A 216 Mu W, 87% Accurate Cow Behavior Classifying Decision Tree On Fpga With Interpolated Arctan2
2021 IEEE INTERNATIONAL SYMPOSIUM ON CIRCUITS AND SYSTEMS (ISCAS)(2021)
摘要
This work presents five different 8-bit fixed point low power hardware implementations of cow behavior classifying axis-parallel decision trees (DT) for a range of five feature sets (FS). Each DT is trained and tested with 3-axis accelerometer data labeled beforehand containing four main behaviors, resting, eating, rumination and moving. Investigation shows a 10% F1-score decrement from software (77.4%) to 8-bit fixed point hardware (67.4%) for the conventional feature set, i.e. average mean and variation of the 3D accelerometer vector magnitude (FS4) due to quantization. The horizontal-longitudinal angle of acceleration of the cow (arctan2(y, x)) is proposed as a new FS together with the vertical z-axis (FS1&FS2). The results of an experimental setup, which simulates a stratified 20% split of the entire dataset from PC to FPGA, shows an average accuracy of 86.81% respectively for FS2. This FS's hardware implementation programmed on a Lattice ICE40UP5K FPGA has a power consumption of 216 mu W with a latency of 2.58 s at the frequency of 2.9 kHz resulting in an energy of 557 mu J per classification, which is competitive with state of the art.
更多查看译文
关键词
low power hardware, decision tree, cow behavior, FPGA, interpolated arctangent
AI 理解论文
溯源树
样例
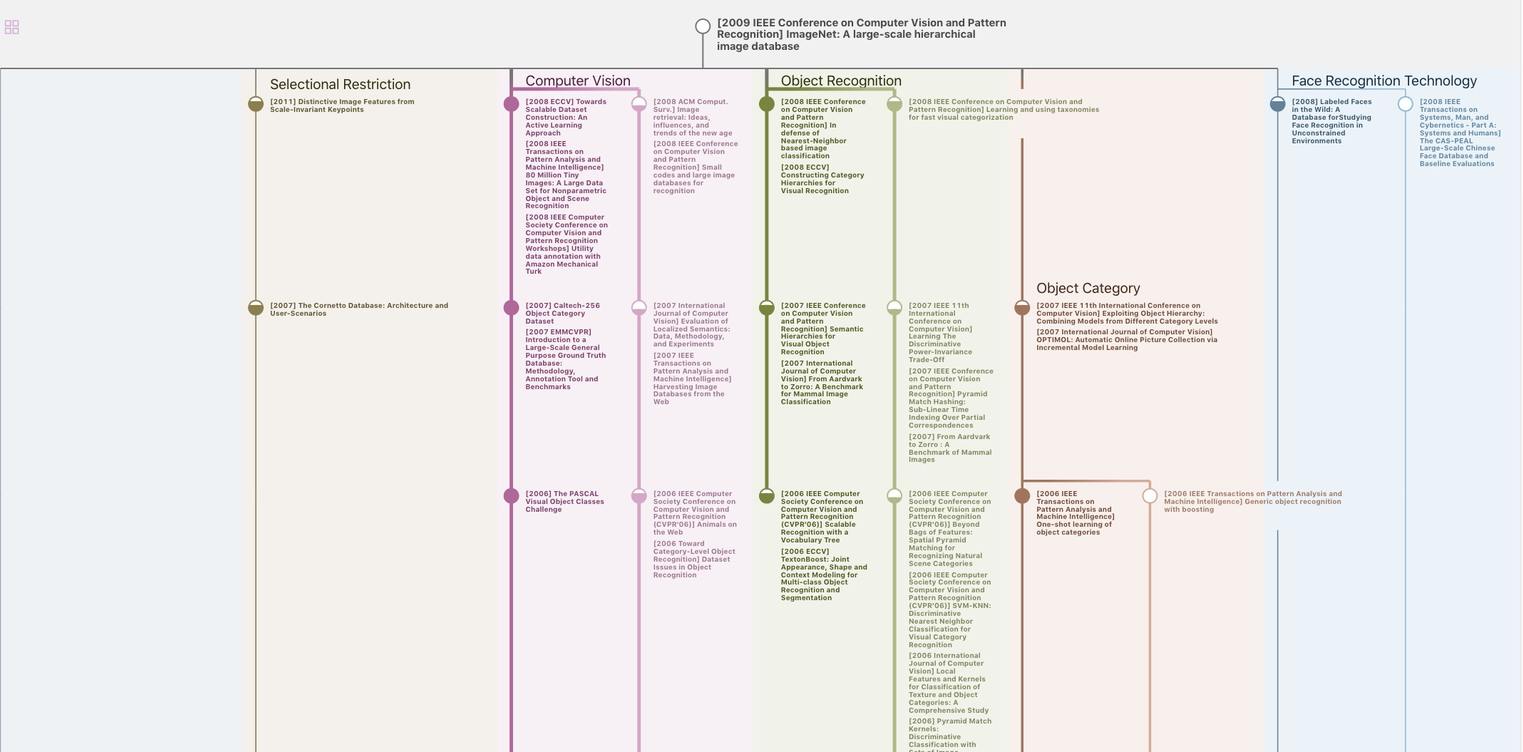
生成溯源树,研究论文发展脉络
Chat Paper
正在生成论文摘要