Attribute-Guided Network Sampling Mechanisms
ACM Transactions on Knowledge Discovery from Data(2021)
摘要
AbstractThis article introduces a novel task-independent sampler for attributed networks. The problem is important because while data mining tasks on network content are common, sampling on internet-scale networks is costly. Link-trace samplers such as Snowball sampling, Forest Fire, Random Walk, and Metropolis–Hastings Random Walk are widely used for sampling from networks. The design of these attribute-agnostic samplers focuses on preserving salient properties of network structure, and are not optimized for tasks on node content. This article has three contributions. First, we propose a task-independent, attribute aware link-trace sampler grounded in Information Theory. Our sampler greedily adds to the sample the node with the most informative (i.e., surprising) neighborhood. The sampler tends to rapidly explore the attribute space, maximally reducing the surprise of unseen nodes. Second, we prove that content sampling is an NP-hard problem. A well-known algorithm best approximates the optimization solution within 1 − 1/e, but requires full access to the entire graph. Third, we show through empirical counterfactual analysis that in many real-world datasets, network structure does not hinder the performance of surprise based link-trace samplers. Experimental results over 18 real-world datasets reveal: surprise-based samplers are sample efficient and outperform the state-of-the-art attribute-agnostic samplers by a wide margin (e.g., 45% performance improvement in clustering tasks).
更多查看译文
关键词
Task-independent sampling, attributed networks, data mining
AI 理解论文
溯源树
样例
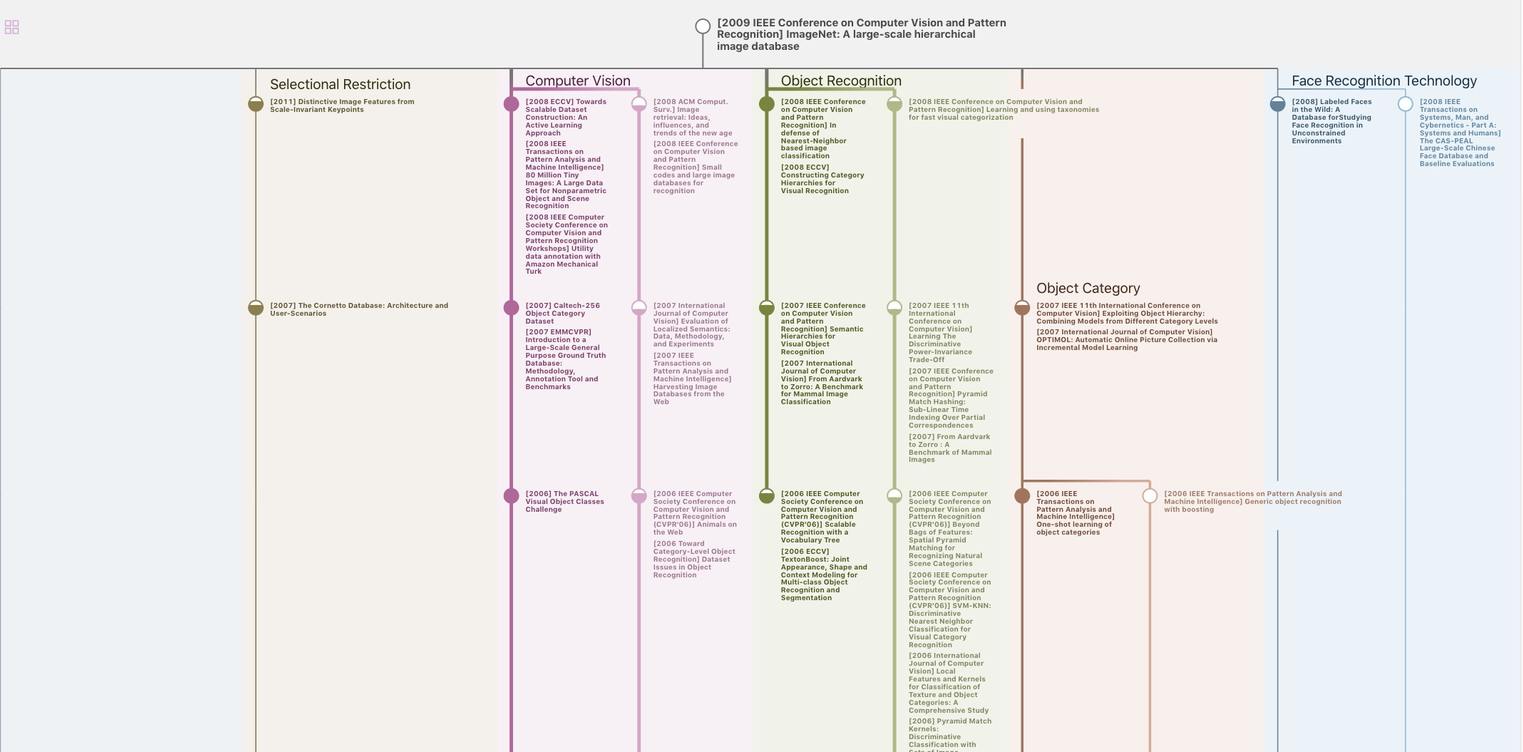
生成溯源树,研究论文发展脉络
Chat Paper
正在生成论文摘要