Data-driven Weight Initialization with Sylvester Solvers
arxiv(2021)
摘要
In this work, we propose a data-driven scheme to initialize the parameters of a deep neural network. This is in contrast to traditional approaches which randomly initialize parameters by sampling from transformed standard distributions. Such methods do not use the training data to produce a more informed initialization. Our method uses a sequential layer-wise approach where each layer is initialized using its input activations. The initialization is cast as an optimization problem where we minimize a combination of encoding and decoding losses of the input activations, which is further constrained by a user-defined latent code. The optimization problem is then restructured into the well-known Sylvester equation, which has fast and efficient gradient-free solutions. Our data-driven method achieves a boost in performance compared to random initialization methods, both before start of training and after training is over. We show that our proposed method is especially effective in few-shot and fine-tuning settings. We conclude this paper with analyses on time complexity and the effect of different latent codes on the recognition performance.
更多查看译文
关键词
sylvester solvers,weight initialization,data-driven
AI 理解论文
溯源树
样例
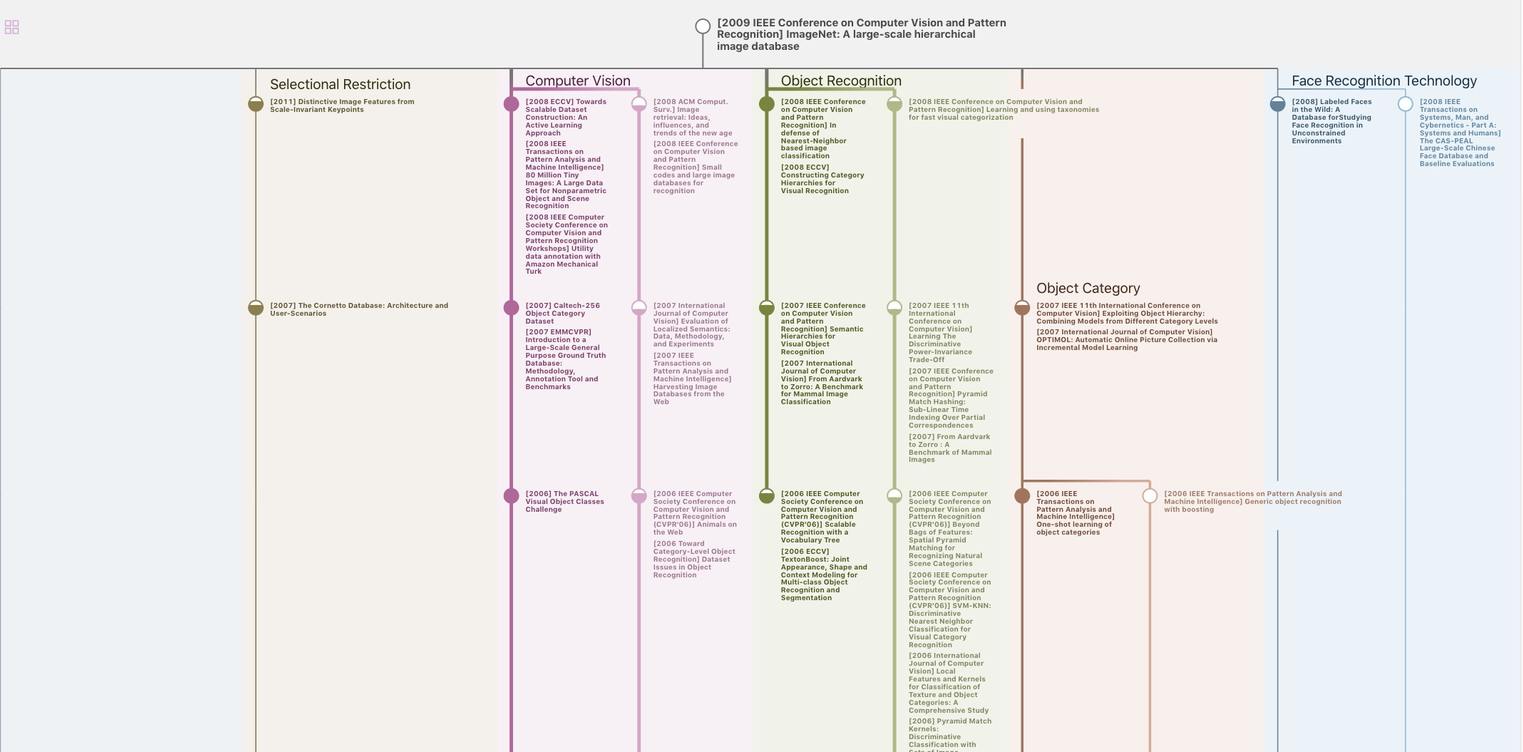
生成溯源树,研究论文发展脉络
Chat Paper
正在生成论文摘要