RF-Based Human Activity Recognition Using Signal Adapted Convolutional Neural Network
IEEE Transactions on Mobile Computing(2023)
摘要
Human activity recognition (HAR) plays a critical role in a wide range of real-world applications, and it is traditionally achieved via wearable sensing. Recently, to avoid the burden and discomfort caused by wearable devices, device-free approaches exploiting radio-frequency (RF) signals arise as a promising alternative for HAR. Most of the latest device-free approaches require training a large deep neural network model in either time or frequency domain, entailing extensive storage to contain the model and intensive computations to infer human activities. Consequently, even with some major advances on device-free HAR, current device-free approaches are still far from practical in real-world scenarios where the computation and storage resources possessed by, for example, edge devices, are limited. To overcome these weaknesses, we introduce HAR-SAnet which is a novel RF-based HAR framework. It adopts an original signal adapted convolutional neural network architecture: instead of feeding the handcraft features of RF signals into a classifier, HAR-SAnet fuses them adaptively from both time and frequency domains to design an end-to-end neural network model. We apply point-wise grouped convolution and depth-wise separable convolutions to confine the model scale and to speed up the inference execution time. The experiment results show that the recognition accuracy of HAR-SAnet substantially outperforms the state-of-the-art algorithms and systems.
更多查看译文
关键词
Human activity recognition,convolutional neural network,RF-sensing,impulse radio
AI 理解论文
溯源树
样例
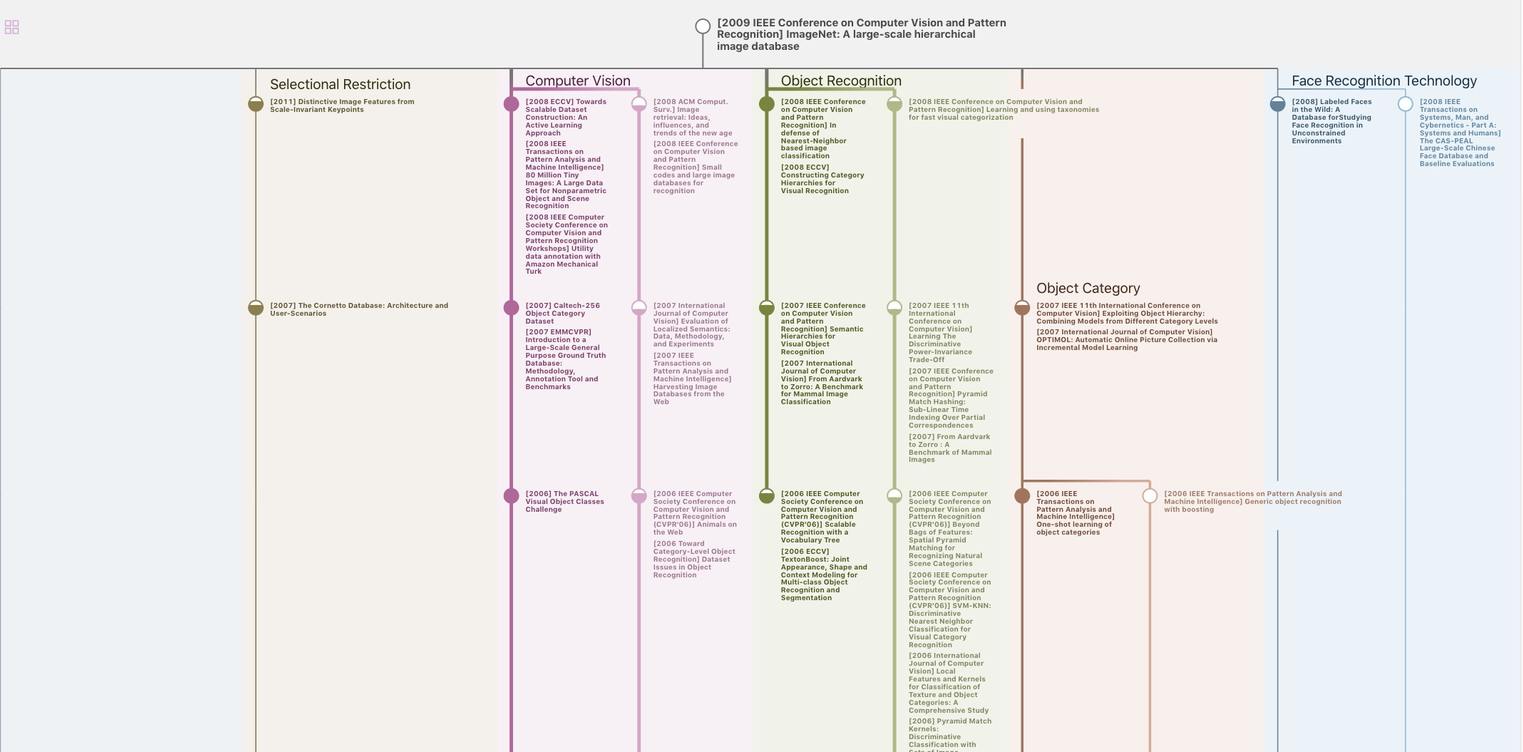
生成溯源树,研究论文发展脉络
Chat Paper
正在生成论文摘要