AppBuddy: Learning to Accomplish Tasks in Mobile Apps via Reinforcement Learning.
Canadian Conference on Artificial Intelligence (AI)(2021)
摘要
Human beings, even small children, quickly become adept at figuring out how to use applications on their mobile devices. Learning to use a new app is often achieved via trial-and-error, accelerated by transfer of knowledge from past experiences with like apps. The prospect of building a smarter smartphone - one that can learn how to achieve tasks using mobile apps - is tantalizing. In this paper we explore the use of Reinforcement Learning (RL) with the goal of advancing this aspiration. We introduce an RL-based framework for learning to accomplish tasks in mobile apps. RL agents are provided with states derived from the underlying representation of on-screen elements, and rewards that are based on progress made in the task. Agents can interact with screen elements by tapping or typing. Our experimental results, over a number of mobile apps, show that RL agents can learn to accomplish multi-step tasks, as well as achieve modest generalization across different apps. More generally, we develop a platform which addresses several engineering challenges to enable an effective RL training environment. Our AppBuddy platform is compatible with OpenAI Gym and includes a suite of mobile apps and benchmark tasks that supports a diversity of RL research in the mobile app setting.
更多查看译文
关键词
mobile apps,reinforcement
AI 理解论文
溯源树
样例
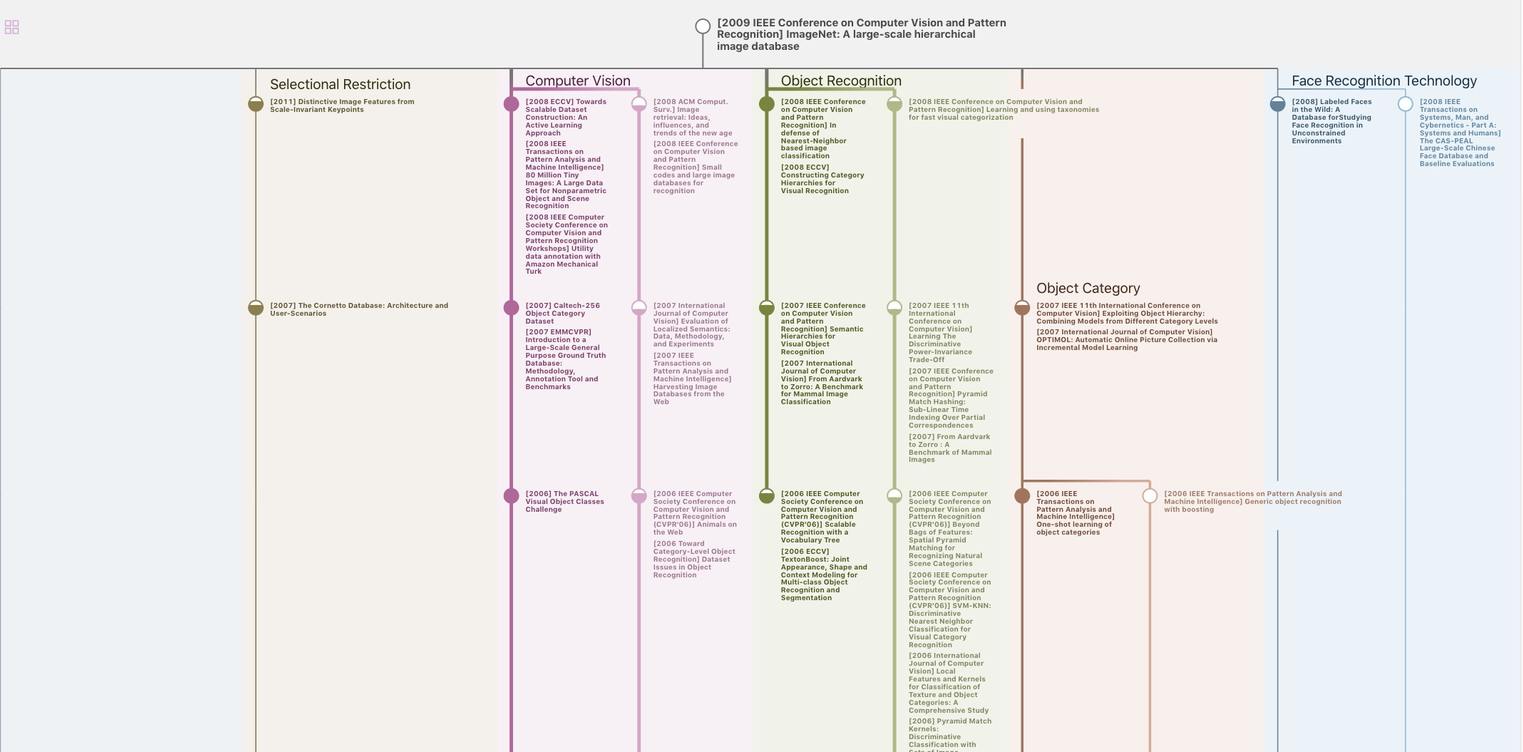
生成溯源树,研究论文发展脉络
Chat Paper
正在生成论文摘要