Knowledge Transfer with Weighted Adversarial Network for Cold-Start Store Site Recommendation
ACM Transactions on Knowledge Discovery from Data(2021)
摘要
AbstractStore site recommendation aims to predict the value of the store at candidate locations and then recommend the optimal location to the company for placing a new brick-and-mortar store. Most existing studies focus on learning machine learning or deep learning models based on large-scale training data of existing chain stores in the same city. However, the expansion of chain enterprises in new cities suffers from data scarcity issues, and these models do not work in the new city where no chain store has been placed (i.e., cold-start problem). In this article, we propose a unified approach for cold-start store site recommendation, Weighted Adversarial Network with Transferability weighting scheme (WANT), to transfer knowledge learned from a data-rich source city to a target city with no labeled data. In particular, to promote positive transfer, we develop a discriminator to diminish distribution discrepancy between source city and target city with different data distributions, which plays the minimax game with the feature extractor to learn transferable representations across cities by adversarial learning. In addition, to further reduce the risk of negative transfer, we design a transferability weighting scheme to quantify the transferability of examples in source city and reweight the contribution of relevant source examples to transfer useful knowledge. We validate WANT using a real-world dataset, and experimental results demonstrate the effectiveness of our proposed model over several state-of-the-art baseline models.
更多查看译文
关键词
Urban computing, cold-start problem, store site recommendation, transfer learning, neural networks
AI 理解论文
溯源树
样例
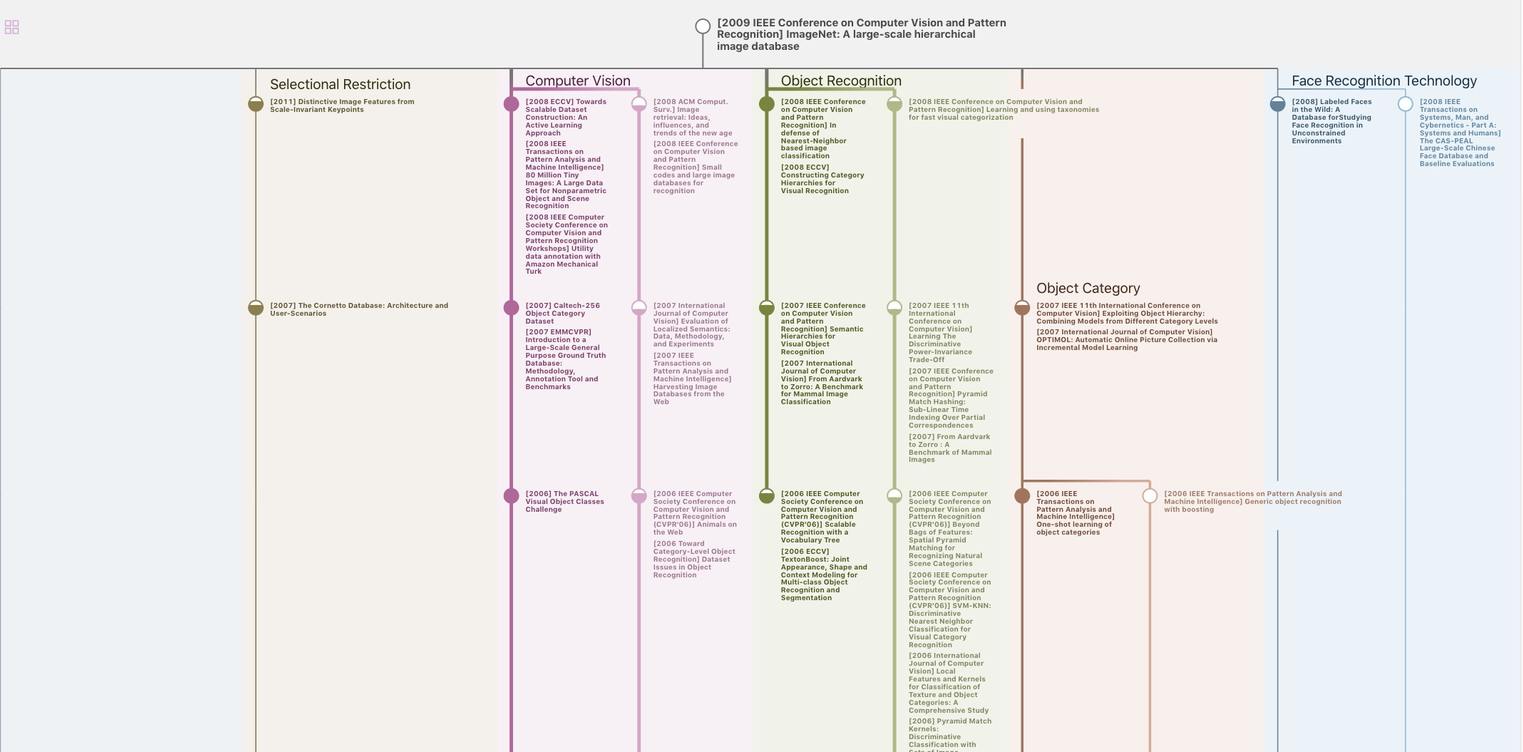
生成溯源树,研究论文发展脉络
Chat Paper
正在生成论文摘要