Multi-objective Optimization for Virtual Machine Allocation in Computational Scientific Workflow under Uncertainty
CLOSER: PROCEEDINGS OF THE 11TH INTERNATIONAL CONFERENCE ON CLOUD COMPUTING AND SERVICES SCIENCE(2021)
摘要
Providing resources and services from various cloud providers is now an increasingly promising paradigm. Workflow applications are becoming increasingly computation-intensive or data-intensive, with resource allocation being maintained in terms of pay per usage. In this paper, a multi-objective optimization study for scientific workflow in a cloud environment is proposed. The aim is to minimize execution time and purchasing cost simultaneously while satisfying the demand requirements of customers. The uncertainties present in the model are identified and handled using a well-known technique called Chance Constrained Programming (CCP) for real-world implementation. The model is solved using the Non-dominated Sorting Genetic Algorithm - II (NSGA-II). This comprehensive study shows that the solutions obtained on considering uncertainties vary from the deterministic case. Based on the probability of constraint satisfaction, the objective functions improve but at the cost of reliability of the solution.
更多查看译文
关键词
Cloud Computing, Scientific Workflow, Resource Allocation, Multi-objective Optimization, NSGA-II, Chance Constrained Programming
AI 理解论文
溯源树
样例
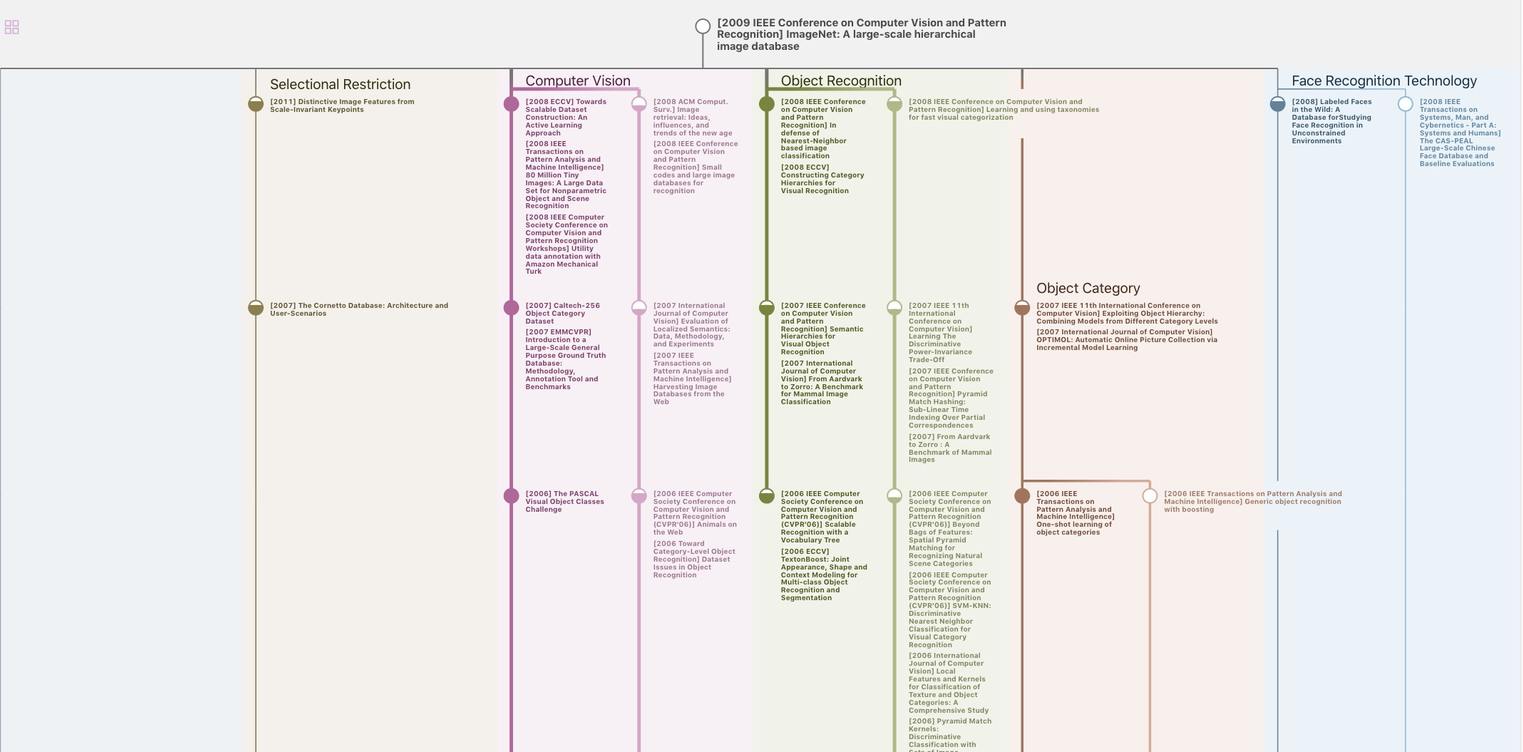
生成溯源树,研究论文发展脉络
Chat Paper
正在生成论文摘要