In Vitro Measurements of Shear-Mediated Platelet Adhesion Kinematics as Analyzed through Machine Learning
ANNALS OF BIOMEDICAL ENGINEERING(2021)
摘要
Platelet adhesion to blood vessel walls in shear flow is essential to initiating the blood coagulation cascade and prompting clot formation in vascular disease processes and prosthetic cardiovascular devices. Validation of predictive adhesion kinematics models at the single platelet level is difficult due to gaps in high resolution, dynamic morphological data or a mismatch between simulation and experimental parameters. Gel-filtered platelets were perfused at 30 dyne/cm 2 in von Willebrand Factor (vWF)-coated microchannels, with flipping platelets imaged at high spatial and temporal resolution. A semi-unsupervised learning system (SULS), consisting of a series of convolutional neural networks, was used to segment platelet geometry, which was compared with expert-analyzed images. Resulting time-dependent rotational angles were smoothed with wavelet-denoising and shifting techniques to characterize the rotational period and quantify flipping kinematics. We observed that flipping platelets do not follow the previously-modeled modified Jefferey orbit, but are characterized by a longer lift-off and shorter reattachment period. At the juncture of the two periods, rotational velocity approached 257.48 ± 13.31 rad/s. Our SULS approach accurately segmented large numbers of moving platelet images to identify distinct adhesive kinematic characteristics which may further validate the physical accuracy of individual platelet motion in multiscale models of shear-mediated thrombosis.
更多查看译文
关键词
Platelet adhesion, Machine learning, Neural networks, Jeffery orbit, Thrombosis
AI 理解论文
溯源树
样例
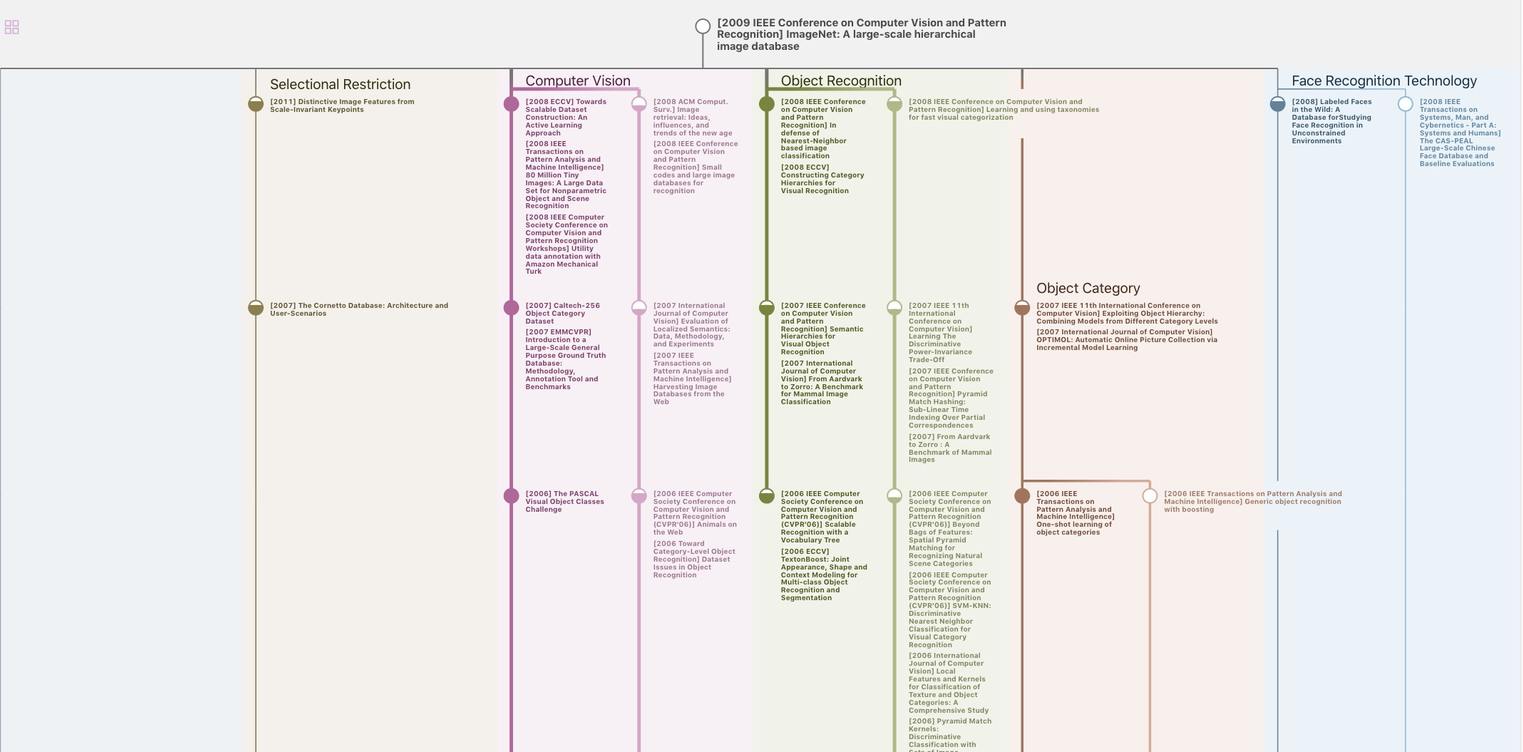
生成溯源树,研究论文发展脉络
Chat Paper
正在生成论文摘要