User-Level Label Leakage from Gradients in Federated Learning.
Proc. Priv. Enhancing Technol.(2022)
摘要
Federated learning enables multiple users to build a joint model by sharing their model updates (gradients), while their raw data remains local on their devices. In contrast to the common belief that this provides privacy benefits, we here add to the very recent results on privacy risks when sharing gradients. Specifically, we propose Label Leakage from Gradients (LLG), a novel attack to extract the labels of the users' training data from their shared gradients. The attack exploits the direction and magnitude of gradients to determine the presence or absence of any label. LLG is simple yet effective, capable of leaking potential sensitive information represented by labels, and scales well to arbitrary batch sizes and multiple classes. We empirically and mathematically demonstrate the validity of our attack under different settings. Moreover, empirical results show that LLG successfully extracts labels with high accuracy at the early stages of model training. We also discuss different defense mechanisms against such leakage. Our findings suggest that gradient compression is a practical technique to prevent our attack.
更多查看译文
关键词
label,learning,gradients,user-level
AI 理解论文
溯源树
样例
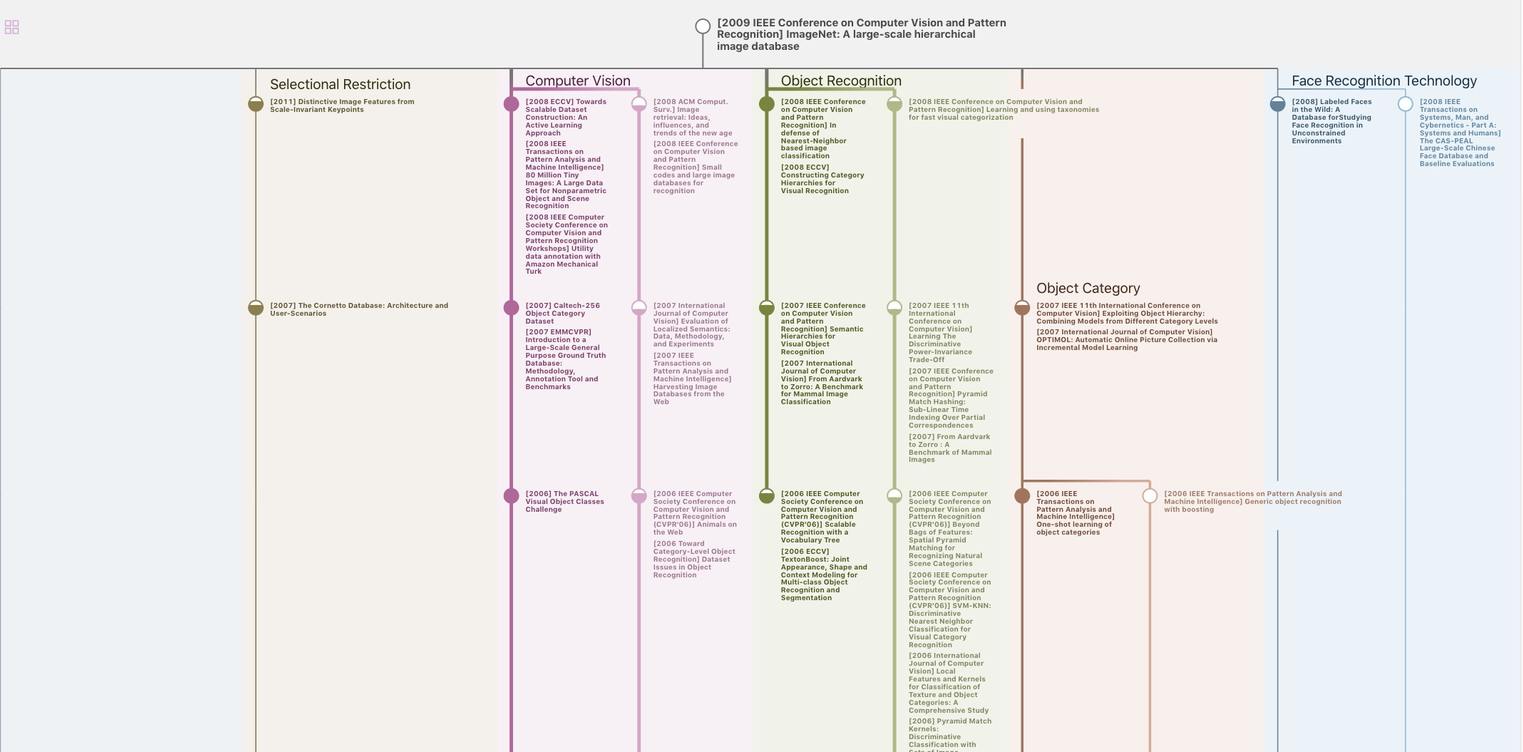
生成溯源树,研究论文发展脉络
Chat Paper
正在生成论文摘要