Robust Gaussian process regression with G-confluent likelihood
IFAC-PapersOnLine(2020)
摘要
Abstract For robust Gaussian process regression problems where the measurements are contaminated by outliers, a likelihood/measurement noise model with heavy-tailed distributions should be used to improve the prediction performance. In this paper, we propose to use a G-confluent distribution as the measurement noise model and a coordinate ascent variational inference method to infer the overall statistical model. In contrast with the commonly used Student’s t distribution, the G-confluent distribution can also be written as a Gaussian scale mixture, but its inverse scale follows a Beta distribution rather than a Gamma distribution, and its main advantage is that it is more flexible for modeling outliers while being equally suitable for variational inference. Numerical simulations based on benchmark data show that the G-confluent distribution performs better than or as well as the Student’s t distribution.
更多查看译文
关键词
Bayesian methods,Machine learning,Nonparametric methods,Gaussian process regression,Outliers,Variational inference
AI 理解论文
溯源树
样例
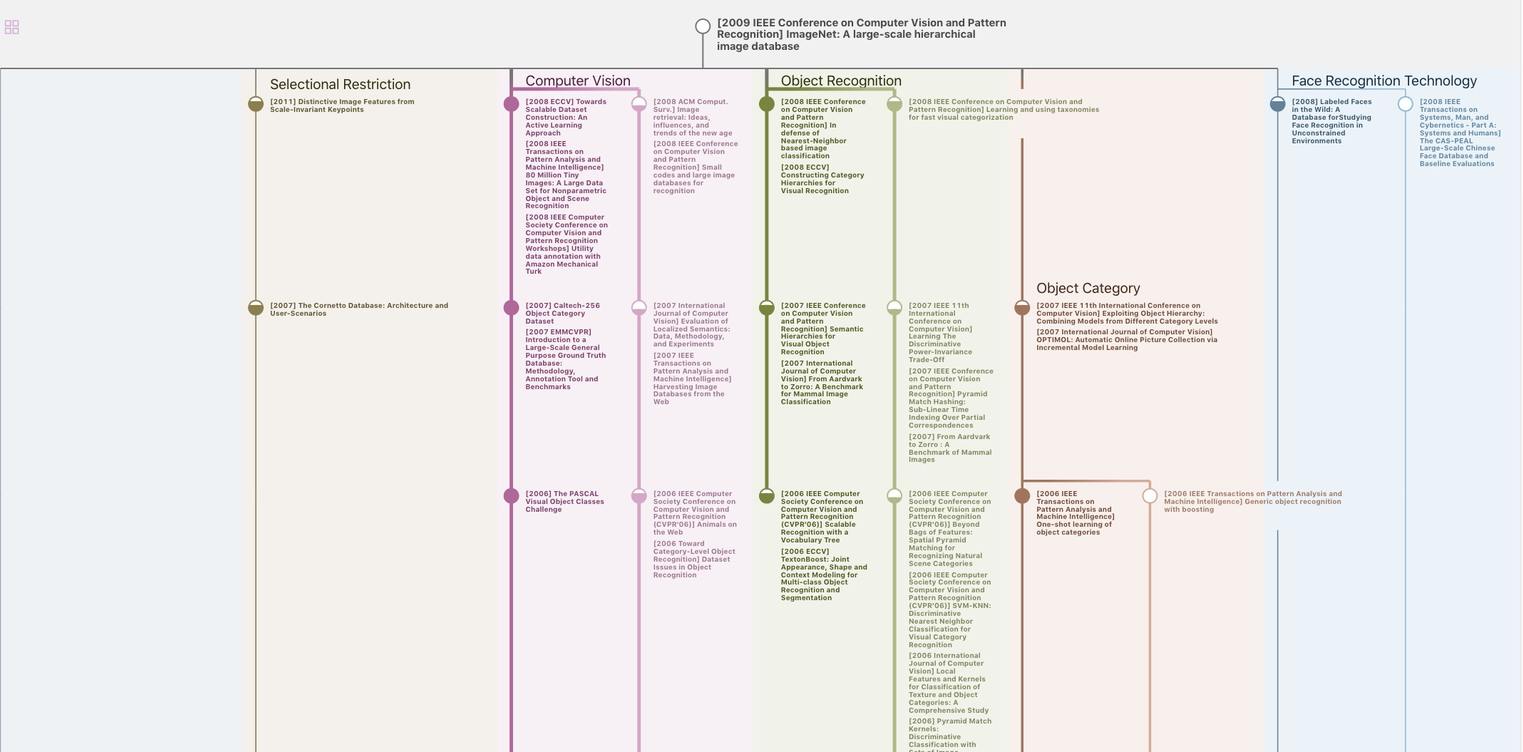
生成溯源树,研究论文发展脉络
Chat Paper
正在生成论文摘要