Assessing Map Accuracy From A Suite Of Site-Specific, Non-Site Specific, And Spatial Distribution Approaches.
REMOTE SENSING OF ENVIRONMENT(2021)
摘要
Assessing a map's accuracy and efficacy requires careful consideration and calculation of a comprehensive set of measures, particularly when characteristics in geospatial datasets may be used for addressing multiple objectives. We describe a map accuracy assessment framework for categorical geospatial data over large areas that utilizes site-specific (plot-pixel), non-site specific (area estimates), and comparative accuracy (spatial and statistical distributions) methods. This comprehensive framework spans the range of approaches applied in most other studies, maximizing opportunities for both within- and between-study comparisons. The analysis framework incorporated recommended good practices for sampling design, response design, analysis protocols, and designbased inference for providing rigorous estimates of accuracy and precision. Further partitioning of disagreement allows users to assess the relative importance of spatial location versus total abundance of map classes to map error; we introduced a ratio of allocation disagreement to quantity disagreement for comparing relative importance of each across datasets. Assessment of fuzzy membership with linguistic scales may have minimal effect on overall accuracy, but can provide substantial improvement in user's accuracy and producer's accuracy for uncommon classes. We adapted non-site specific approaches to provide metrics and insights into spatial variability of map accuracy of thematic classes. Comparing estimates based on reference data and co-located samples of map data may reveal potential issues with reference data. We demonstrated example applications all components of the assessment framework for thematic maps of early successional forest and other land cover classes across the western Great Lakes region, USA.
更多查看译文
关键词
Assessment framework, Map validation
AI 理解论文
溯源树
样例
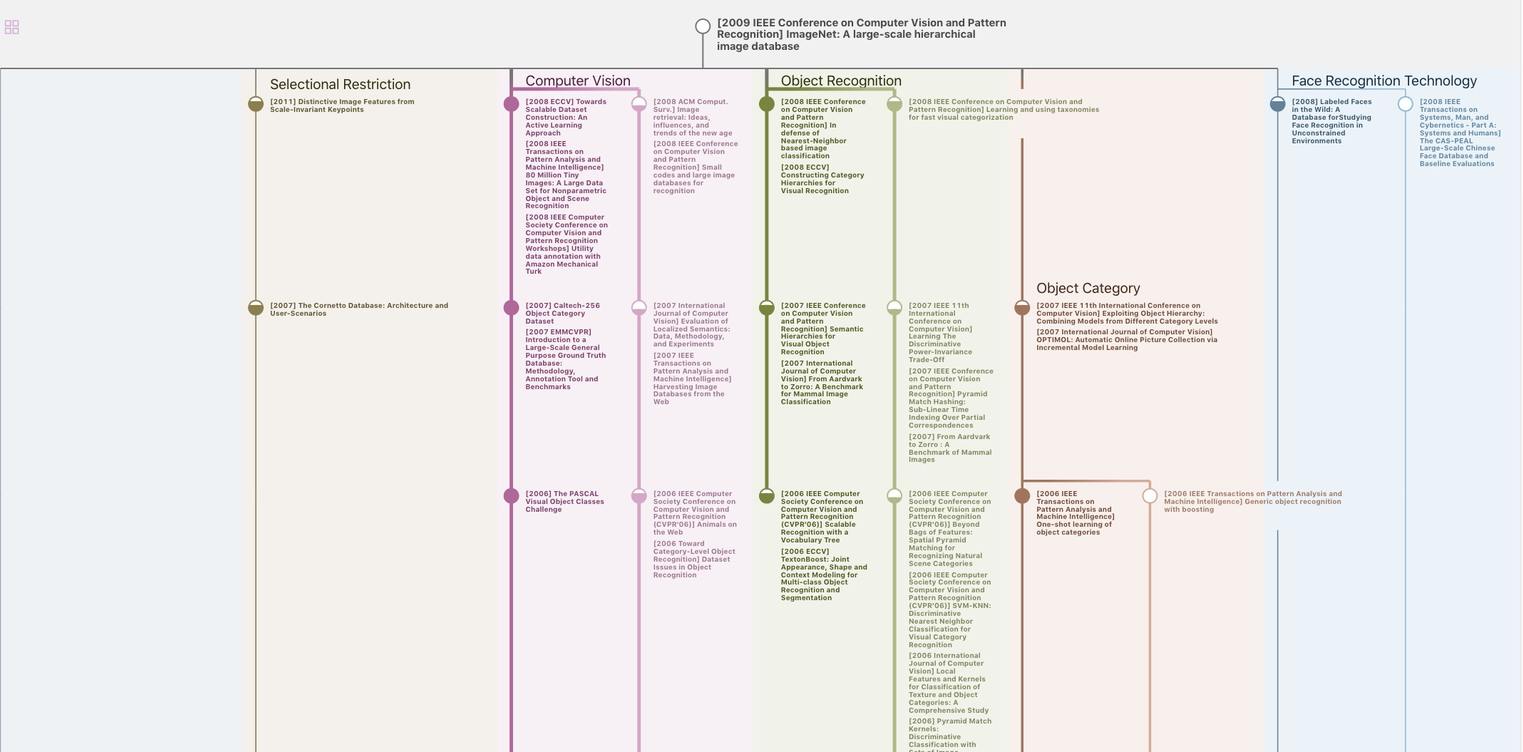
生成溯源树,研究论文发展脉络
Chat Paper
正在生成论文摘要