A Reinforcement Learning-Empowered Feedback Control System for Industrial Internet of Things
IEEE Transactions on Industrial Informatics(2022)
摘要
The rapid development of the Industrial Internet of Things (IIoT) enables IIoT devices to offload their computation-intensive tasks to nearby edges via wireless base stations and thus relieve their resource constraints. To better guarantee quality-of-service, it has become necessary to cooperate multiple edges instead of letting them work alone. However, the existing solutions commonly use a centralized decision-making manner and cannot effectively achieve good load balancing among massive edges that are widely distributed in IIoT environments. This results in long decision-making time and high communication costs. To address this important problem, in this article, we propose a reinforcement learning (RL)-empowered feedback control method for cooperative load balancing (RF-CLB). First, by integrating RL and machine learning (ML) algorithms, each edge independently schedules tasks and performs load balancing between adjacent edges based on the local information. Next, through feedback control and multiedge cooperation, the objective multiedge load-balancing plan for IIoT can be found. Simulation results demonstrate that the RF-CLB chooses the adjustment operations of load balancing with 96.3% correctness. Moreover, the RF-CLB achieves the near-optimal performance, which outperforms the classic ML-based and rule-based methods by 6–9% and 10–12%, respectively.
更多查看译文
关键词
Feedback control,Industrial Internet of Things,multiedge load balancing,reinforcement learning
AI 理解论文
溯源树
样例
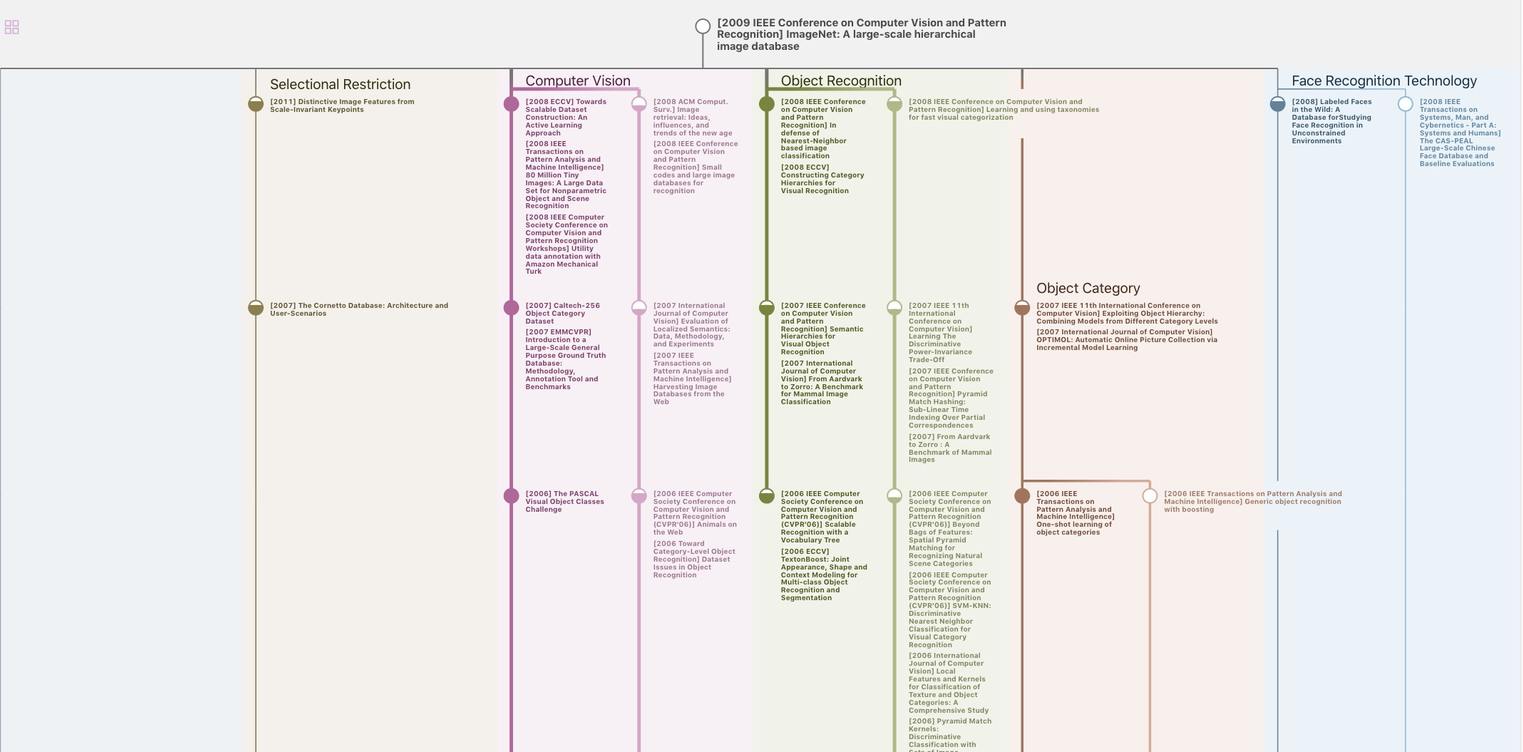
生成溯源树,研究论文发展脉络
Chat Paper
正在生成论文摘要