A Generalizable Approach to Learning Optimizers
arxiv(2021)
摘要
A core issue with learning to optimize neural networks has been the lack of generalization to real world problems. To address this, we describe a system designed from a generalization-first perspective, learning to update optimizer hyperparameters instead of model parameters directly using novel features, actions, and a reward function. This system outperforms Adam at all neural network tasks including on modalities not seen during training. We achieve 2x speedups on ImageNet, and a 2.5x speedup on a language modeling task using over 5 orders of magnitude more compute than the training tasks.
更多查看译文
关键词
generalizable approach,learning
AI 理解论文
溯源树
样例
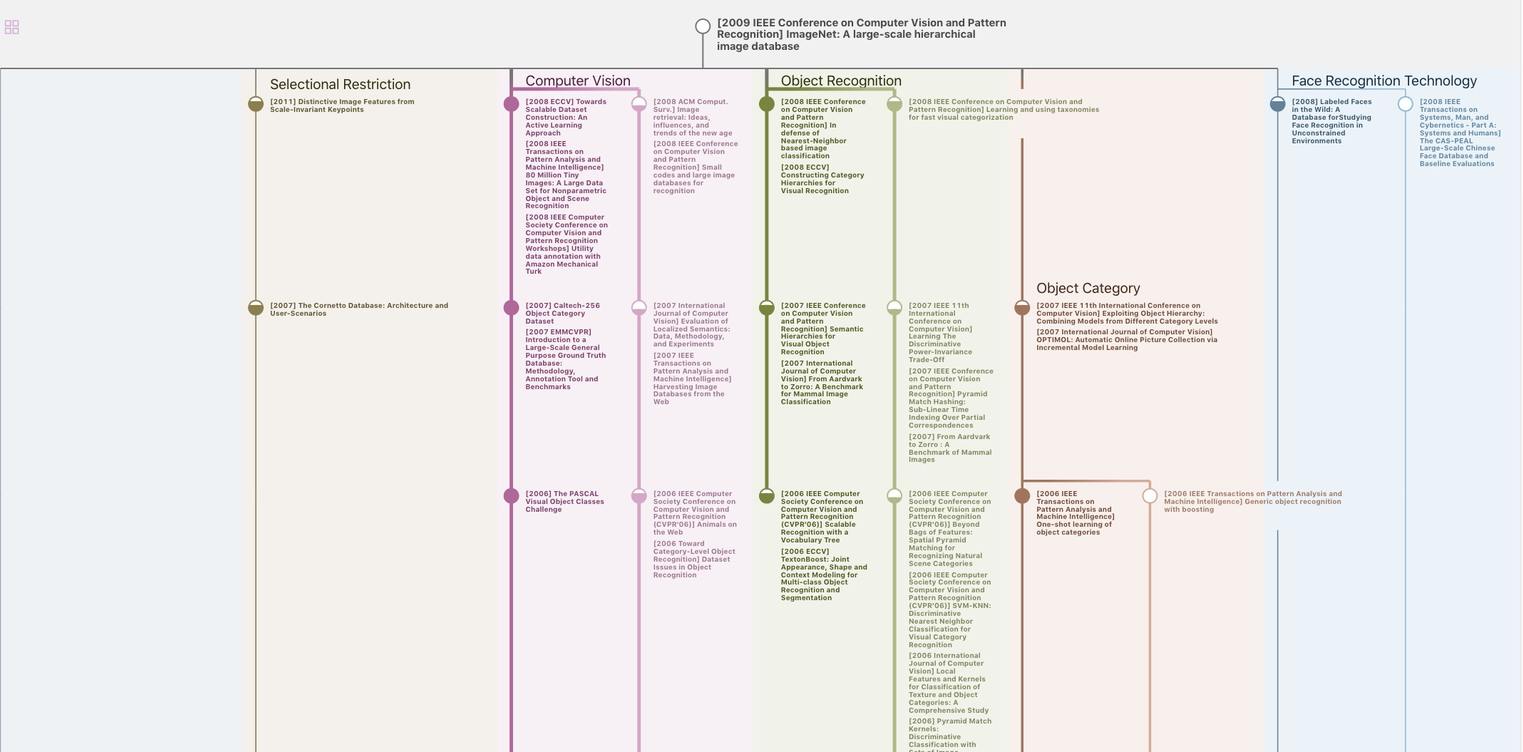
生成溯源树,研究论文发展脉络
Chat Paper
正在生成论文摘要