfunction in the network model-which lead to consistent results in terms of the represented posteriors, providing support for the view that both descriptions might constitute two sides of the same coin. Author Summary Two different views of autism, one regarding altered probabilistic computations, and one regarding inhibitory dysfunction, are brought together by means of a recurrent neural network model trained to perform sampling-based inference in a visual setting. Moreover, the model captures a variety of experimental observations regarding differences in neural variability and oscillations in subjects with autism. By linking neural connectivity, dynamics, and function, this work contributes to the understanding of the physiological underpinnings of perceptual traits in autism spectrum disorder.
更多查看译文
关键词
Autism, Neural circuits, Inhibitory dysfunction, Hypopriors, Sampling-based inference
AI 理解论文
溯源树
样例
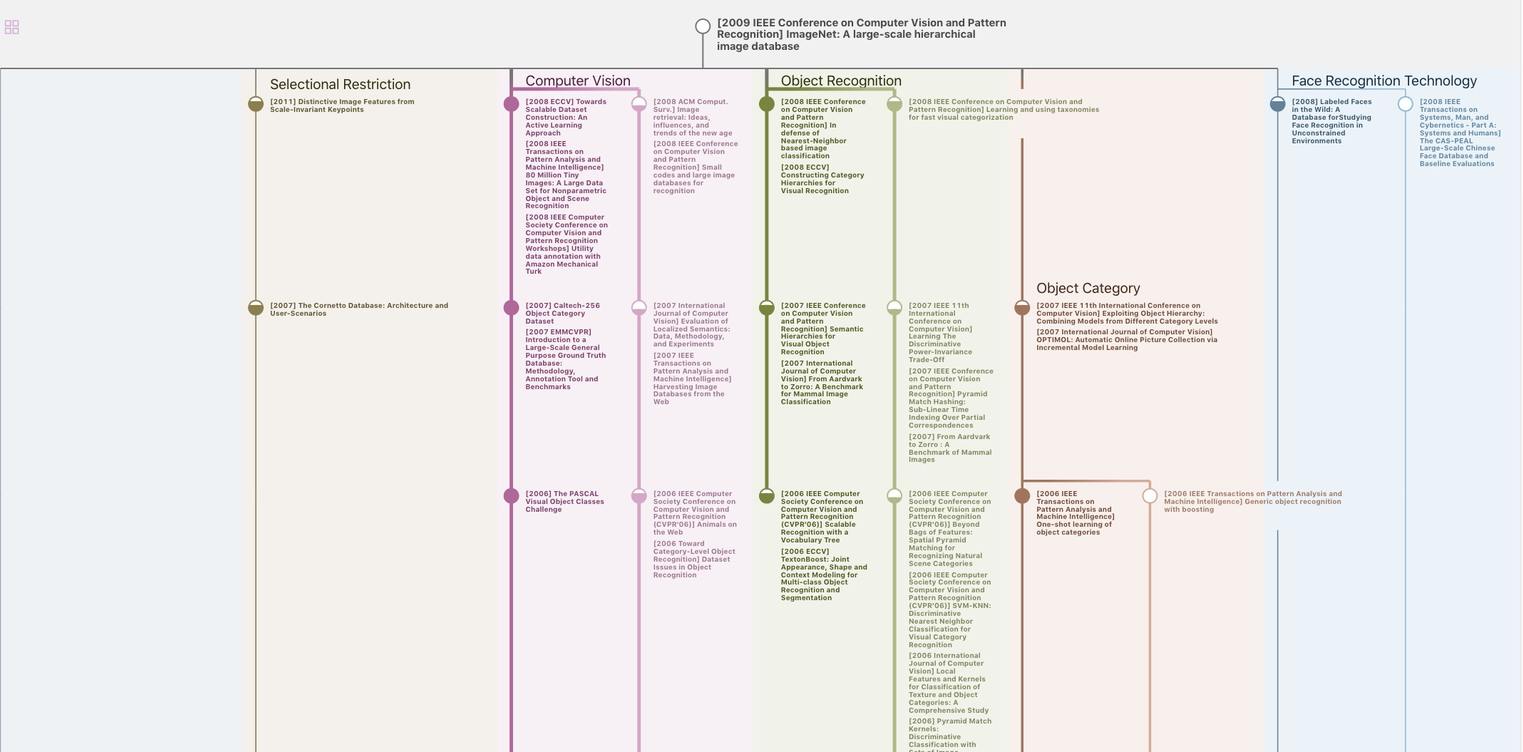
生成溯源树,研究论文发展脉络
Chat Paper
正在生成论文摘要