Continual World: A Robotic Benchmark For Continual Reinforcement Learning.
Annual Conference on Neural Information Processing Systems(2021)
摘要
Continual learning (CL) --- the ability to continuously learn, building on previously acquired knowledge --- is a natural requirement for long-lived autonomous reinforcement learning (RL) agents. While building such agents, one needs to balance opposing desiderata, such as constraints on capacity and compute, the ability to not catastrophically forget, and to exhibit positive transfer on new tasks. Understanding the right trade-off is conceptually and computationally challenging, which we argue has led the community to overly focus on catastrophic forgetting. In response to these issues, we advocate for the need to prioritize forward transfer and propose Continual World, a benchmark consisting of realistic and meaningfully diverse robotic tasks built on top of Meta-World as a testbed. Following an in-depth empirical evaluation of existing CL methods, we pinpoint their limitations and highlight unique algorithmic challenges in the RL setting. Our benchmark aims to provide a meaningful and computationally inexpensive challenge for the community and thus help better understand the performance of existing and future solutions. Information about the benchmark, including the open-source code, is available at https://sites.google.com/view/continualworld.
更多查看译文
关键词
continual world,robotic benchmark,learning
AI 理解论文
溯源树
样例
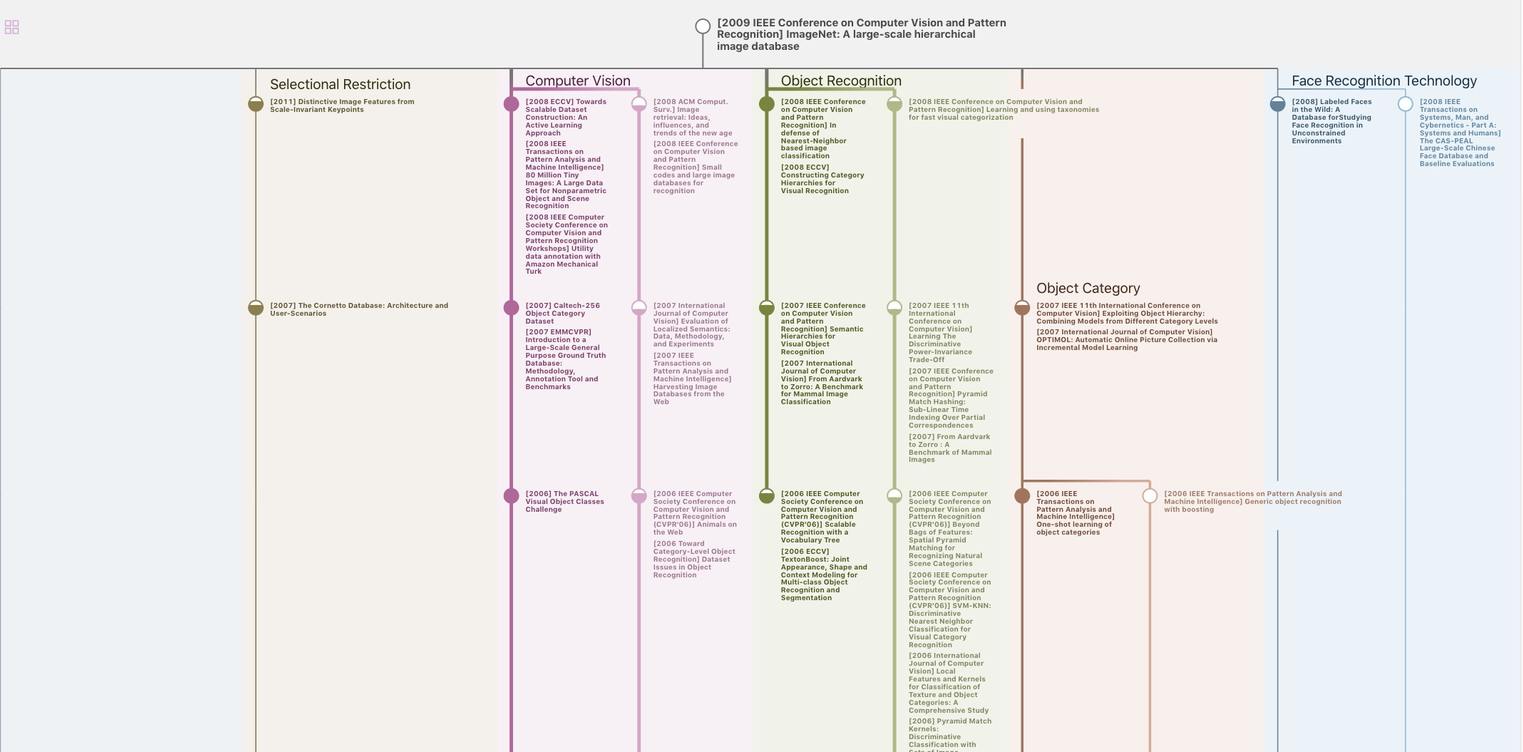
生成溯源树,研究论文发展脉络
Chat Paper
正在生成论文摘要