Neural Estimator Of Information For Time-Series Data With Dependency
ENTROPY(2021)
摘要
Novel approaches to estimate information measures using neural networks are well-celebrated in recent years both in the information theory and machine learning communities. These neural-based estimators are shown to converge to the true values when estimating mutual information and conditional mutual information using independent samples. However, if the samples in the dataset are not independent, the consistency of these estimators requires further investigation. This is of particular interest for a more complex measure such as the directed information, which is pivotal in characterizing causality and is meaningful over time-dependent variables. The extension of the convergence proof for such cases is not trivial and demands further assumptions on the data. In this paper, we show that our neural estimator for conditional mutual information is consistent when the dataset is generated with samples of a stationary and ergodic source. In other words, we show that our information estimator using neural networks converges asymptotically to the true value with probability one. Besides universal functional approximation of neural networks, a core lemma to show the convergence is Birkhoff's ergodic theorem. Additionally, we use the technique to estimate directed information and demonstrate the effectiveness of our approach in simulations.
更多查看译文
关键词
neural networks, conditional mutual information, directed information, Markov source, variational bound
AI 理解论文
溯源树
样例
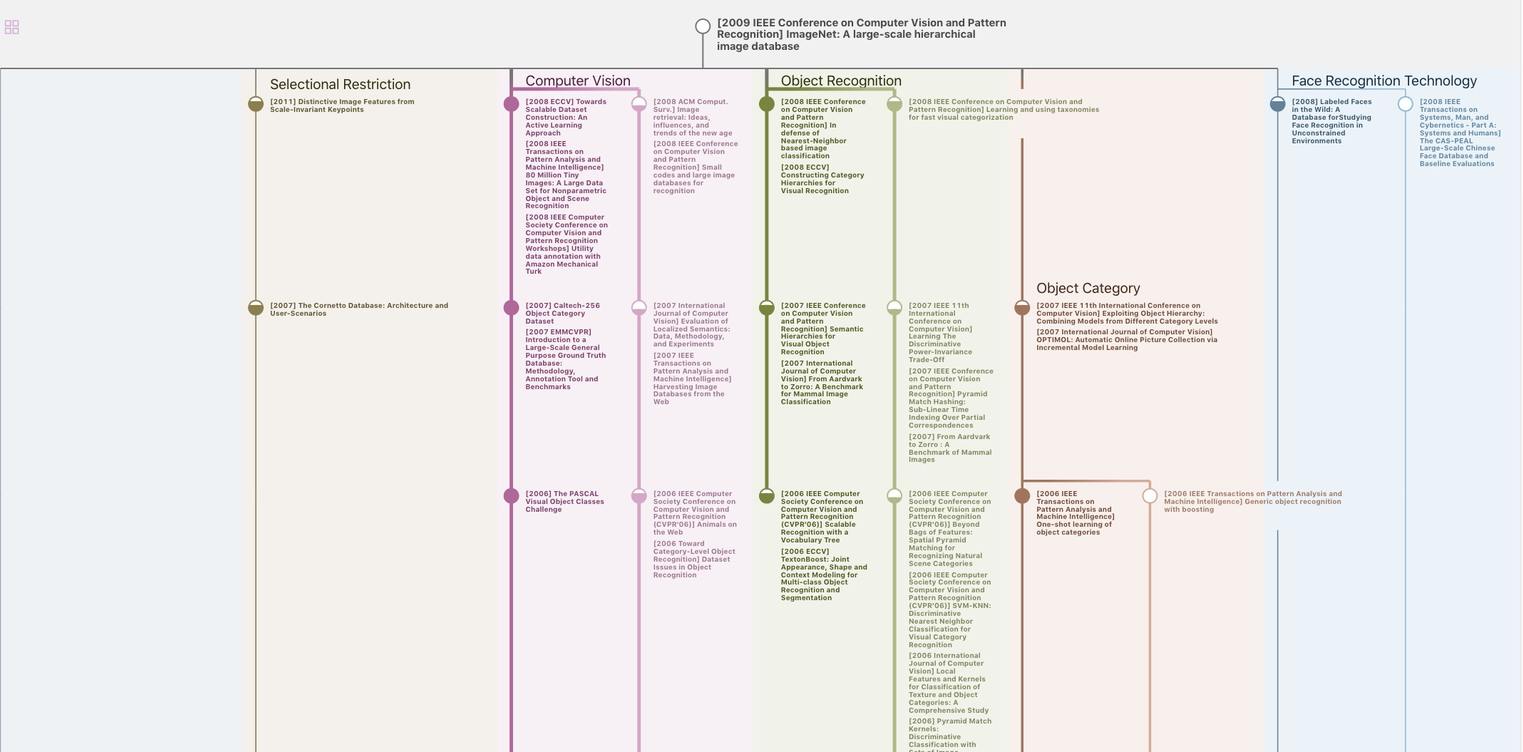
生成溯源树,研究论文发展脉络
Chat Paper
正在生成论文摘要