Improving Current Glycated Hemoglobin Prediction In Adults: Use Of Machine Learning Algorithms With Electronic Health Records
JMIR MEDICAL INFORMATICS(2021)
摘要
Background: Predicting the risk of glycated hemoglobin (HbA(1c)) elevation can help identify patients with the potential for developing serious chronic health problems, such as diabetes. Early preventive interventions based upon advanced predictive models using electronic health records data for identifying such patients can ultimately help provide better health outcomes.Objective: Our study investigated the performance of predictive models to forecast HbA1c elevation levels by employing several machine learning models. We also examined the use of patient electronic health record longitudinal data in the performance of the predictive models. Explainable methods were employed to interpret the decisions made by the black box models.Methods: This study employed multiple logistic regression, random forest, support vector machine, and logistic regression models, as well as a deep learning model (multilayer perceptron) to classify patients with normal (<5.7%) and elevated (>= 5.7%) levels of HbA(1c). We also integrated current visit data with historical (longitudinal) data from previous visits. Explainable machine learning methods were used to interrogate the models and provide an understanding of the reasons behind the decisions made by the models. All models were trained and tested using a large data set from Saudi Arabia with 18,844 unique patient records.Results: The machine learning models achieved promising results for predicting current HbA(1c) elevation risk. When coupled with longitudinal data, the machine learning models outperformed the multiple logistic regression model used in the comparative study. The multilayer perceptron model achieved an accuracy of 83.22% for the area under receiver operating characteristic curve when used with historical data. All models showed a close level of agreement on the contribution of random blood sugar and age variables with and without longitudinal data.Conclusions: This study shows that machine learning models can provide promising results for the task of predicting current HbA(1c) levels (>= 5.7% or less). Using patients' longitudinal data improved the performance and affected the relative importance for the predictors used. The models showed results that are consistent with comparable studies.
更多查看译文
关键词
glycated hemoglobin HbA1c, prediction, machine learning, deep learning, neural network, multilayer perceptron, electronic health records, time series data, longitudinal data, diabetes
AI 理解论文
溯源树
样例
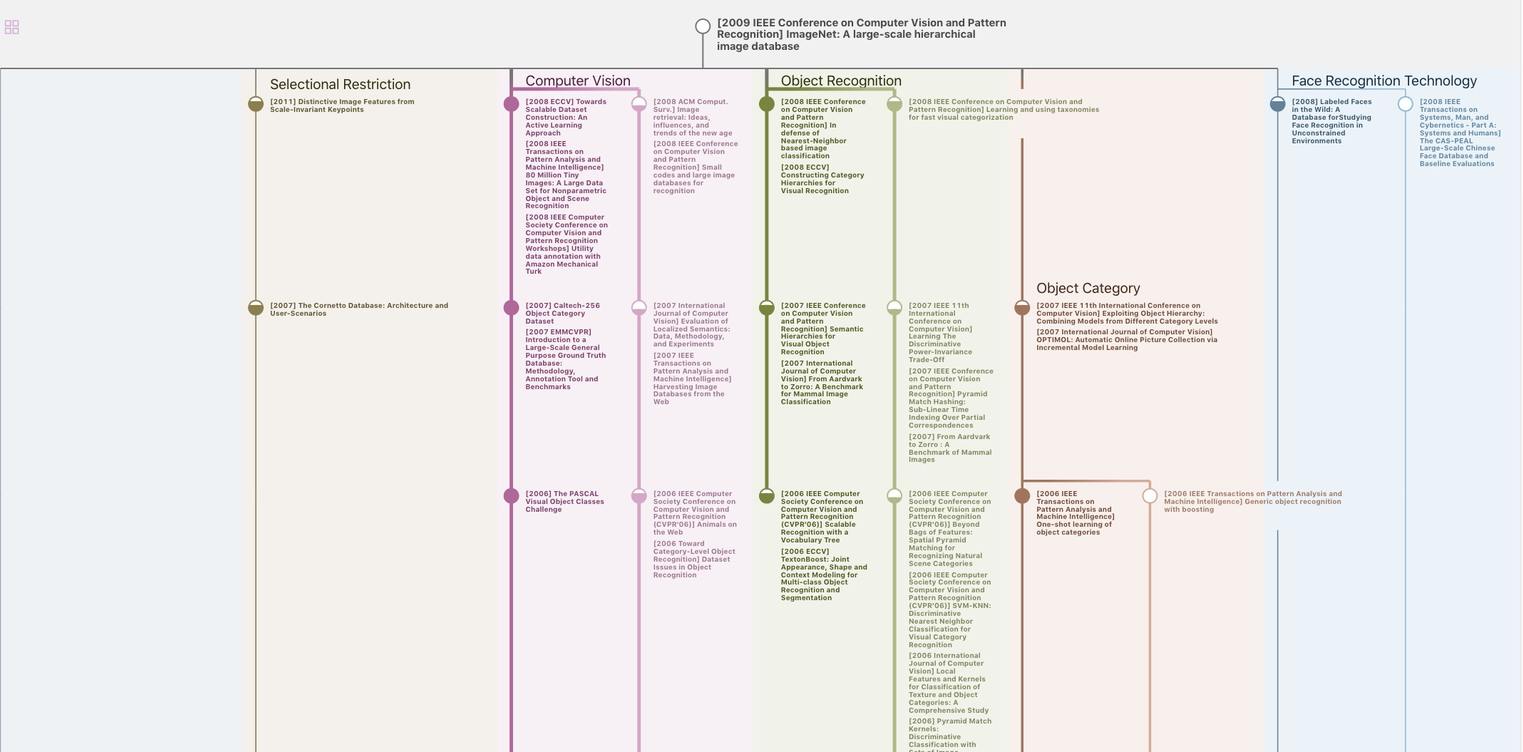
生成溯源树,研究论文发展脉络
Chat Paper
正在生成论文摘要