An Interpretable Graph-Based Mapping Of Trustworthy Machine Learning Research
COMPLEX NETWORKS XII(2021)
摘要
There is an increasing interest in ensuring machine learning (ML) frameworks behave in a socially responsible manner and are deemed trustworthy. Although considerable progress has been made in the field of Trustworthy ML (TwML) in the recent past, much of the current characterization of this progress is qualitative. Consequently, decisions about how to address issues of trustworthiness and future research goals are often left to the interested researcher. In this paper, we present the first quantitative approach to characterize the comprehension of TwML research. We build a co-occurrence network of words using a web-scraped corpus of more than 7,000 peer-reviewed recent ML papers-consisting of papers both related and unrelated to TwML. We use community detection to obtain semantic clusters of words in this network that can infer relative positions of TwML topics. We propose an innovative fingerprinting algorithm to obtain probabilistic similarity scores for individual words, then combine them to give a paper-level relevance score. The outcomes of our analysis inform a number of interesting insights on advancing the field of TwML research.
更多查看译文
关键词
Trustworthy machine learning, Natural language processing, Research space, Co-occurrence network, Community detection
AI 理解论文
溯源树
样例
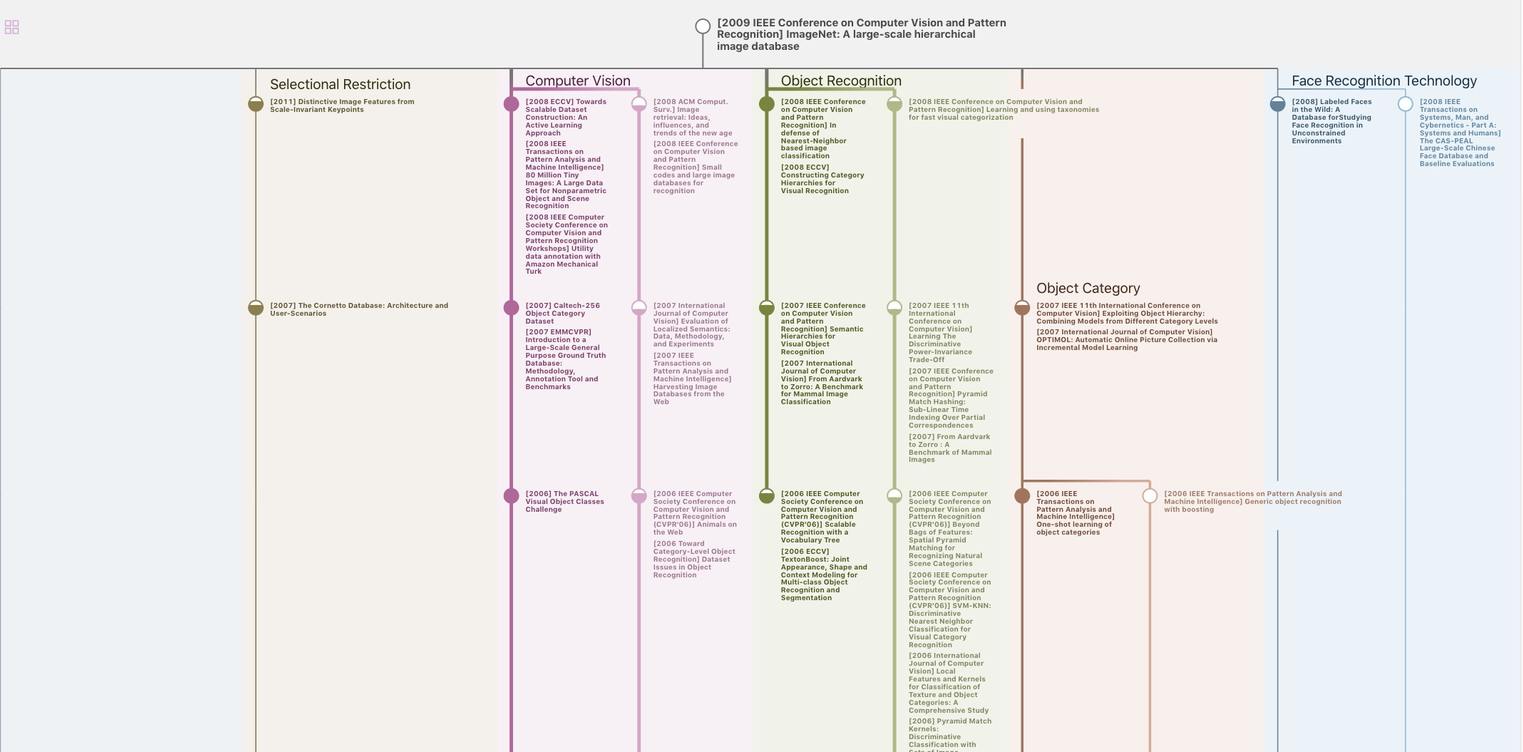
生成溯源树,研究论文发展脉络
Chat Paper
正在生成论文摘要