Performance of support vector regression in correcting UWB ranging measurements under LOS/NLOS conditions
CPSWEEK(2021)
摘要
ABSTRACTUltra-wideband technology has recently gained interest due to its inherently fine temporal resolution, which enables precise measurements of the time-of-flight between devices. The accuracy of these measurements depends, among others, on the presence of a free line-of-sight (LOS): in case the latter is partly or fully blocked, the direct path component cannot be accurately identified, leading to large errors in the estimated distance. To cope with this problem, many approaches based on machine learning have been proposed to detect non-line-of-sight (NLOS) conditions and mitigate erroneous ranging measurements. However, the performance of these approaches as a function of various features and different LOS/NLOS conditions has rarely been evaluated on off-the-shelf devices in an exhaustive way. In this work we systematically benchmark the accuracy of error correction models based on support vector regression. To this end, we perform a large experimental campaign on off-the-shelf ultra-wideband devices, in which we collect 35050 ranging measurements in various environments and different LOS conditions. Our analysis of the collected data shows that a detection and mitigation strategy, where measurements are first classified and only corrected if a NLOS condition is detected, can help reducing the root mean square error in NLOS conditions by up to 38%, while preserving the accuracy and precision of measurements in LOS conditions.
更多查看译文
AI 理解论文
溯源树
样例
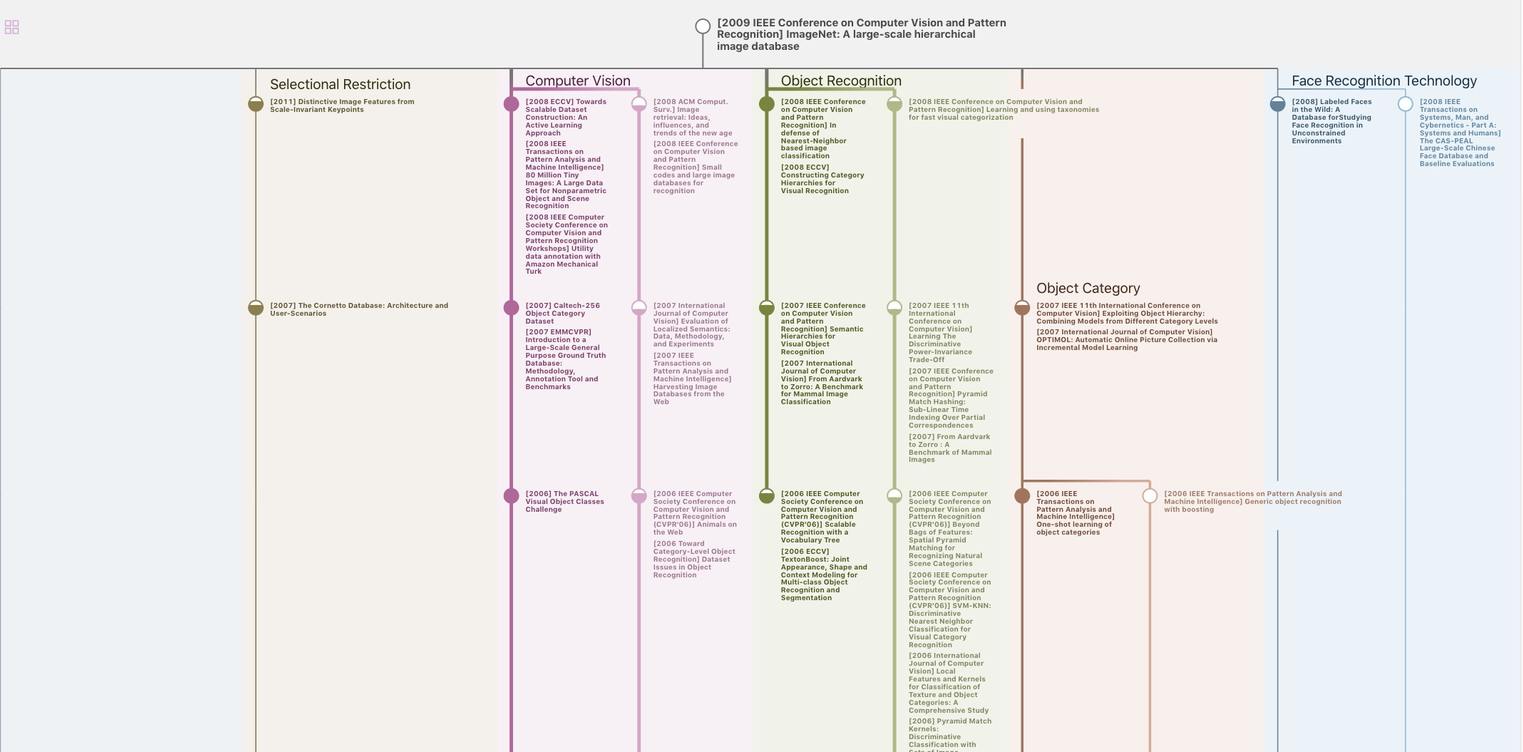
生成溯源树,研究论文发展脉络
Chat Paper
正在生成论文摘要