A Novel Key Point Trajectory Model for Fall Detection from RGB-D Videos
PROCEEDINGS OF THE 2021 IEEE 24TH INTERNATIONAL CONFERENCE ON COMPUTER SUPPORTED COOPERATIVE WORK IN DESIGN (CSCWD)(2021)
摘要
This paper aims to address the problem of fall detection from RGB-D image sequences. Towards this goal, we propose a novel Key Point Trajectory Model which represents a fall action as a series of trajectory descriptors. In the proposed model, 16 key points including 14 skeleton points and 2 centers of body parts are extracted from each pair of RGB and depth images. Then a global trajectory descriptor is constructed on 16 trajectories that are obtained by connecting the key points across several frames in the RGB-D sequence. The trajectory descriptor incorporates the spatial, depth, and temporal context of key points and characterizes the global motion of human over a short period of time. A random forest is employed to learn the classifier of trajectory descriptors, and an integration rule is developed for detecting falls according to the classification results of all trajectory descriptors within a video. Experiments conducted on two fall detection datasets demonstrate that our method achieves better performance in comparison with state-of-the-art methods.
更多查看译文
关键词
fall detection, key point, trajectory descriptor, random forest, RGB-D image sequence
AI 理解论文
溯源树
样例
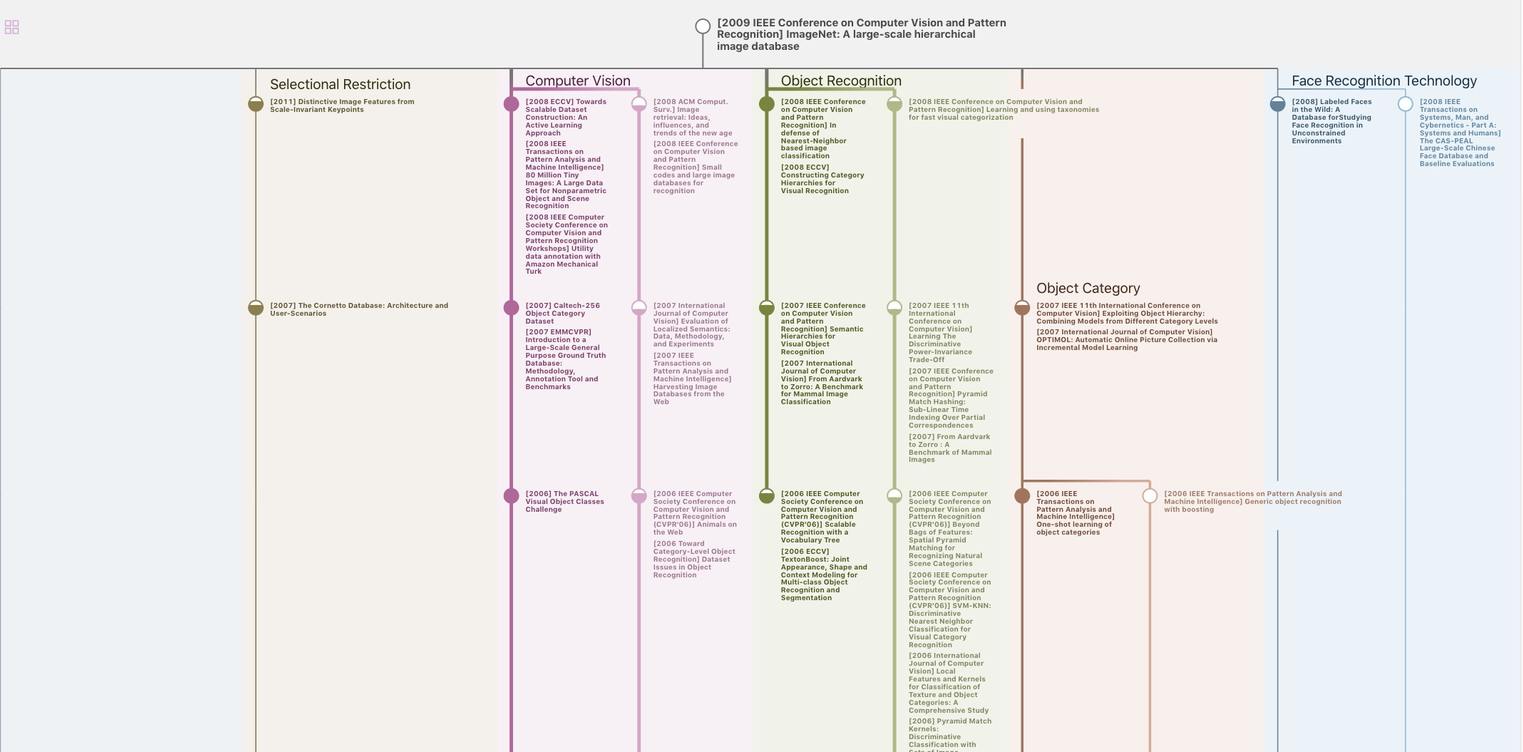
生成溯源树,研究论文发展脉络
Chat Paper
正在生成论文摘要