Trajectory modeling via random utility inverse reinforcement learning
INFORMATION SCIENCES(2024)
摘要
We consider the problem of modeling trajectories of drivers in a road network from the perspective of inverse reinforcement learning. Cars are detected by sensors placed on sparsely distributed points on the street network of a city. As rational agents, drivers are trying to maximize some reward function unknown to an external observer. We apply the concept of random utility from econometrics to model the unknown reward function as a function of observed and unobserved features. In contrast to current inverse reinforcement learning approaches, we do not assume that agents act according to a stochastic policy; rather, we assume that agents act according to a deterministic optimal policy and show that randomness in data arises because the exact rewards are not fully observed by an external observer. We introduce the concept of extended state to cope with unobserved features and develop a Markov decision process formulation of drivers decisions. We present theoretical results which guarantee the existence of solutions and show that maximum entropy inverse reinforcement learning is a particular case of our approach. Finally, we illustrate Bayesian inference on model parameters through a case study with real trajectory data from a large city in Brazil.
更多查看译文
关键词
Trajectory modeling,Inverse reinforcement learning,Random utility theory,External sensor data
AI 理解论文
溯源树
样例
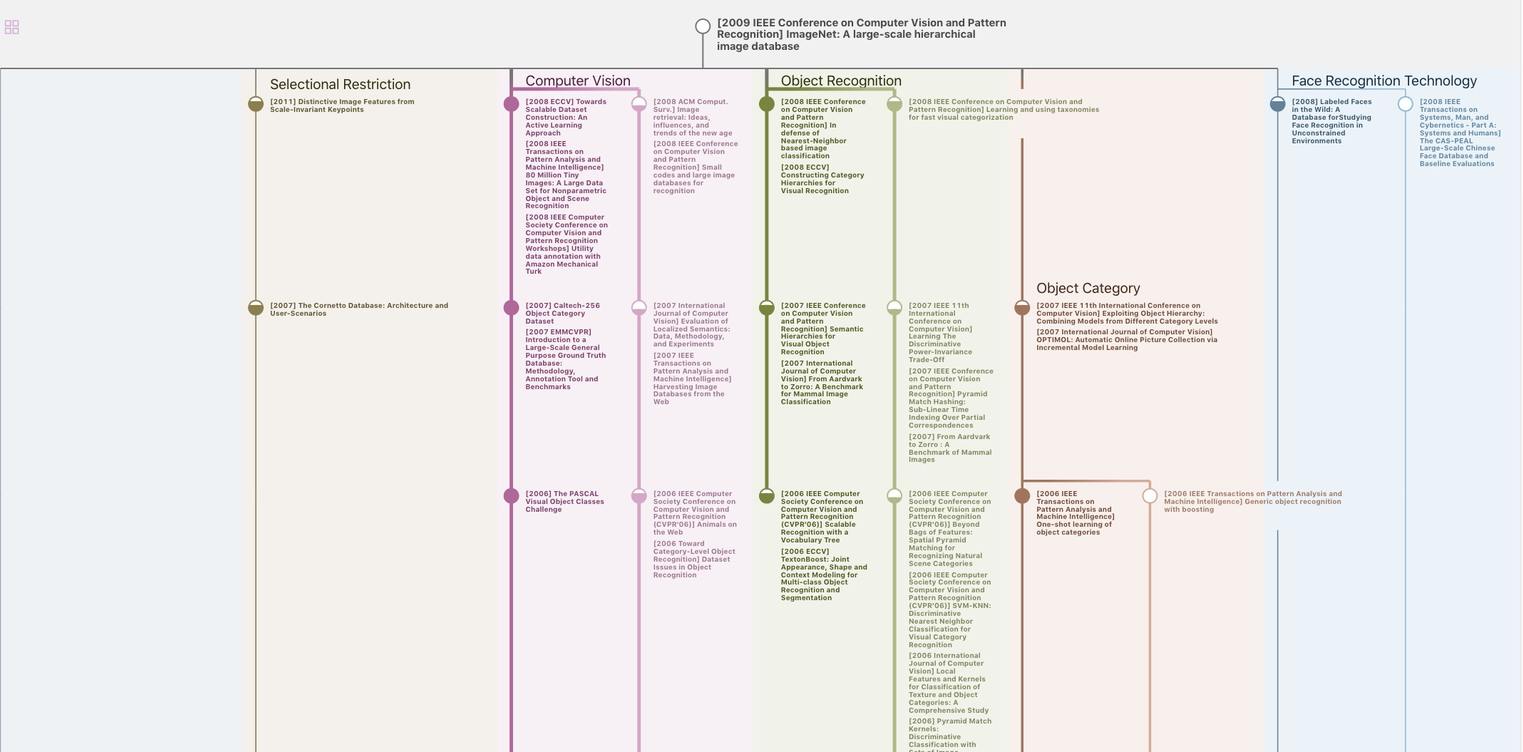
生成溯源树,研究论文发展脉络
Chat Paper
正在生成论文摘要