Piston Error Measurement For Segmented Telescopes With An Artificial Neural Network
SENSORS(2021)
摘要
A piston error detection method is proposed based on the broadband intensity distribution on the image plane using a back-propagation (BP) artificial neural network. By setting a mask with a sparse circular clear multi-subaperture configuration in the exit pupil plane of a segmented telescope to fragment the pupil, the relation between the piston error of segments and amplitude of the modulation transfer function (MTF) sidelobes is strictly derived according to the Fourier optics principle. Then the BP artificial neural network is utilized to establish the mapping relation between them, where the amplitudes of the MTF sidelobes directly calculated from theoretical relationship and the introduced piston errors are used as inputs and outputs respectively to train the network. With the well trained-network, the piston errors are measured to a good precision using one in-focused broadband image without defocus division as input, and the capture range achieving the coherence length of the broadband light is available. Adequate simulations demonstrate the effectiveness and accuracy of the proposed method; the results show that the trained network has high measurement accuracy, wide detection range, quite good noise immunity and generalization ability. This method provides a feasible and easily implemented way to measure piston error and can simultaneously detect the multiple piston errors of the entire aperture of the segmented telescope.
更多查看译文
关键词
piston error detection, segmented telescope, BP artificial neural network, modulation transfer function
AI 理解论文
溯源树
样例
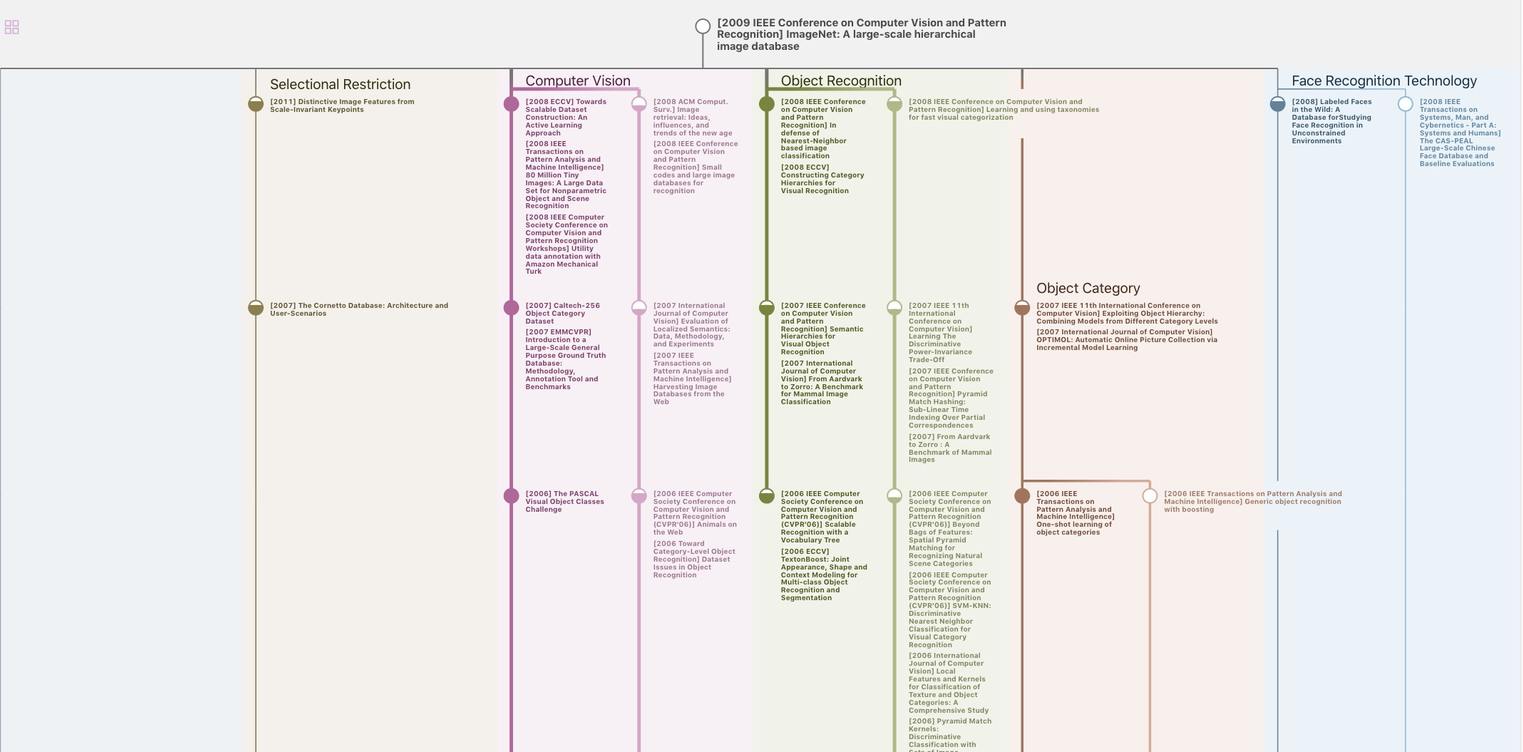
生成溯源树,研究论文发展脉络
Chat Paper
正在生成论文摘要