Learning a shared deformation space for efficient design-preserving garment transfer
Graphical Models(2021)
摘要
Garment transfer from a source mannequin to a shape-varying individual is a vital technique in computer graphics. Existing garment transfer methods are either time consuming or lack designed details especially for clothing with complex styles. In this paper, we propose a data-driven approach to efficiently transfer garments between two distinctive bodies while preserving the source design. Given two sets of simulated garments on a source body and a target body, we utilize the deformation gradients as the representation. Since garments in our dataset are with various topologies, we embed cloth deformation to the body. For garment transfer, the deformation is decomposed into two aspects, typically style and shape. An encoder-decoder network is proposed to learn a shared space which is invariant to garment style but related to the deformation of human bodies. For a new garment in a different style worn by the source human, our method can efficiently transfer it to the target body with the shared shape deformation, meanwhile preserving the designed details. We qualitatively and quantitatively evaluate our method on a diverse set of 3D garments that showcase rich wrinkling patterns. Experiments show that the transferred garments can preserve the source design even if the target body is quite different from the source one.
更多查看译文
关键词
Garment transfer,Cloth deformation,Shape analysis
AI 理解论文
溯源树
样例
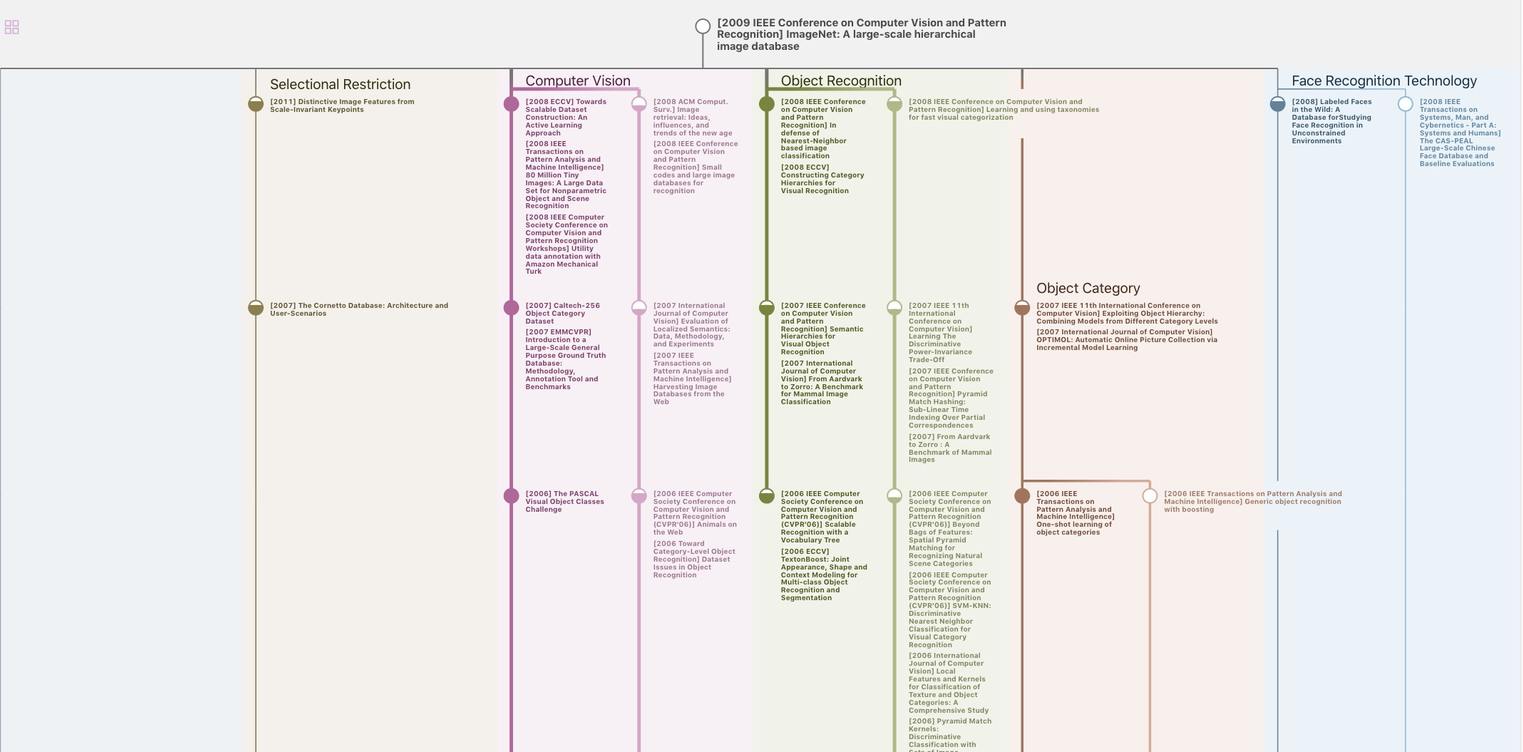
生成溯源树,研究论文发展脉络
Chat Paper
正在生成论文摘要