Vector Quantized Models for Planning
INTERNATIONAL CONFERENCE ON MACHINE LEARNING, VOL 139(2021)
摘要
Recent developments in the field of model-based RL have proven successful in a range of environments, especially ones where planning is essential. However, such successes have been limited to deterministic fully-observed environments. We present a new approach that handles stochastic and partially-observable environments. Our key insight is to use discrete autoencoders to capture the multiple possible effects of an action in a stochastic environment. We use a stochastic variant of Monte Carlo tree search to plan over both the agent's actions and the discrete latent variables representing the environment's response. Our approach significantly outperforms an offline version of MuZero on a stochastic interpretation of chess where the opponent is considered part of the environment. We also show that our approach scales to DeepMind Lab, a first-person 3D environment with large visual observations and partial observability.
更多查看译文
关键词
vector quantized models,planning
AI 理解论文
溯源树
样例
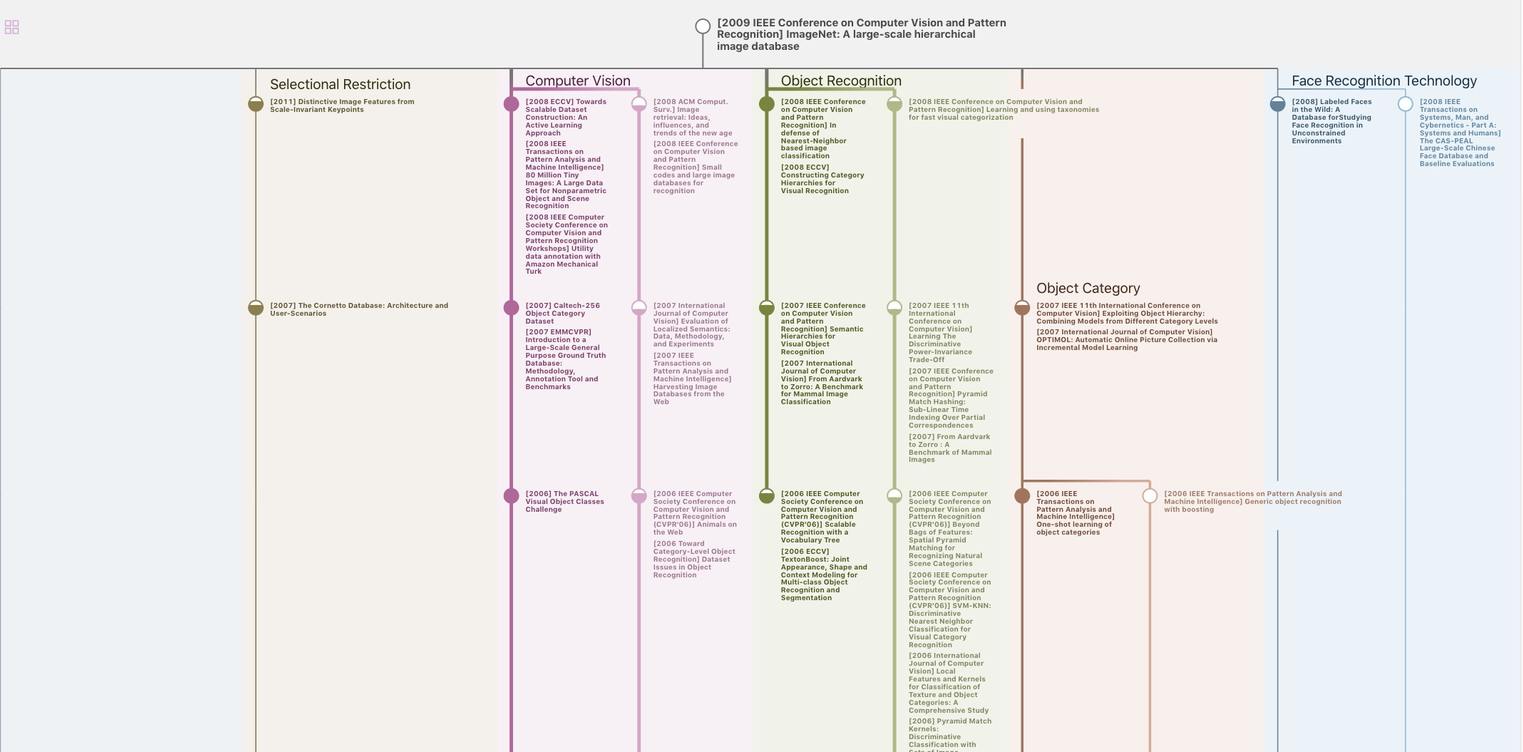
生成溯源树,研究论文发展脉络
Chat Paper
正在生成论文摘要