Image-Guided Human Reconstruction via Multi-Scale Graph Transformation Networks
IEEE TRANSACTIONS ON IMAGE PROCESSING(2021)
摘要
3D human reconstruction from a single image is a challenging problem. Existing methods have difficulties to infer 3D clothed human models with consistent topologies for various poses. In this paper, we propose an efficient and effective method using a hierarchical graph transformation network. To deal with large deformations and avoid distorted geometries, rather than using Euclidean coordinates directly, 3D human shapes are represented by a vertex-based deformation representation that effectively encodes the deformation and copes well with large deformations. To infer a 3D human mesh consistent with the input real image, we also use a perspective projection layer to incorporate perceptual image features into the deformation representation. Our model is easy to train and fast to converge with short test time. Besides, we present the
$D^{2}Human$
(Dynamic Detailed Human) dataset, including variously posed 3D human meshes with consistent topologies and rich geometry details, together with the captured color images and SMPL models, which is useful for training and evaluation of deep frameworks, particularly for graph neural networks. Experimental results demonstrate that our method achieves more plausible and complete 3D human reconstruction from a single image, compared with several state-of-the-art methods. The code and dataset are available for research purposes at
http://cic.tju.edu.cn/faculty/likun/projects/MGTnet
.
更多查看译文
关键词
3D human reconstruction, single image, deformation representation, graph neural networks, dataset
AI 理解论文
溯源树
样例
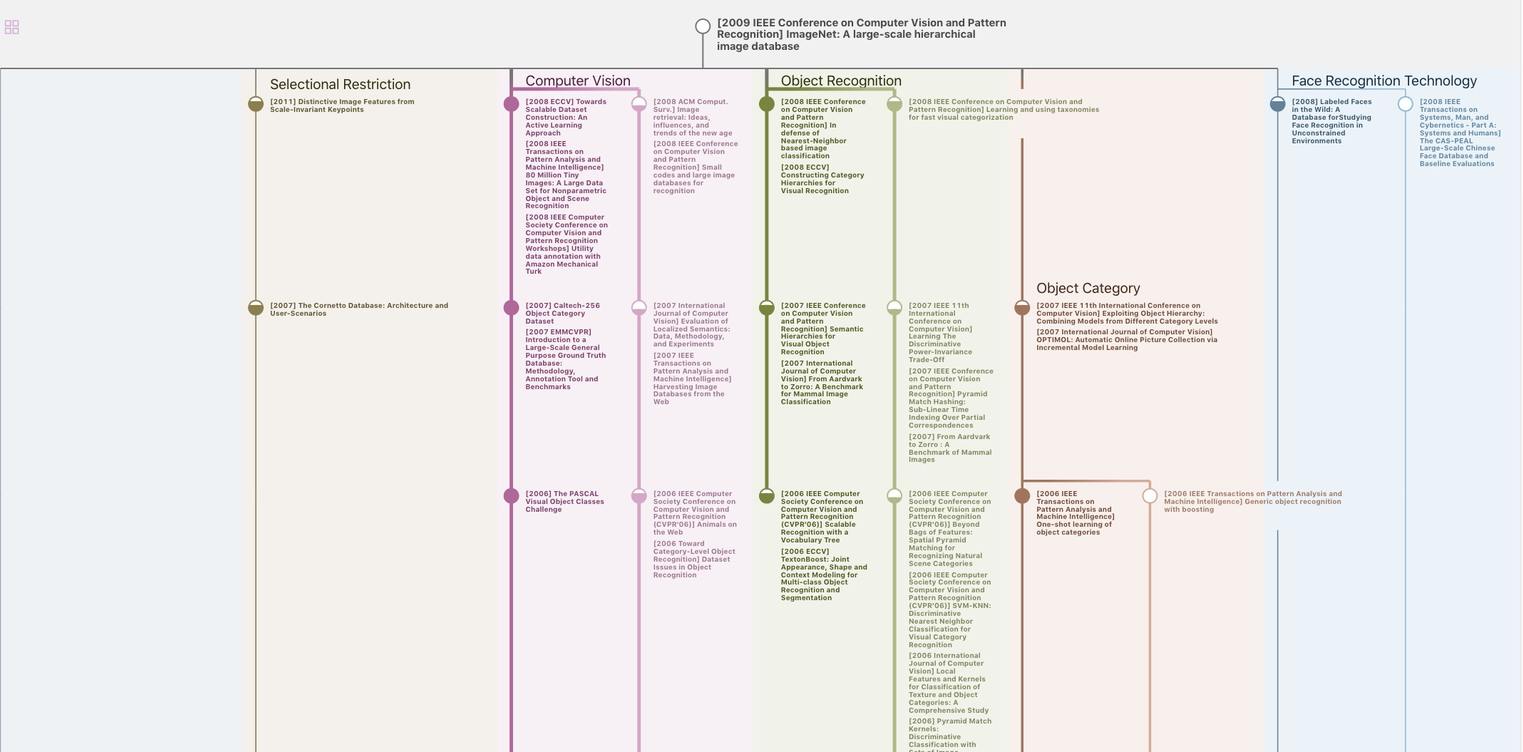
生成溯源树,研究论文发展脉络
Chat Paper
正在生成论文摘要