Inplace knowledge distillation with teacher assistant for improved training of flexible deep neural networks
29TH EUROPEAN SIGNAL PROCESSING CONFERENCE (EUSIPCO 2021)(2021)
摘要
Deep neural networks (DNNs) have achieved great success in various machine learning tasks. However, most existing powerful DNN models are computationally expensive and memory demanding, hindering their deployment in devices with low memory and computational resources or in applications with strict latency requirements. Thus, several resource-adaptable or flexible approaches were recently proposed that train at the same time a big model and several resource-specific sub-models. Inplace knowledge distillation (IPKD) became a popular method to train those models and consists in distilling the knowledge from a larger model (teacher) to all other sub-models (students). In this work a novel generic training method called IPKD with teacher assistant (IPKD-TA) is introduced, where sub-models themselves become teacher assistants teaching smaller sub-models. We evaluated the proposed IPKD-TA training method using two state-of-the-art flexible models (MSDNet and Slimmable MobileNet-V1) with two popular image classification benchmarks (CIFAR-10 and CIFAR-100). Our results demonstrate that the IPKD-TA is on par with the existing state of the art while improving it in most cases.
更多查看译文
关键词
Deep Neural Networks, Flexible Models, Inplace Knowledge Distillation with Teacher Assistant
AI 理解论文
溯源树
样例
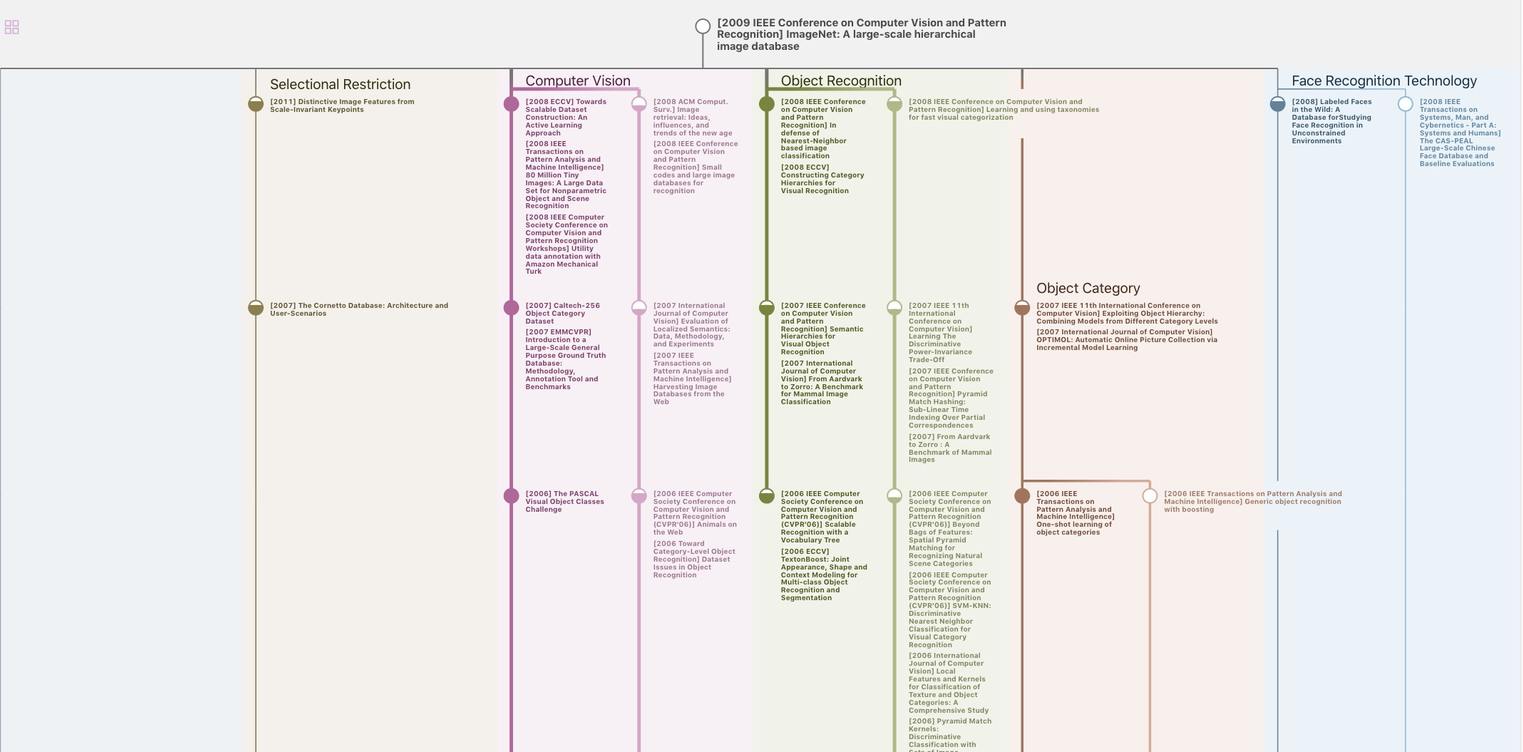
生成溯源树,研究论文发展脉络
Chat Paper
正在生成论文摘要