EFCNet: Ensemble Full Convolutional Network for Semantic Segmentation of High-Resolution Remote Sensing Images
IEEE GEOSCIENCE AND REMOTE SENSING LETTERS(2022)
摘要
Convolutional neural networks (CNNs) have achieved remarkable results in semantic segmentation of high-resolution remote sensing images (HRRSIs). However, the scales and textures of HRRSIs are diverse, which makes it difficult for a fixed-layer CNN to obtain rich features. In this regard, we propose an end-to-end ensemble fully convolutional network (EFCNet), which mainly includes two modules: the adaptive fusion module (AFM) and the separable convolutional module (SCM). The AFM can fuse features of different scales based on ensemble learning, whereas the SCM can reduce the complexity of the model under multifeature fusion. In the experiment, we use UNet and PSPNet to verify the framework on the ISPRS Vaihingen and Potsdam datasets. The experimental results show that the EFCNet can effectively improve the final segmentation performance and reduce the complexity of the ensemble model.
更多查看译文
关键词
Convolution, Feature extraction, Kernel, Training, Remote sensing, Image segmentation, Spatial resolution, Convolutional neural network (CNN), ensemble learning, remote sensing, semantic segmentation
AI 理解论文
溯源树
样例
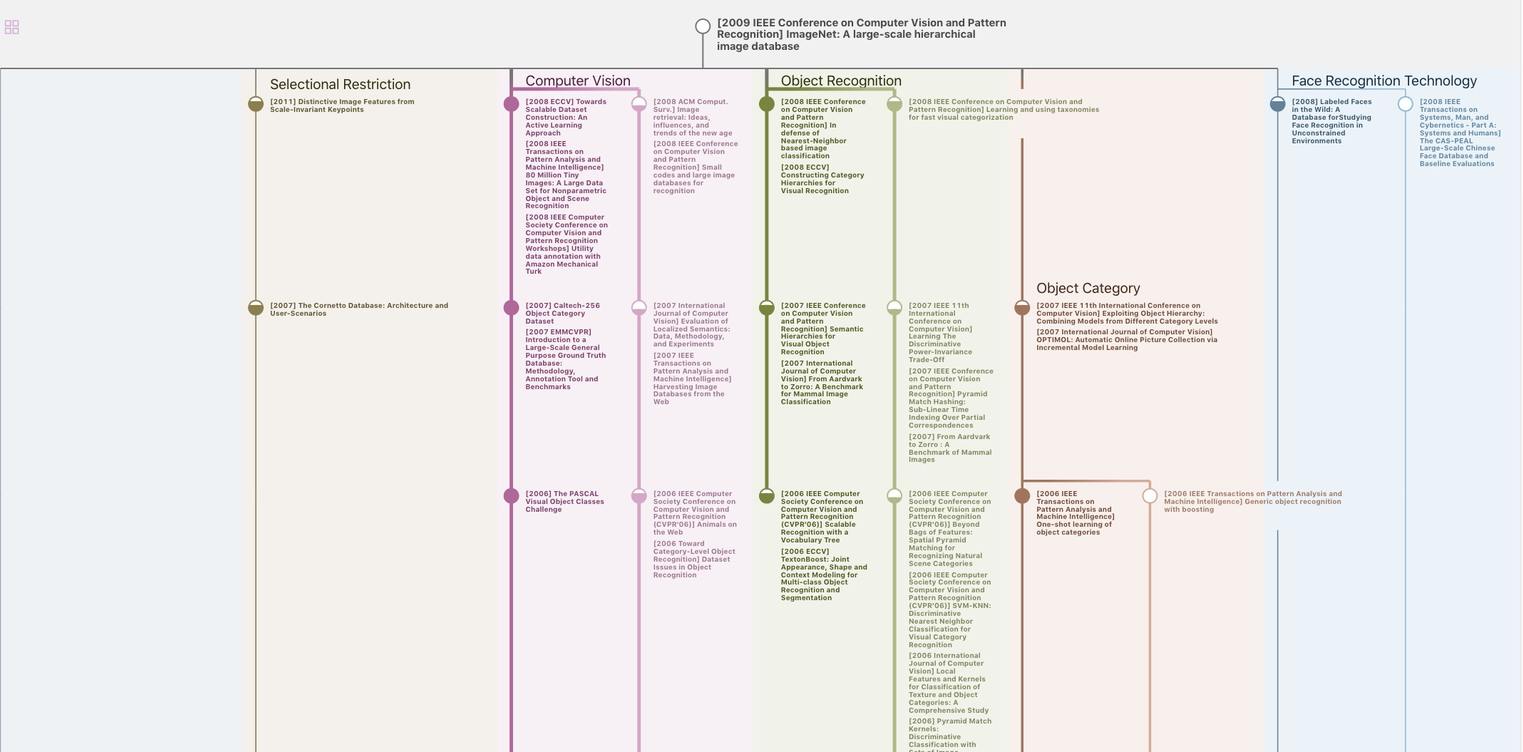
生成溯源树,研究论文发展脉络
Chat Paper
正在生成论文摘要