Sample Selection for Efficient Image Annotation
2021 9th European Workshop on Visual Information Processing (EUVIP)(2021)
摘要
Supervised object detection has been proven to be successful in many benchmark datasets achieving human-level performances. However, acquiring a large amount of labeled image samples for supervised detection training is tedious, time-consuming, and costly. In this paper, we propose an efficient image selection approach that samples the most informative images from the unlabeled dataset and utilizes human-machine collaboration in an iterative train-annotate loop. Image features are extracted by the CNN network followed by the similarity score calculation, Euclidean distance. Unlabeled images are then sampled into different approaches based on the similarity score. The proposed approach is straightforward, simple and sampling takes place prior to the network training. Experiments on datasets show that our method can reduce up to 80% of manual annotation workload, compared to full manual labeling setting, and performs better than random sampling.
更多查看译文
关键词
Object Detection,Image Annotation,Sample Selection,Bounding Box
AI 理解论文
溯源树
样例
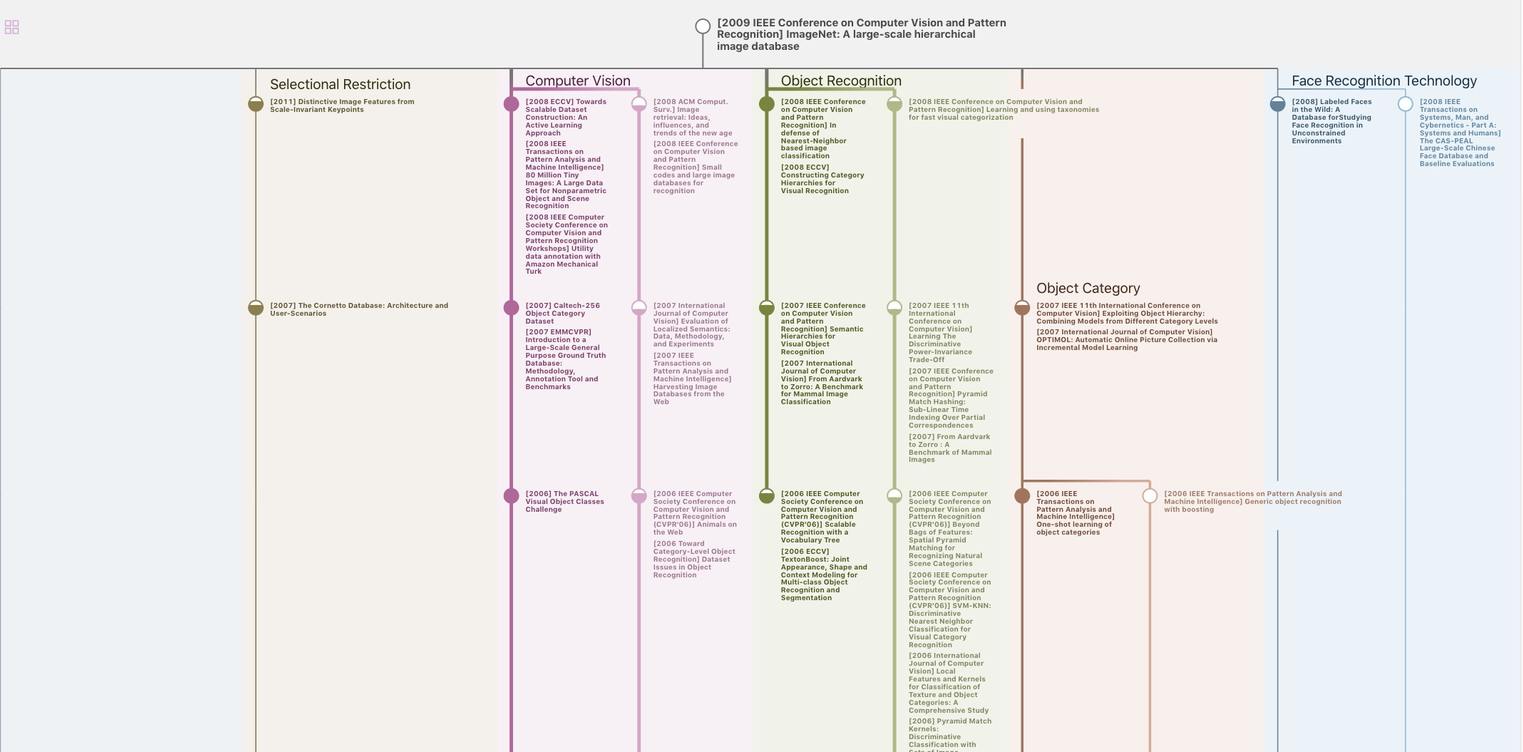
生成溯源树,研究论文发展脉络
Chat Paper
正在生成论文摘要