Sufficient Reasons for Classifier Decisions in the Presence of Domain Constraints.
arxiv(2022)
摘要
Recent work has unveiled a theory for reasoning about the decisions made by binary classifiers: a classifier describes a Boolean function, and the reasons behind an instance being classified as positive are the prime-implicants of the function that are satisfied by the instance. One drawback of these works is that they do not explicitly treat scenarios where the underlying data is known to be constrained, e.g., certain combinations of features may not exist, may not be observable, or may be required to be disregarded. We propose a more general theory, also based on prime-implicants, tailored to taking constraints into account. The main idea is to view classifiers as describing partial Boolean functions that are undefined on instances that do not satisfy the constraints. We prove that this simple idea results in more parsimonious reasons. That is, not taking constraints into account (e.g., ignoring, or taking them as negative instances) results in reasons that are subsumed by reasons that do take constraints into account. We illustrate this improved succinctness on synthetic classifiers and classifiers learnt from real data.
更多查看译文
关键词
Knowledge Representation And Reasoning (KRR)
AI 理解论文
溯源树
样例
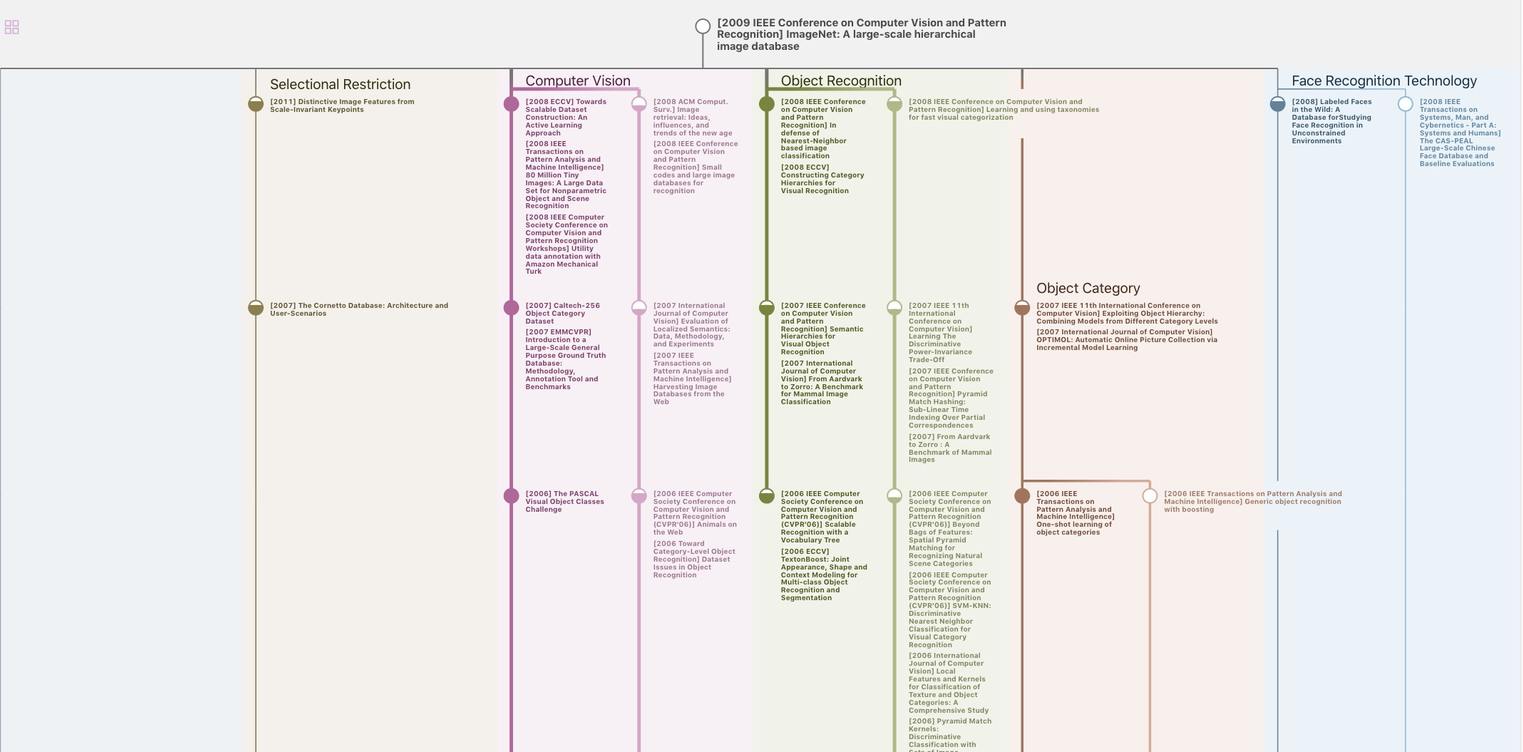
生成溯源树,研究论文发展脉络
Chat Paper
正在生成论文摘要