Learning Transaction Cohesiveness for Online Payment Fraud Detection.
CONF-CDS(2021)
摘要
In the online payment fraud detection scenario, transactions are characterized by attributes. A fraud detection system makes use of the attributes to build a binary classifier that tells fraudulent transactions from legitimate ones. The key factor that affects the quality of a fraud detection system is how to extract useful features from transaction attributes. This paper proposes a novel automatic feature learning approach for online payment fraud detection. To begin with, it represents transaction attributes as vectors in a latent vector space. With these vectors, transaction cohesiveness is defined. By maximizing the probability that legitimate transactions have larger cohesiveness than fraudulent ones, the vector representations of attributes can be optimized. Experiments demonstrate that the selected classifiers trained with the cohesive features show superior performance than those with the original attributes.
更多查看译文
AI 理解论文
溯源树
样例
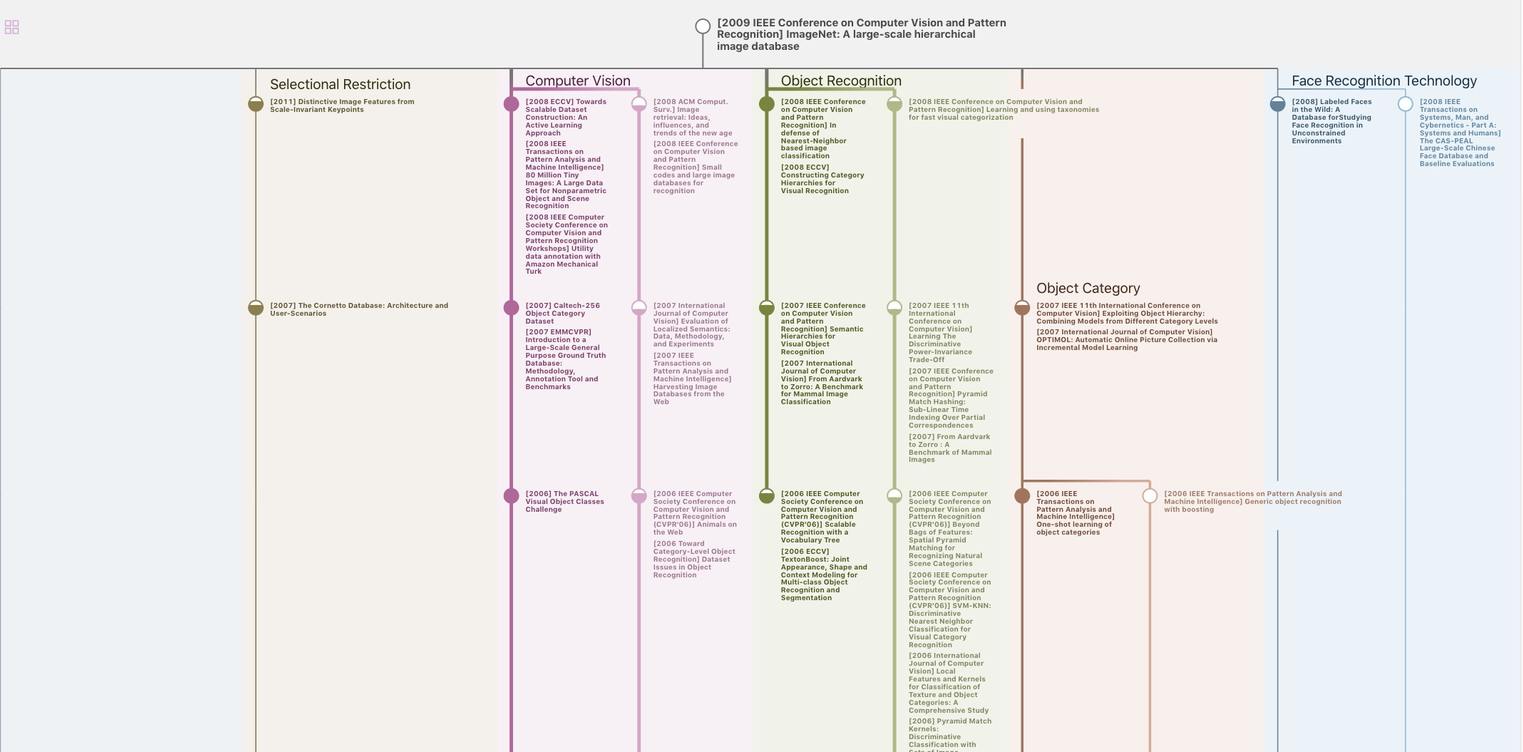
生成溯源树,研究论文发展脉络
Chat Paper
正在生成论文摘要