Intelligent bearing fault diagnosis based on Teager energy operator demodulation and multiscale compressed sensing deep autoencoder
Measurement(2021)
摘要
Accurate fault diagnosis is essential to ensure the stability and reliability of machine systems. Deep learning (DL) techniques have demonstrated potential in realizing intelligent fault diagnosis. However, the obtained bearing signals in the actual environment involve various interferences and are consistently nonstationary. Moreover, it is difficult to simultaneously acquire a large amount of balanced data to ensure suitable training. Consequently, traditional DL-based methods cannot achieve a satisfactory diagnostic accuracy. To overcome this problem and enhance the diagnostic performance in complex situations, this paper proposes a method to realize effective intelligent bearing fault diagnosis. A novel index is established to evaluate the quality of the demodulation spectra, along with a novel compressed sensing deep autoencoder based on compressed sensing. Additionally, a multiscale neural network architecture incorporating a multiscale coarse-grained procedure is adopted. Experimental results and comparative analysis with existing intelligent diagnosis methods demonstrate the effectiveness and robustness of the proposed method.
更多查看译文
关键词
Bearing fault diagnosis,Teager energy operator,Deep autoencoder,Compressed sensing,Multiscale feature extraction
AI 理解论文
溯源树
样例
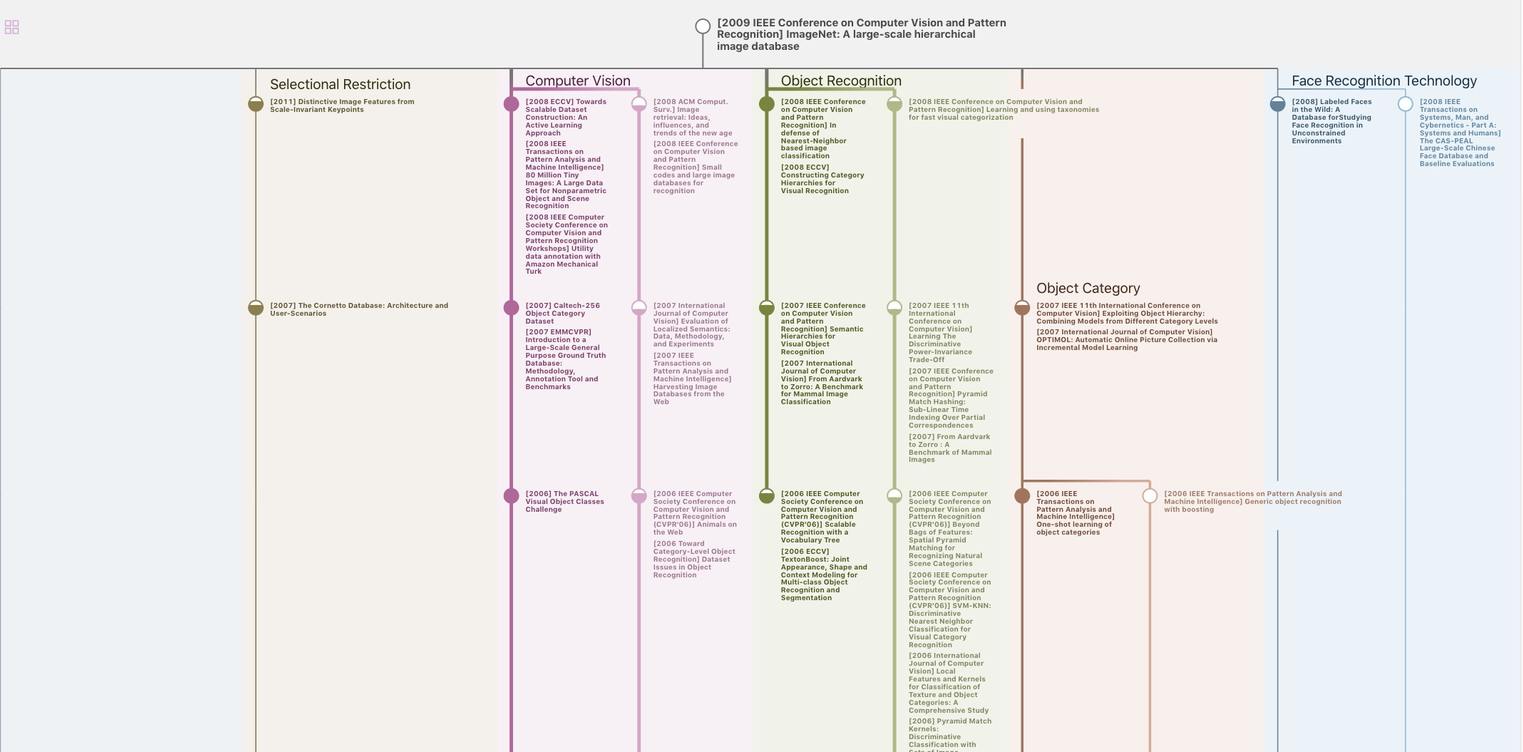
生成溯源树,研究论文发展脉络
Chat Paper
正在生成论文摘要