A hybrid feature selection-based approach for brain tumor detection and automatic segmentation on multiparametric magnetic resonance images
MEDICAL PHYSICS(2021)
摘要
Purpose To develop a novel method based on feature selection, combining convolutional neural network (CNN) and ensemble learning (EL), to achieve high accuracy and efficiency of glioma detection and segmentation using multiparametric MRIs. Methods We proposed an evolutionary feature selection-based hybrid approach for glioma detection and segmentation on 4 MR sequences (T2-FLAIR, T1, T1Gd, and T2). First, we trained a lightweight CNN to detect glioma and mask the suspected region to process large batch of MRI images. Second, we employed a differential evolution algorithm to search a feature space, which composed of 416-dimension radiomic features extracted from four sequences of MRIs and 128-dimension high-order features extracted by the CNN, to generate an optimal feature combination for pixel classification. Finally, we trained an EL classifier using the optimal feature combination to segment whole tumor (WT) and its subregions including nonenhancing tumor (NET), peritumoral edema (ED), and enhancing tumor (ET) in the suspected region. Experiments were carried out on 300 glioma patients from the BraTS2019 dataset using fivefold cross validation, the model was independently validated using the rest 35 patients from the same database. Results The approach achieved a detection accuracy of 98.8% using four MRIs. The Dice coefficients (and standard deviations) were 0.852 +/- 0.057, 0.844 +/- 0.046, and 0.799 +/- 0.053 for segmentation of WT (NET+ET+ED), tumor core (NET+ET), and ET, respectively. The sensitivities and specificities were 0.873 +/- 0.074, 0.863 +/- 0.072, and 0.852 +/- 0.082; the specificities were 0.994 +/- 0.005, 0.994 +/- 0.005, and 0.995 +/- 0.004 for the WT, tumor core, and ET, respectively. The performances and calculation times were compared with the state-of-the-art approaches, our approach yielded a better overall performance with average processing time of 139.5 s per set of four sequence MRIs. Conclusions We demonstrated a robust and computational cost-effective hybrid segmentation approach for glioma and its subregions on multi-sequence MR images. The proposed approach can be used for automated target delineation for glioma patients.
更多查看译文
关键词
auto-segmentation, ensemble classifier, evolutionary feature selection, glioma, tumor detection
AI 理解论文
溯源树
样例
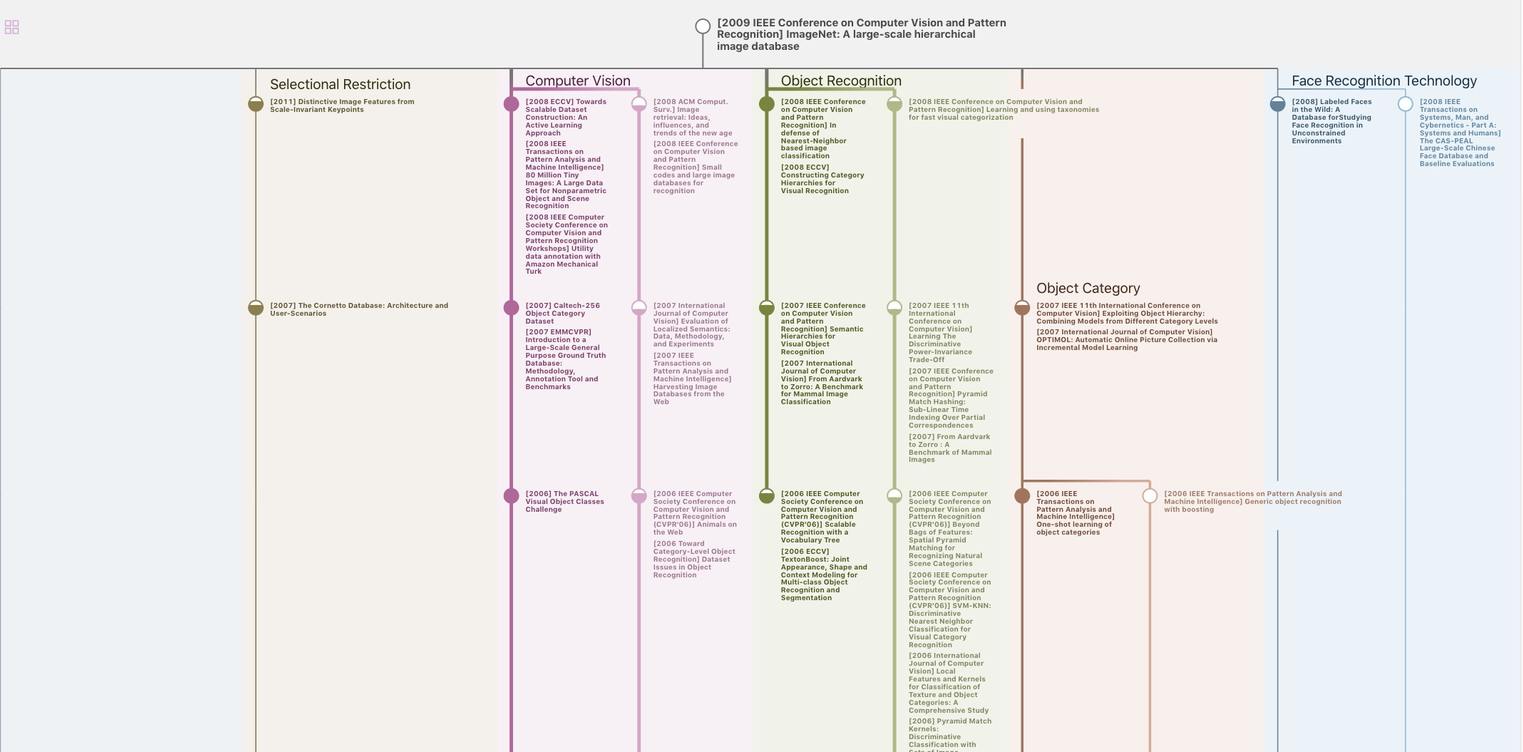
生成溯源树,研究论文发展脉络
Chat Paper
正在生成论文摘要