Bearing Fault Diagnosis Approach Under Data Quality Issues
APPLIED SCIENCES-BASEL(2021)
摘要
In rotary machinery, bearings are susceptible to different types of mechanical faults, including ball, inner race, and outer race faults. In condition-based monitoring (CBM), several techniques have been proposed in fault diagnostics based on the vibration measurements. For this paper, we studied the fractal characteristics of non-stationary vibration signals collected from bearings under different health conditions. Using the detrended fluctuation analysis (DFA), we proposed a novel method to diagnose the bearing faults based on the scaling exponent (alpha(1)) of vibration signal at the short-time scale. In vibration data with high sampling rate, our results showed that the proposed measure, scaling exponent, provides an accurate identification of the health state of the bearing. At the end, we evaluated the performance of the proposed method under different data quality issues, data loss and induced noise.
更多查看译文
关键词
bearing, vibration, diagnostic, non-stationarity, detrended fluctuation analysis, fractal characteristics, scaling exponent, data anomalies, Gaussian noise
AI 理解论文
溯源树
样例
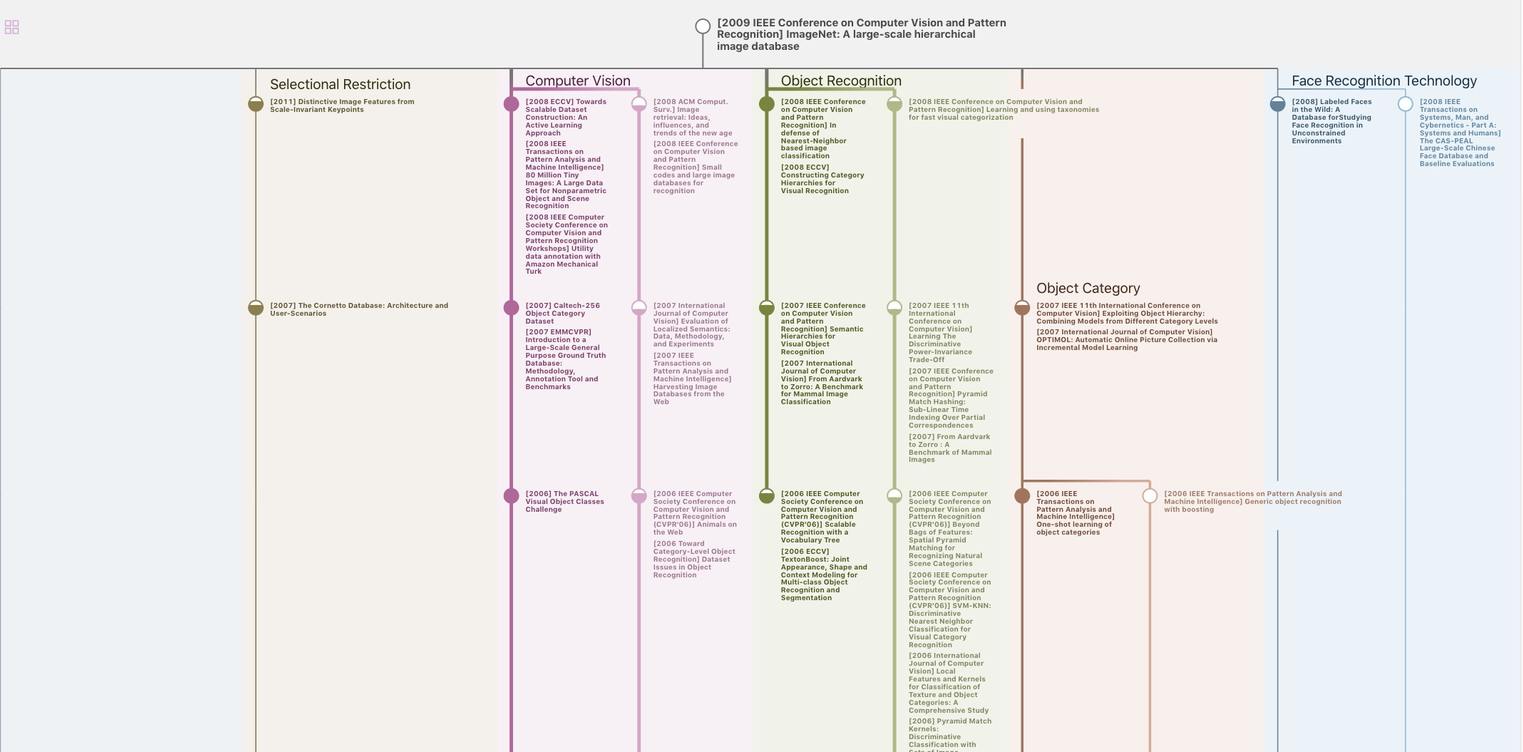
生成溯源树,研究论文发展脉络
Chat Paper
正在生成论文摘要