Efficient Algorithms for Estimating the Parameters of Mixed Linear Regression Models
arxiv(2021)
摘要
Mixed linear regression (MLR) model is among the most exemplary statistical tools for modeling non-linear distributions using a mixture of linear models. When the additive noise in MLR model is Gaussian, Expectation-Maximization (EM) algorithm is a widely-used algorithm for maximum likelihood estimation of MLR parameters. However, when noise is non-Gaussian, the steps of EM algorithm may not have closed-form update rules, which makes EM algorithm impractical. In this work, we study the maximum likelihood estimation of the parameters of MLR model when the additive noise has non-Gaussian distribution. In particular, we consider the case that noise has Laplacian distribution and we first show that unlike the the Gaussian case, the resulting sub-problems of EM algorithm in this case does not have closed-form update rule, thus preventing us from using EM in this case. To overcome this issue, we propose a new algorithm based on combining the alternating direction method of multipliers (ADMM) with EM algorithm idea. Our numerical experiments show that our method outperforms the EM algorithm in statistical accuracy and computational time in non-Gaussian noise case.
更多查看译文
关键词
mixed linear regression models,efficient algorithms,parameters
AI 理解论文
溯源树
样例
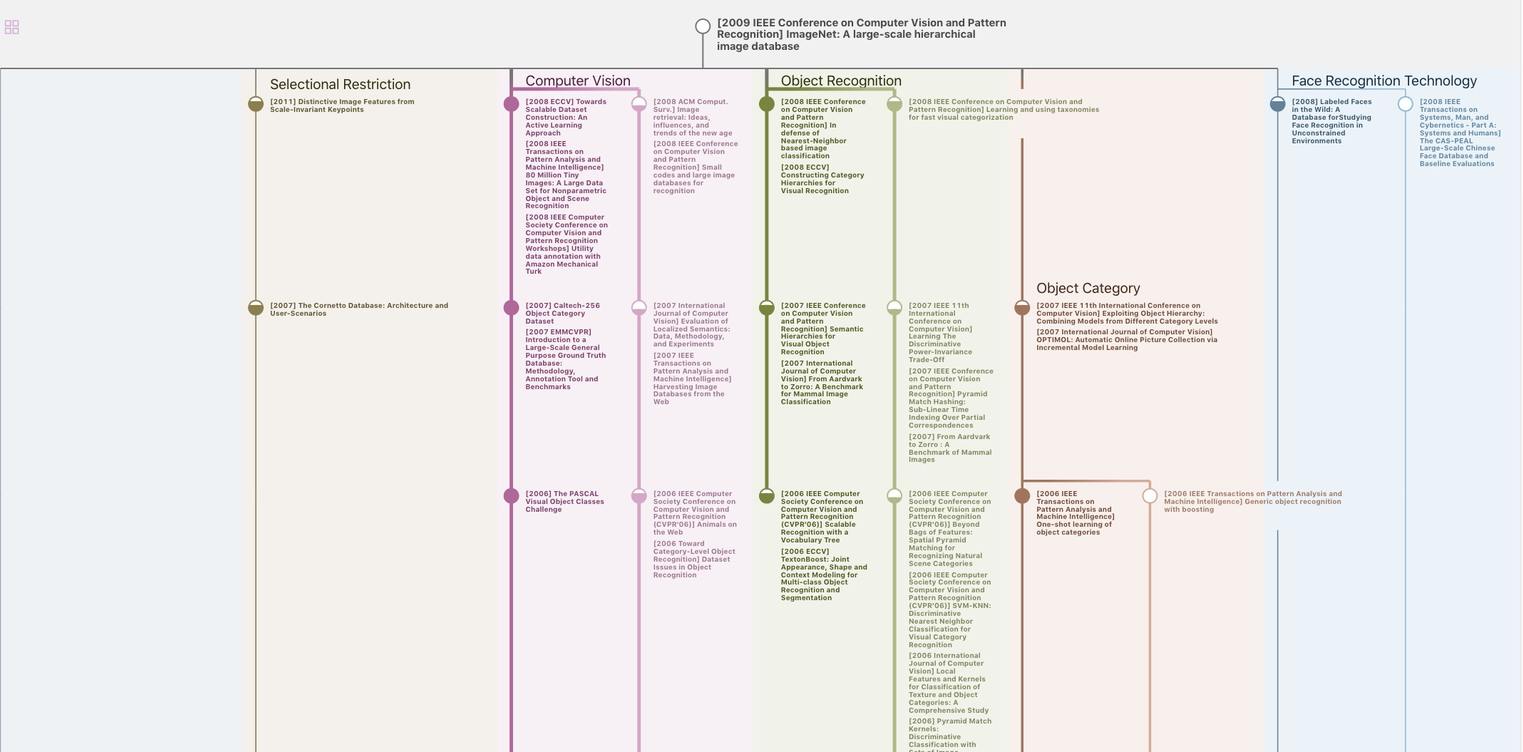
生成溯源树,研究论文发展脉络
Chat Paper
正在生成论文摘要