FedScale: Benchmarking Model and System Performance of Federated Learning
SOSP(2021)
摘要
We present FedScale, a diverse set of challenging and realistic benchmark datasets to facilitate scalable, comprehensive, and reproducible federated learning (FL) research. FedScale datasets are large-scale, encompassing a diverse range of important FL tasks, such as image classification, object detection, language modeling, speech recognition, and reinforcement learning. For each dataset, we provide a unified evaluation protocol using realistic data splits and evaluation metrics. To meet the pressing need for reproducing realistic FL at scale, we have also built an efficient evaluation platform to simplify and standardize the process of FL experimental setup and model evaluation. Our evaluation platform provides flexible APIs to implement new FL algorithms and include new execution backends with minimal developer efforts. Finally, we perform indepth benchmark experiments on these datasets. Our experiments suggest that FedScale presents significant challenges of heterogeneity-aware co-optimizations of the system and statistical efficiency under realistic FL characteristics, indicating fruitful opportunities for future research. FedScale is open-source with permissive licenses and actively maintained, and we welcome feedback and contributions from the community.
更多查看译文
关键词
benchmarking model,learning,system performance
AI 理解论文
溯源树
样例
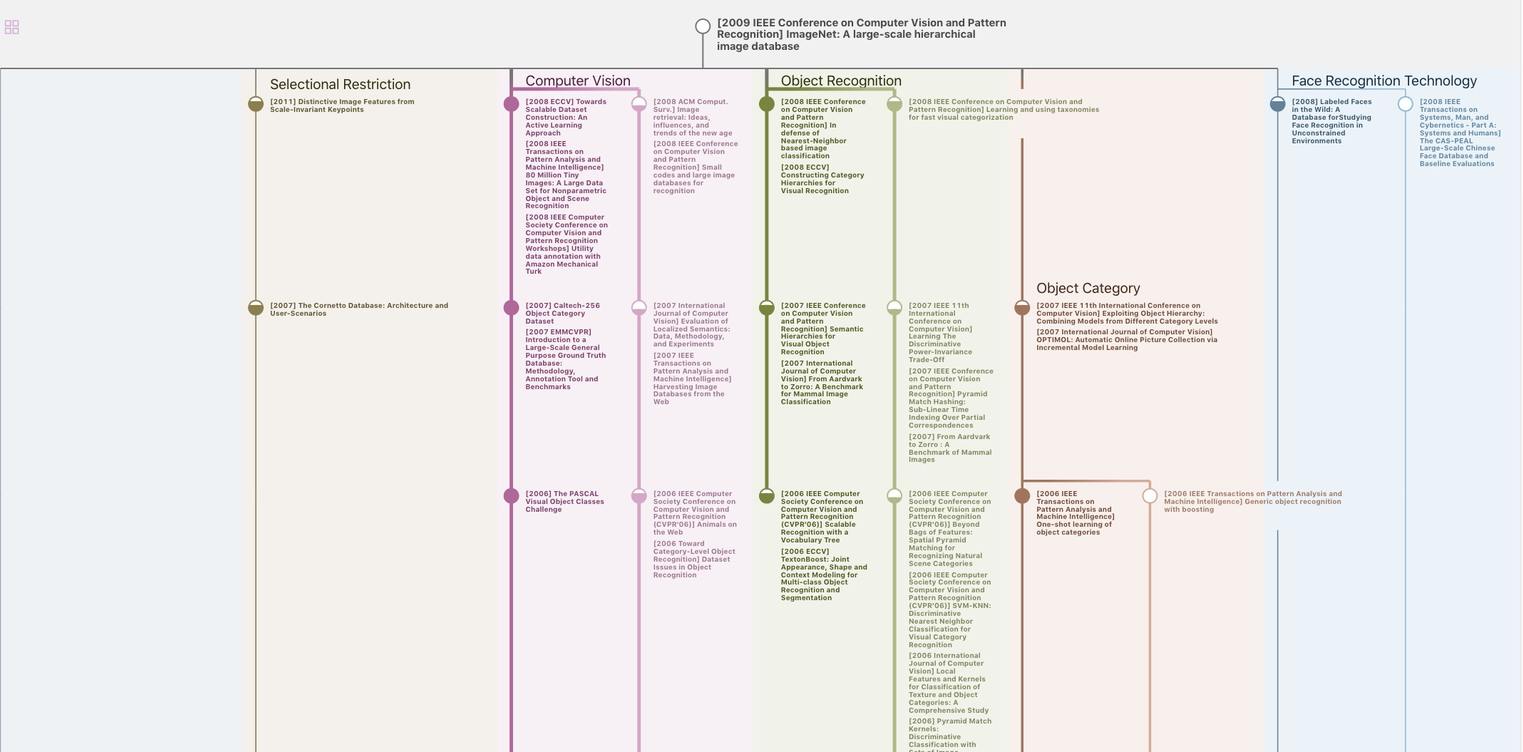
生成溯源树,研究论文发展脉络
Chat Paper
正在生成论文摘要