Investigating The Impact Of The Bit Depth Of Fluorescence-Stained Images On The Performance Of Deep Learning-Based Nuclei Instance Segmentation
DIAGNOSTICS(2021)
摘要
Nuclei instance segmentation can be considered as a key point in the computer-mediated analysis of histological fluorescence-stained (FS) images. Many computer-assisted approaches have been proposed for this task, and among them, supervised deep learning (DL) methods deliver the best performances. An important criterion that can affect the DL-based nuclei instance segmentation performance of FS images is the utilised image bit depth, but to our knowledge, no study has been conducted so far to investigate this impact. In this work, we released a fully annotated FS histological image dataset of nuclei at different image magnifications and from five different mouse organs. Moreover, by different pre-processing techniques and using one of the state-of-the-art DL-based methods, we investigated the impact of image bit depth (i.e., eight bits vs. sixteen bits) on the nuclei instance segmentation performance. The results obtained from our dataset and another publicly available dataset showed very competitive nuclei instance segmentation performances for the models trained with 8 bit and 16 bit images. This suggested that processing 8 bit images is sufficient for nuclei instance segmentation of FS images in most cases. The dataset including the raw image patches, as well as the corresponding segmentation masks is publicly available in the published GitHub repository.
更多查看译文
关键词
bit depth, nuclei segmentation, fluorescence staining, medical image analysis, computational pathology, deep learning
AI 理解论文
溯源树
样例
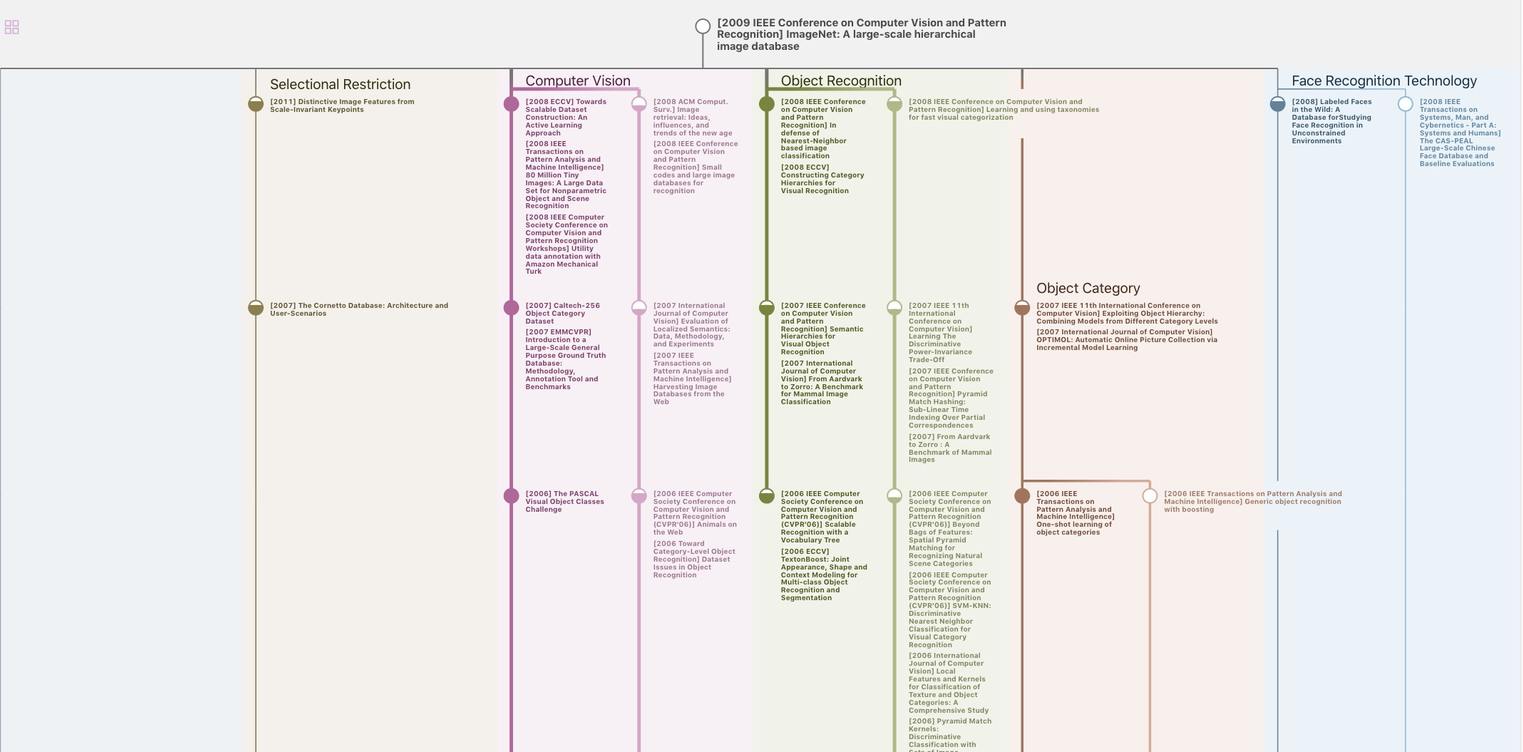
生成溯源树,研究论文发展脉络
Chat Paper
正在生成论文摘要