Uranium Oxide Synthetic Pathway Discernment through Unsupervised Morphological Analysis
Journal of Nuclear Materials(2021)
摘要
We present a novel unsupervised machine learning method for quantitative representation of scanning electron micrographs and its applications and performance for nuclear forensic analysis of uranium ore concentrates. The method uses a vector quantizing variational autoencoder followed by a histogram operation to encode a micrograph into a single dimensional representation, called the feature vector. The method requires no extant labeling of the data and can be applied over large datasets of micrographs with minimal human interaction. The representations generated are broadly descriptive of each micrograph and the microstructure of the material imaged. In the case of uranium ore concentrate analysis, the representations were amenable to processing reagent and ore concentrate species classification with accuracy of 81.8%, which is competitive with state-of-the-art supervised networks [1]. The representations were also able to classify previously processing routes not included in the training set, were able to classify imaging parameters such as magnification (to 76.0% accuracy), were able to classify fine grained process parameters such as calcining temperature (to 74.4% accuracy), and their informatic properties indicate that they are generally descriptive of the image represented. This method can be applied across microstructure analysis fields to perform quantitative analysis without the need for labor intensive and possibly biased human analysis.
更多查看译文
关键词
Scanning Electron Microscopy,Vector Quantizing Variational Autoencoder,Unsupervised Learning,Nuclear Forensic Analysis
AI 理解论文
溯源树
样例
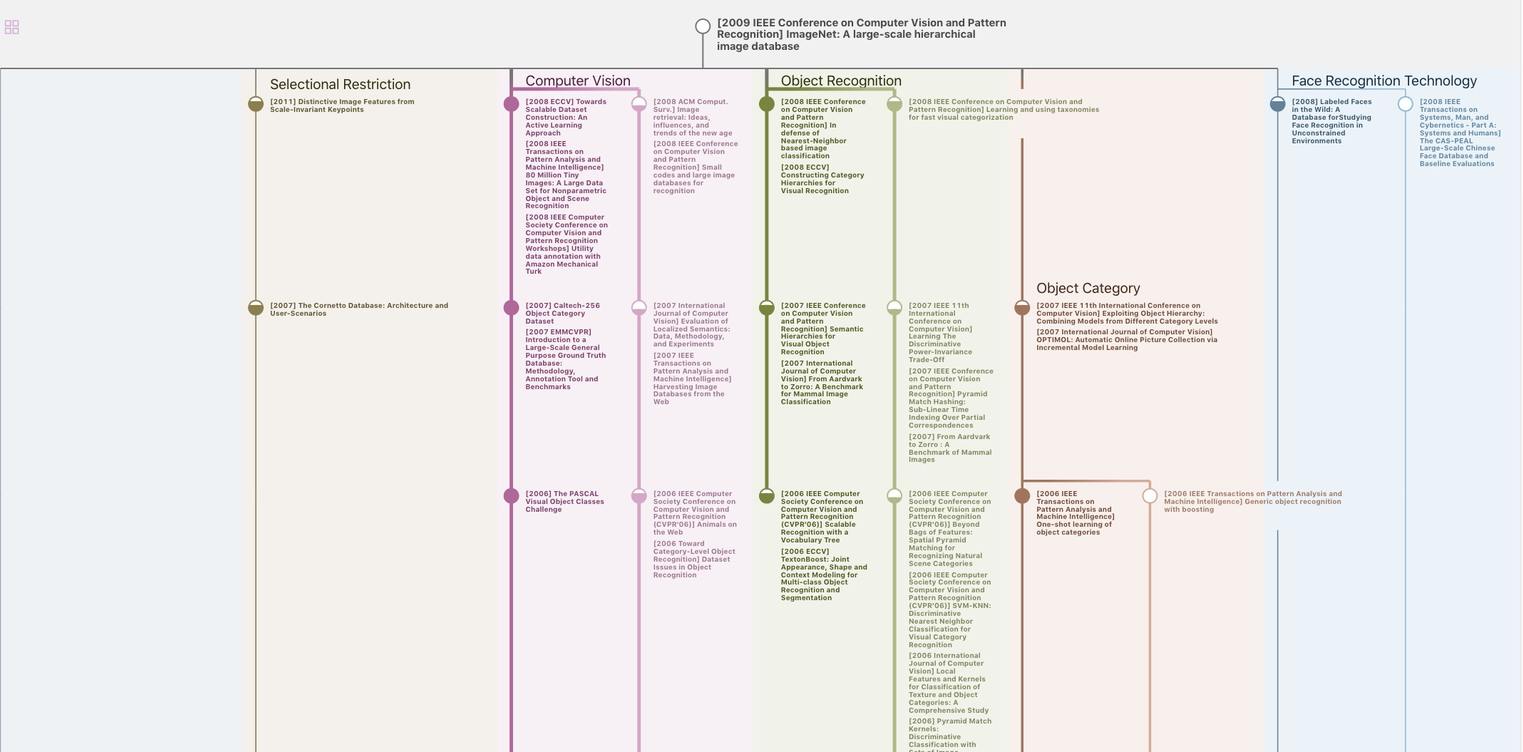
生成溯源树,研究论文发展脉络
Chat Paper
正在生成论文摘要