Boosting Variational Inference With Locally Adaptive Step-Sizes.
IJCAI(2021)
摘要
Variational Inference makes a trade-off between the capacity of the variational family and the tractability of finding an approximate posterior distribution. Instead, Boosting Variational Inference allows practitioners to obtain increasingly good posterior approximations by spending more compute. The main obstacle to widespread adoption of Boosting Variational Inference is the amount of resources necessary to improve over a strong Variational Inference baseline. In our work, we trace this limitation back to the global curvature of the KL-divergence. We characterize how the global curvature impacts time and memory consumption, address the problem with the notion of local curvature, and provide a novel approximate backtracking algorithm for estimating local curvature. We give new theoretical convergence rates for our algorithms and provide experimental validation on synthetic and real-world datasets.
更多查看译文
关键词
variational inference,step-sizes
AI 理解论文
溯源树
样例
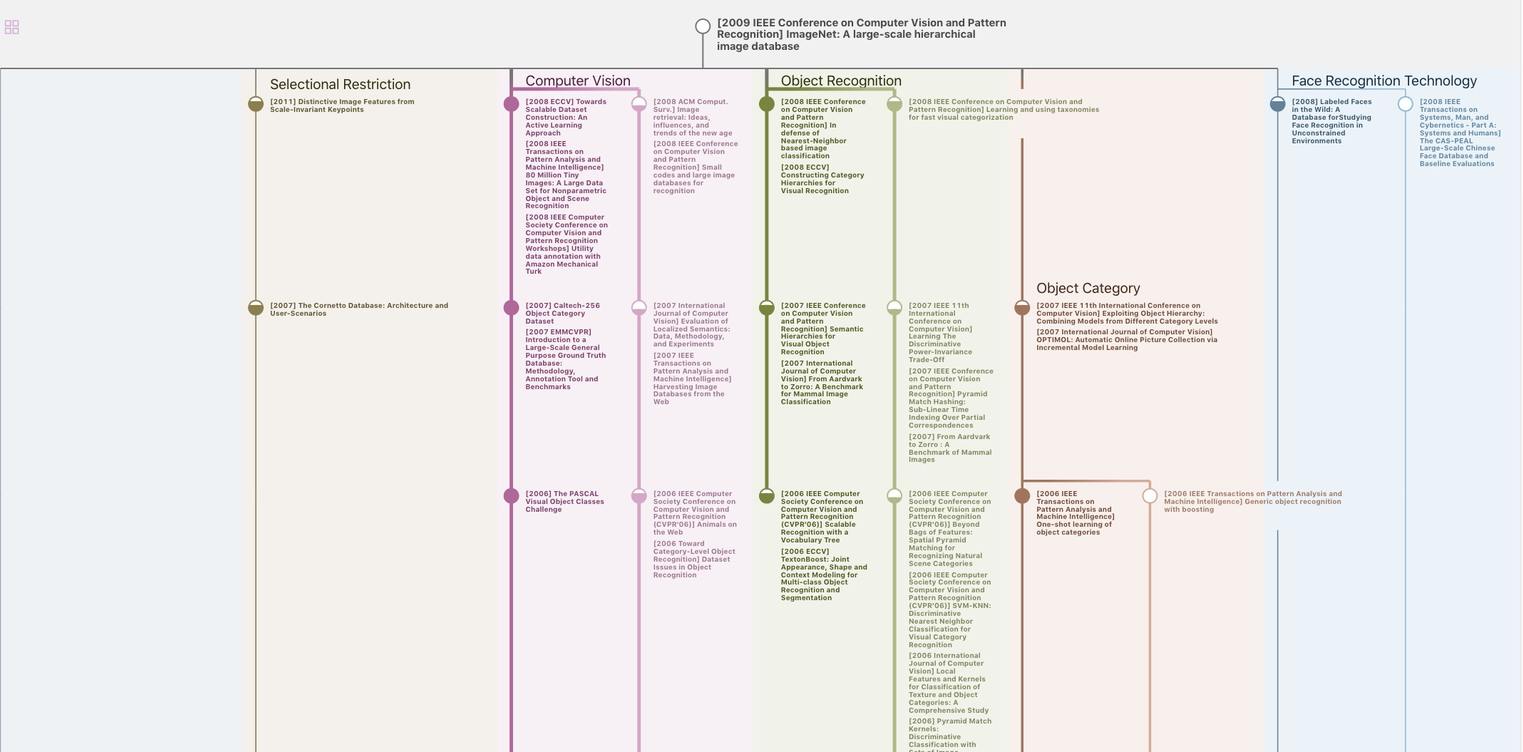
生成溯源树,研究论文发展脉络
Chat Paper
正在生成论文摘要