Abstract 011: Cardiovascular Risk Prediction Using Machine Learning In A Large Japanese Cohort
Circulation(2021)
摘要
Introduction: Screening for cardiovascular diseases (CVD) at middle age entails precise event prediction to orient risk stratification, resource allocation and insurance policy. Machine learning may be useful to characterize CVD risk and predict outcomes by identifying unique markers of incident CVD. We tested the ability of random survival forests (RSF) to identify the most important markers of incident CVD among adults enrolled in a mandatory screening program. Methods: We examined a dataset comprising annual health checkup, medication, and disease outcome data on 154,957 adults over the age of 40, collected by Toshiba between 2011 and 2017. Health checkup data included laboratory measurements of biomarkers, health history, and lifestyle questionnaires. CVD outcomes, classified as any of acute ischemic heart disease, myocardial infarction, angina pectoris and atherosclerotic heart disease, were recorded after initial health checkup using ICD-10 coding. In the absence of CVD outcomes, subjects’ latest available health check visit was used as the censoring date. Data was split into training (70%, n=108,470) and test (30%, n=46,487) sets, with RSF utilized to impute missing covariate data and determine the characteristics most predictive of CVD outcomes based on minimum depth of maximal subtree. Results: Subjects were 65% (100,376 of 154,957) male with a median age of 47 years at baseline. A total of 1,669 events occurred in the group over a median follow-up period of 5 years. The RSF error rate stabilized around 1000 trees; we grew the training forest with 1200. The c-index at 2, 4, and 6 years was 85%, 84%, and 82% respectively; prediction error calculated by Brier score was 16.4% at six years. The most important predictors of CVD outcomes were prior heart disease, history of CV procedures and age. HDL cholesterol, HBA1c levels, and use of anti-hypertensive medications were the next 3 most important predictors. Conclusions: Determination of key variables predictive of cardiac endpoints will help guide individuals, health practitioners and policy makers in identifying higher-risk subjects and implementing early interventions and testing to reduce risk. The RSF method greatly facilitates the development of a predictive algorithm to be used for these purposes.
更多查看译文
关键词
Atherosclerosis,Cardiovascular disease,Cardiovascular risk prediction,Coronary artery disease,Random survival forests
AI 理解论文
溯源树
样例
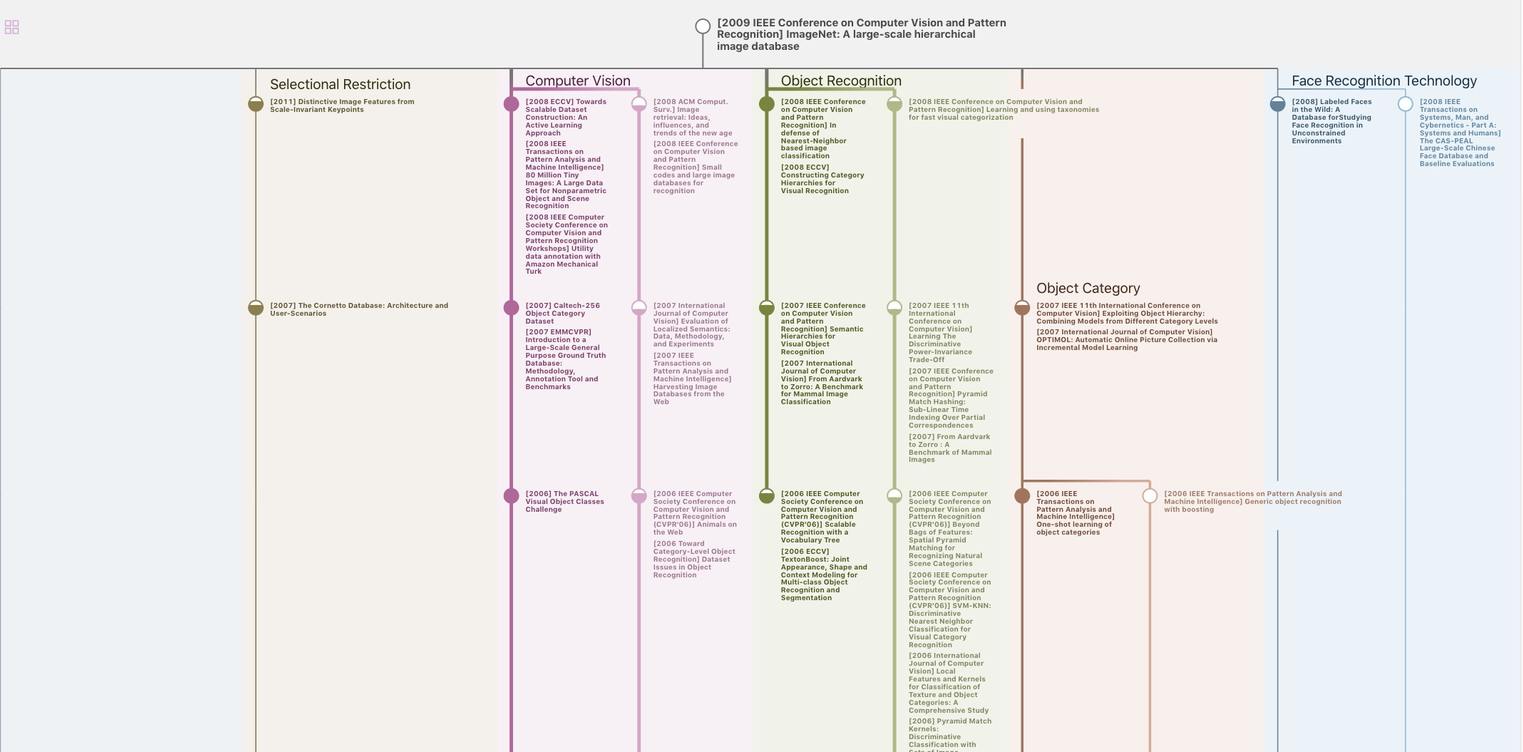
生成溯源树,研究论文发展脉络
Chat Paper
正在生成论文摘要