Prognosis prediction of extremity and trunk wall soft-tissue sarcomas treated with surgical resection with radiomic analysis based on random survival forest
UPDATES IN SURGERY(2021)
摘要
Many researches have applied machine learning methods to find associations between radiomic features and clinical outcomes. Random survival forests (RSF), as an accurate classifier, sort all candidate variables as the rank of importance values. There was no study concerning on finding radiomic predictors in patients with extremity and trunk wall soft-tissue sarcomas using RSF. This study aimed to determine associations between radiomic features and overall survival (OS) by RSF analysis. To identify radiomic features with important values by RSF analysis, construct predictive models for OS incorporating clinical characteristics, and evaluate models’ performance with different method. We collected clinical characteristics and radiomic features extracted from plain and contrast-enhanced computed tomography (CT) from 353 patients with extremity and trunk wall soft-tissue sarcomas treated with surgical resection. All radiomic features were analyzed by Cox proportional hazard (CPH) and followed RSF analysis. The association between radiomics-predicted risks and OS was assessed by Kaplan–Meier analysis. All clinical features were screened by CPH analysis. Prognostic clinical and radiomic parameters were fitted into RSF and CPH integrative models for OS in the training cohort, respectively. The concordance indexes (C-index) and Brier scores of both two models were evaluated in both training and testing cohorts. The model with better predictive performance was interpreted with nomogram and calibration plots. Among all 86 radiomic features, there were three variables selected with high importance values. The RSF on these three features distinguished patients with high predicted risks from patients with low predicted risks for OS in the training set ( P < 0.001) using Kaplan–Meier analysis. Age, lymph node involvement and grade were incorporated into the combined models for OS ( P < 0.05). The C-indexes in both two integrative models fluctuated above 0.80 whose Brier scores maintained less than 15.0 in the training and testing datasets. The RSF model performed little advantages over the CPH model that the calibration curve of the RSF model showed favorable agreement between predicted and actual survival probabilities for the 3-year and 5-year survival prediction. The multimodality RSF model including clinical and radiomic characteristics conducted high capacity in prediction of OS which might assist individualized therapeutic regimens. Level III, prognostic study.
更多查看译文
关键词
Soft-tissue sarcoma,Random survival forests,Cox proportional hazard,Radiomics,Texture analysis,Nomogram,Prognosis
AI 理解论文
溯源树
样例
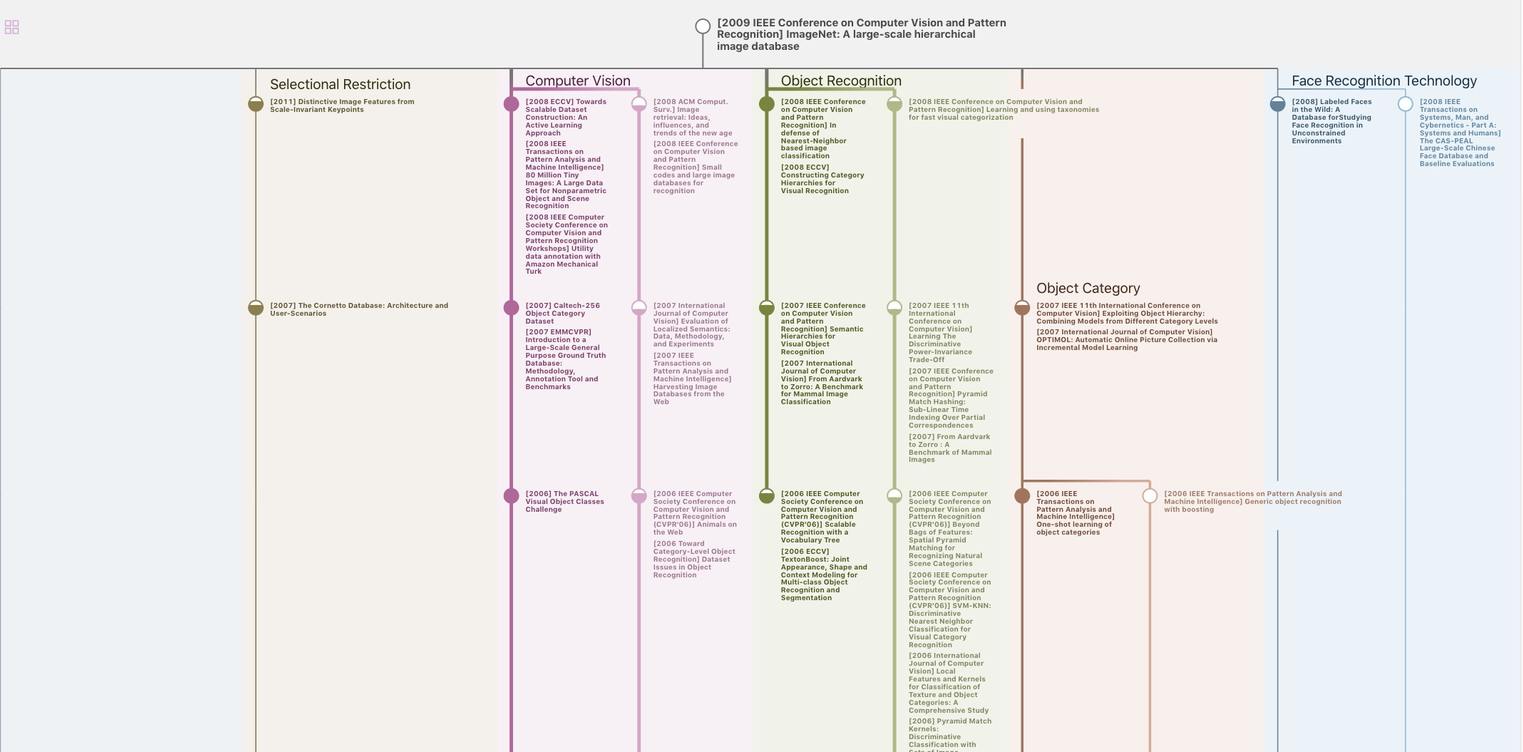
生成溯源树,研究论文发展脉络
Chat Paper
正在生成论文摘要