Enhancing Investigative Pattern Detection via Inexact Matching and Graph Databases
IEEE Transactions on Services Computing(2022)
摘要
Tracking individuals or groups based on their hidden and/or emergent behaviors is an indispensable task in homeland security, mental health evaluation, and consumer analytics. On-line and off-line communication patterns, behavior profiles and social relationships form complex dynamic evolving knowledge graphs. Investigative search involves capturing and mining such large-scale knowledge graphs for emergent profiles of interest. While graph databases facilitate efficient and scalable operations on complex heterogeneous graphs, dealing with incomplete, missing and/or inconsistent information and need for adaptive querying pose major challenges. We address these by proposing an inexact graph pattern matching method, which is implemented in a graph database with a scoring mechanism that helps identify hidden behavioral patterns. PINGS (
P
rocedures for
IN
vestigative
G
raph
S
earch), a graph database library of procedures for investigative graph search is presented. Results presented demonstrate the capability of detecting individuals/groups meeting query criteria as well as the iterative query performance in graph databases. We evaluate our approach on three datasets: a synthetically generated radicalization dataset, a publicly available patient’s ICU hospitalization stays dataset, and a crime dataset. These varied datasets demonstrate the wide-range applicability and the enhanced effectiveness of observing suspicious or latent trends in investigative domains.
更多查看译文
关键词
Social networks,data mining,graph pattern matching,inexact matching,investigative graph search,graph databases
AI 理解论文
溯源树
样例
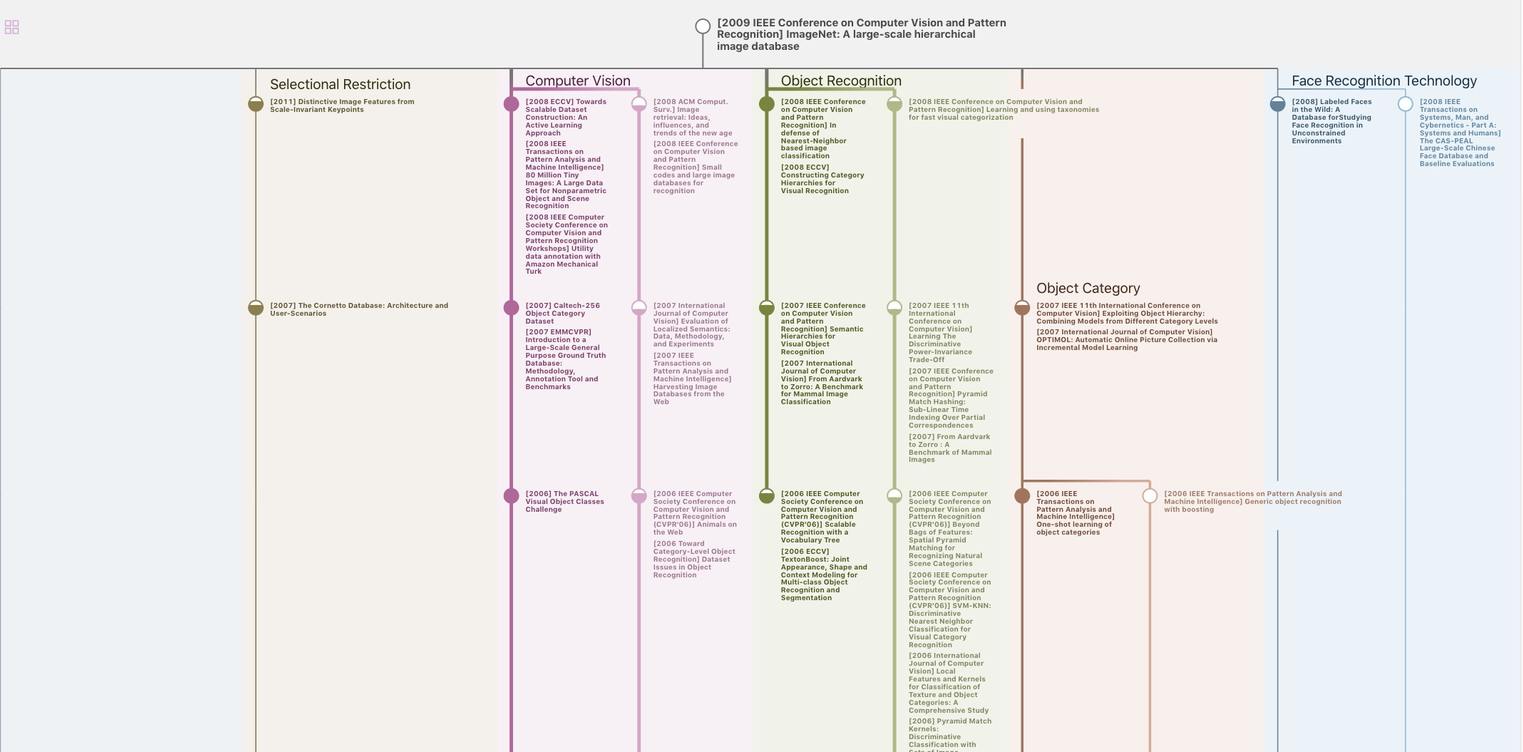
生成溯源树,研究论文发展脉络
Chat Paper
正在生成论文摘要